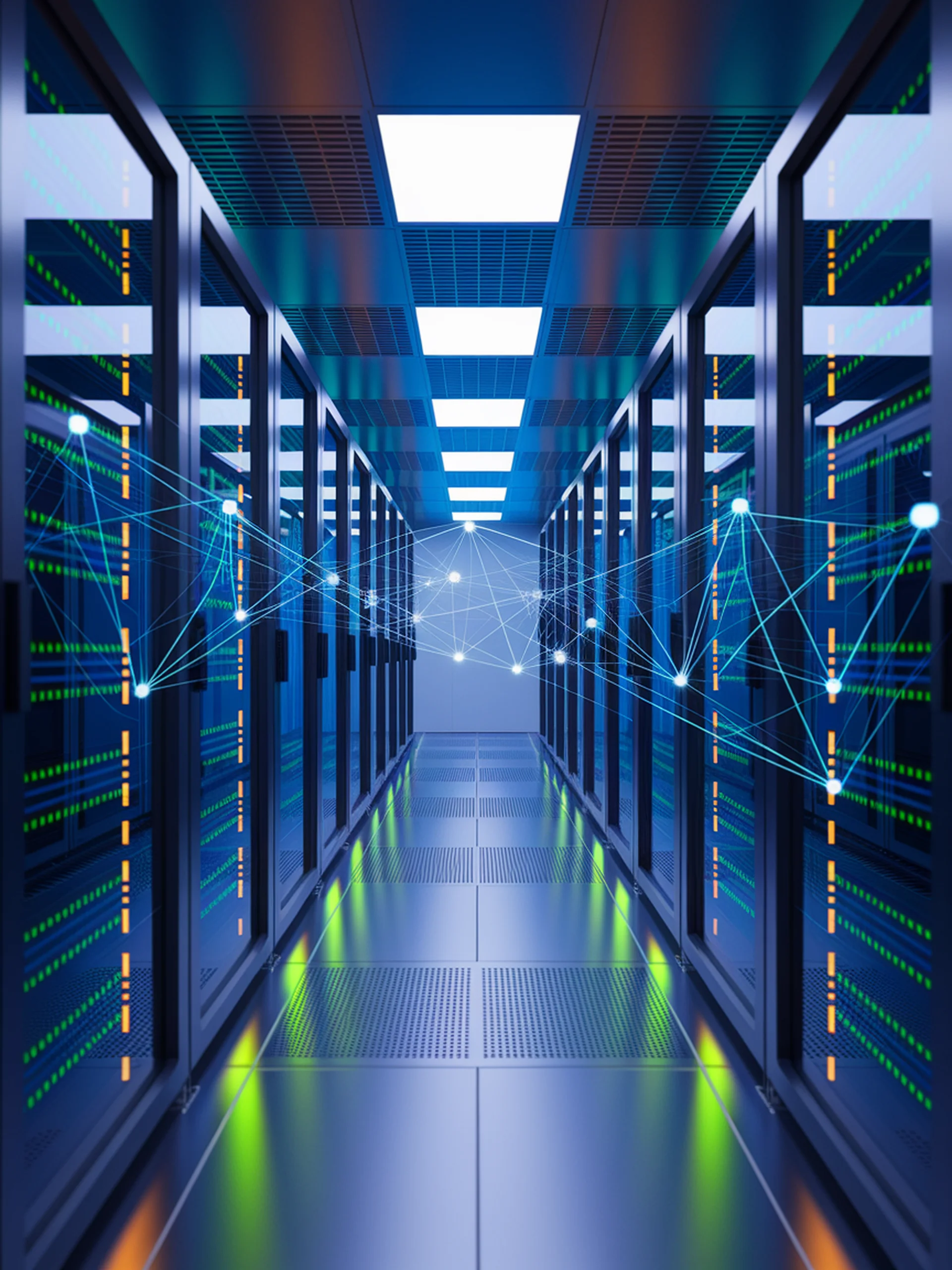
Securing Graph Learning with LLMs
A privacy-preserving approach to federated graph learning using large language models
This research introduces a novel data-centric approach to tackle heterogeneity problems in federated graph learning (FGL) while preserving privacy.
- Leverages large language models to address non-IID data distributions across clients
- Enables collaborative model training without sharing sensitive raw graph data
- Enhances both convergence and performance of federated graphs
- Prioritizes security by only transmitting model parameters between clients
This innovation matters for security teams as it provides a framework for organizations to collaborate on graph learning tasks while maintaining strict data privacy compliance.
Data-centric Federated Graph Learning with Large Language Models