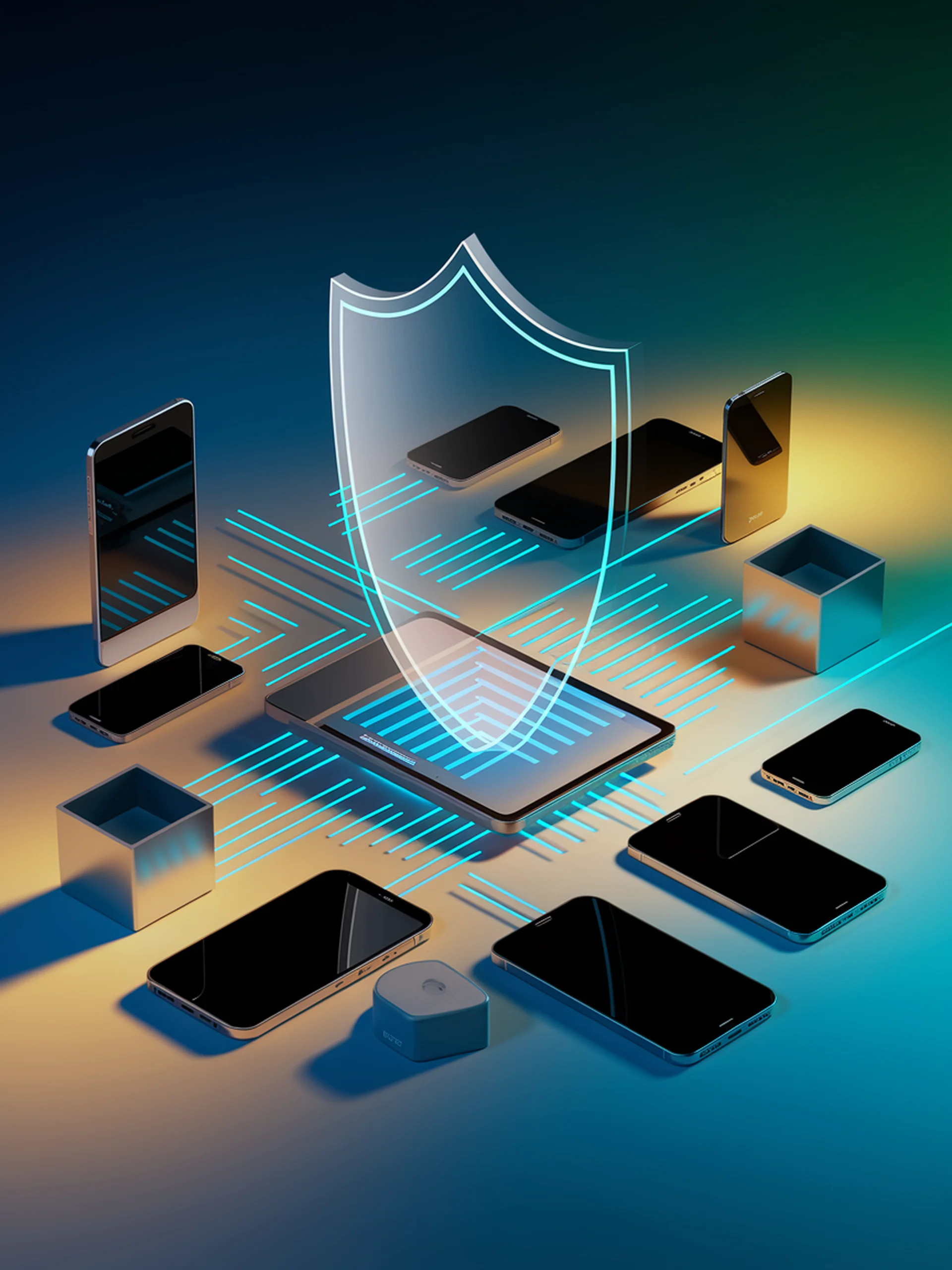
Securing Federated Learning at the Edge
Building Resilient Collaborative AI Systems for Decentralized Environments
This research advances resilient federated learning (ResFL) for CyberEdge networks, combining security mechanisms with adaptive learning strategies to enable robust collaborative AI in distributed environments.
- Tackles non-IID data challenges through hierarchical learning approaches that improve scalability and reduce communication overhead
- Implements fault tolerance techniques to detect unreliable devices and refine model updates in adversarial settings
- Integrates feature-oriented security mechanisms with privacy-preserving techniques to protect against poisoning and inference attacks
- Provides a comprehensive framework for secure multi-party computation in federated learning deployments
This research is critical for security professionals as it addresses the growing need for AI systems that can operate securely across decentralized networks while preserving data privacy and maintaining resilience against sophisticated attacks.
Towards Resilient Federated Learning in CyberEdge Networks: Recent Advances and Future Trends