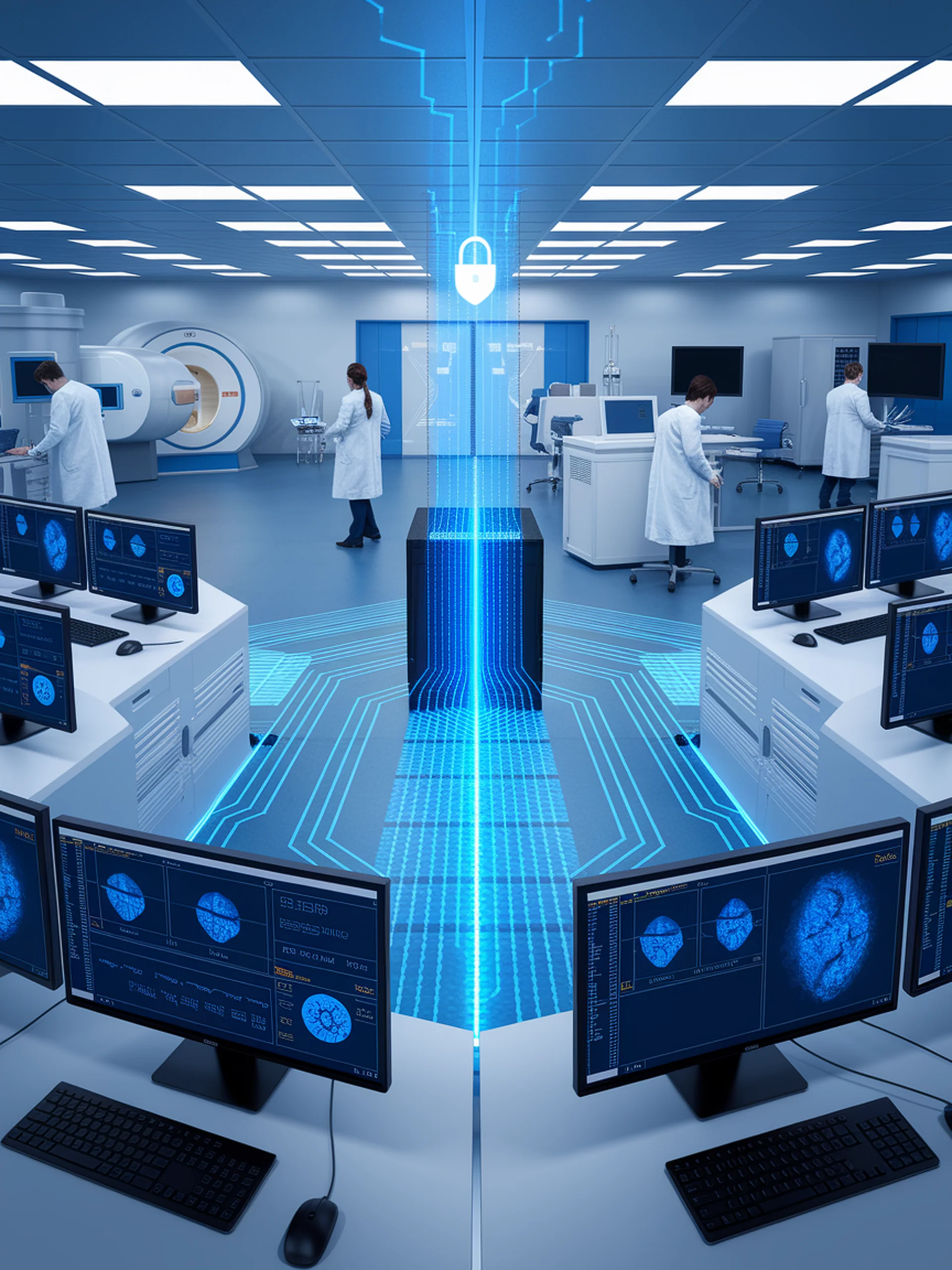
Efficient Federated Learning for Healthcare LLMs
Designing Faster, Privacy-Preserving Models with Layer-Skipping
A novel approach to federated learning that reduces computational costs while preserving privacy for healthcare NLP applications.
- Introduces Layer-Skipping Federated Learning that selectively fine-tunes only certain layers of pre-trained LLMs
- Demonstrates 83% reduction in parameters to train while maintaining comparable performance
- Achieves 30% faster convergence on healthcare NLP tasks like clinical entity recognition
- Successfully handles data heterogeneity across different healthcare institutions
This research enables healthcare organizations to collaborate on developing powerful NLP models without sharing sensitive patient data, addressing critical regulatory compliance needs while advancing clinical NLP capabilities.