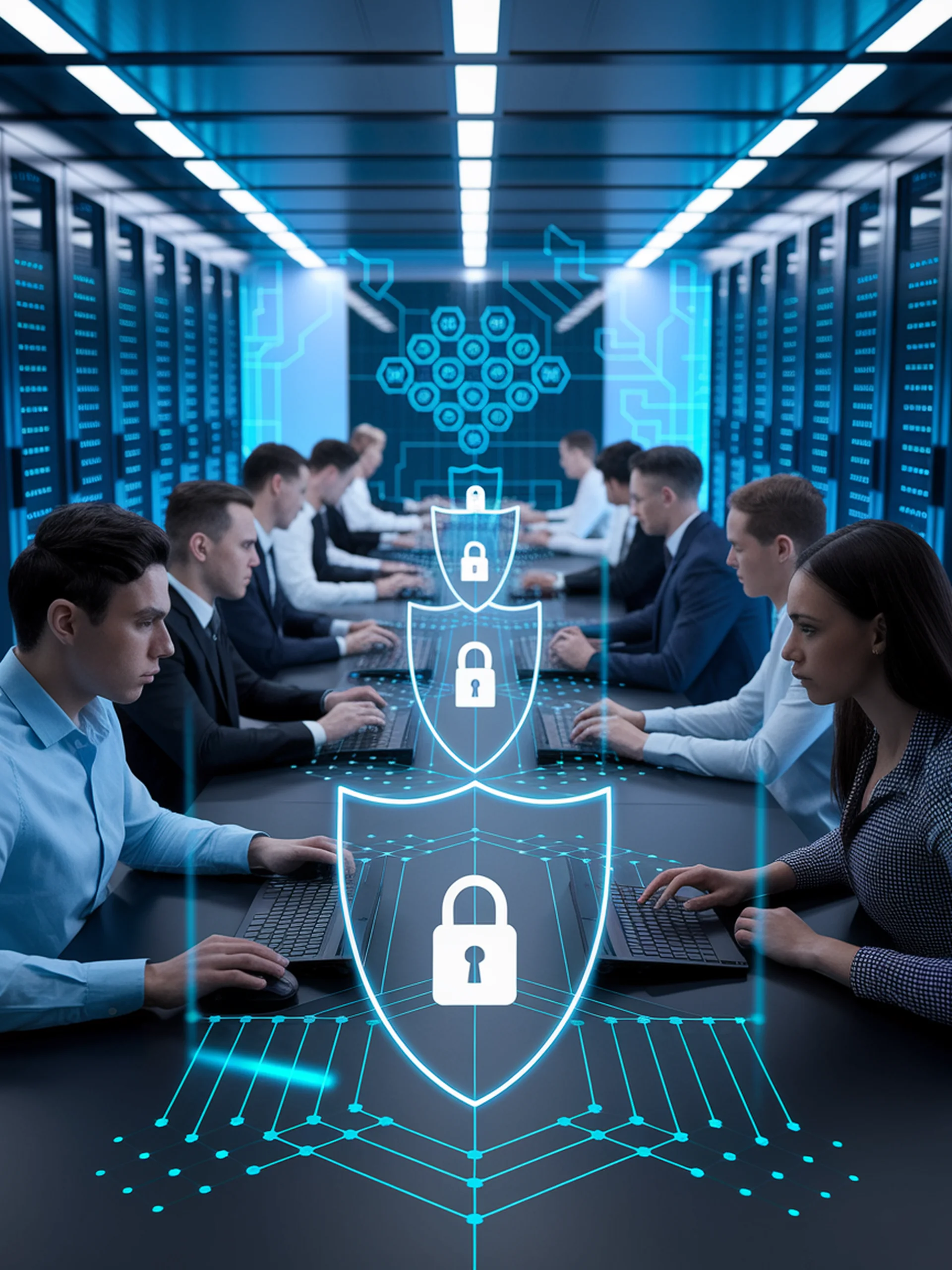
Privacy-Preserving LLM Alignment
Aligning AI with diverse human values without compromising privacy
PluralLLM introduces a federated learning approach that allows LLMs to respect diverse human preferences while protecting user privacy.
- Enables multiple user groups to collaboratively train preference predictors without sharing sensitive data
- Addresses limitations of centralized RLHF methods that are computationally expensive and privacy-invasive
- Preserves both privacy and fairness in model training
- Demonstrates how federated learning can enhance security in LLM alignment
This research matters for security professionals as it establishes a framework for developing AI systems that respect user privacy by design while maintaining cultural diversity in AI responses.
PluralLLM: Pluralistic Alignment in LLMs via Federated Learning