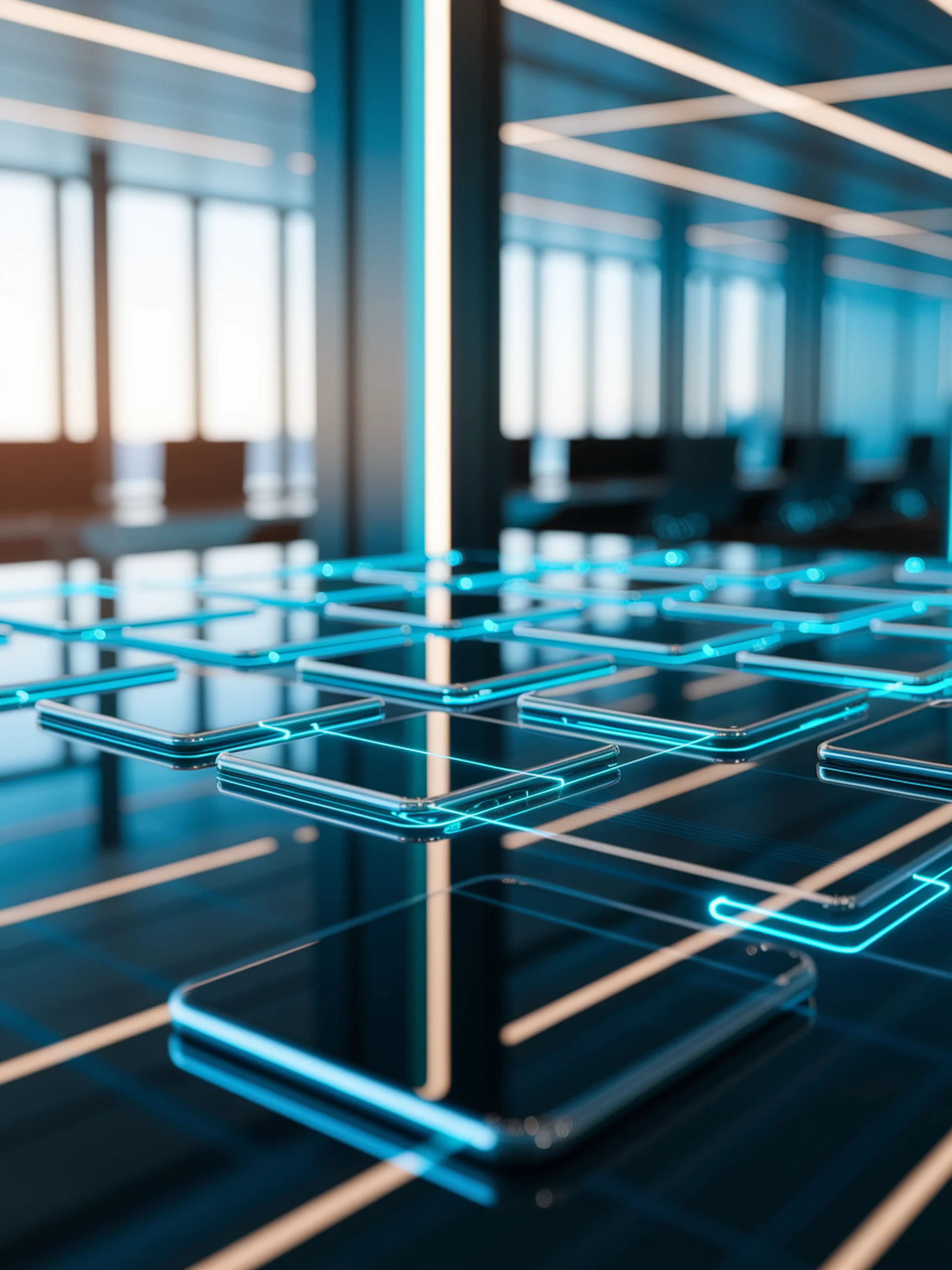
Beyond Centralized Models: Decentralized Federated Learning
Enhancing privacy, robustness, and performance in distributed ML systems
This research explores Decentralized Federated Learning (DFL) as an alternative to centralized approaches, enabling direct device-to-device collaboration without relying on a central server.
Key findings:
- DFL eliminates single points of failure while maintaining strong privacy guarantees
- Performance analysis reveals trade-offs between network topology and model convergence rates
- Decentralized approaches reduce latency and improve system robustness in real-world deployments
- Security benefits include enhanced resilience against certain types of attacks
For security professionals, this research demonstrates how decentralized architectures can improve both data privacy protection and system reliability in distributed machine learning deployments.
Performance Analysis of Decentralized Federated Learning Deployments