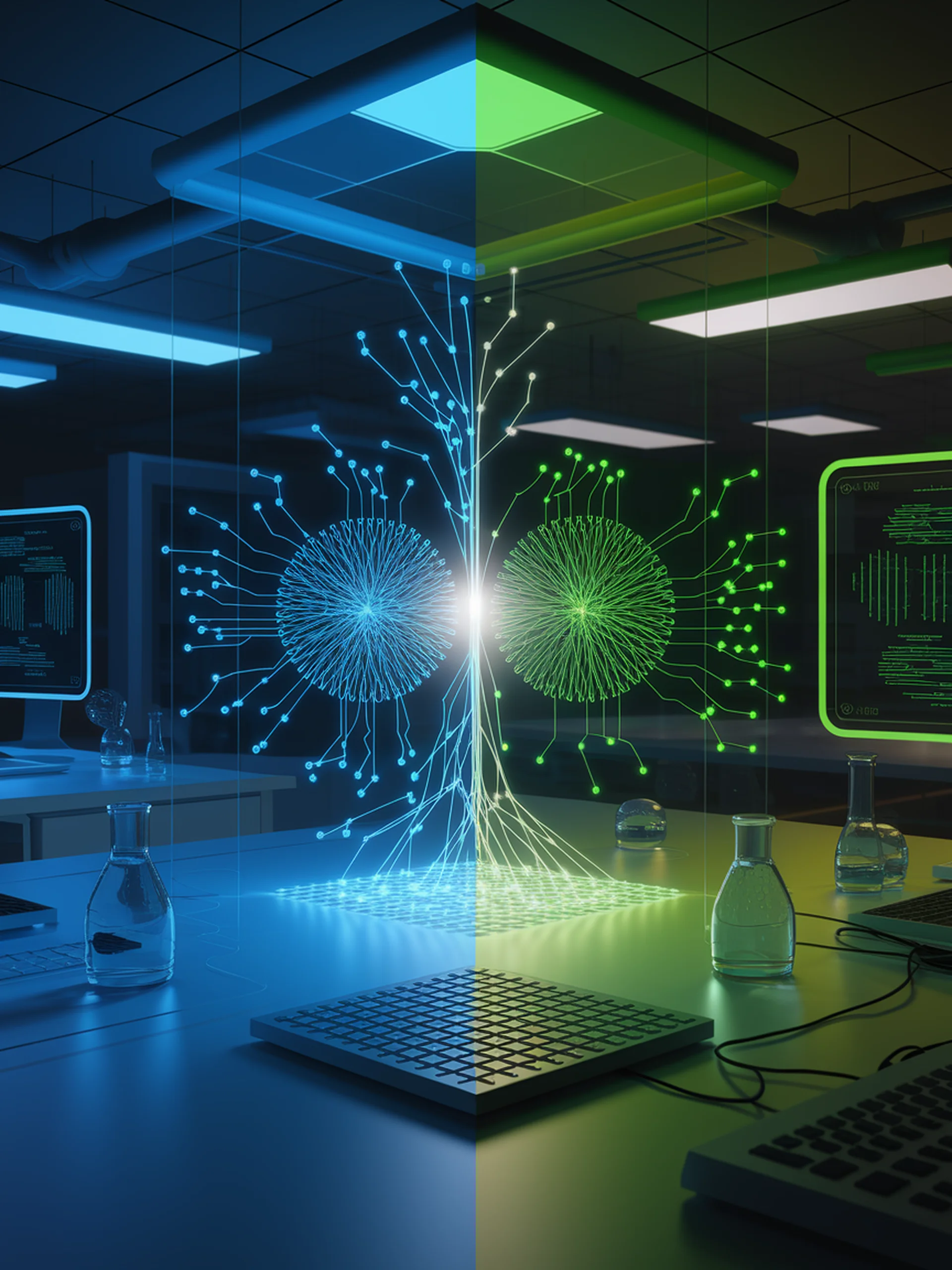
SafeMERGE: Preserving AI Safety During Fine-Tuning
Selective layer merging technique maintains safety without compromising performance
SafeMERGE addresses a critical challenge in LLM deployment: maintaining safety guardrails while fine-tuning models for specific applications. This novel approach selectively merges layers from safety-aligned and task-optimized models.
Key Innovations:
- Preserves safety alignment that typically deteriorates during standard fine-tuning
- Uses cosine similarity to identify and merge only layers that deviate from safe behavior
- Maintains task performance while significantly reducing harmful outputs
- Works as a post-fine-tuning technique, making it adaptable to various models
Medical Impact: SafeMERGE shows promising results on medical datasets like PubMedQA, enabling safer deployment of LLMs in healthcare settings where accuracy and safety are paramount.
This approach offers a practical solution for organizations seeking to customize AI capabilities while maintaining strong safety guardrails.