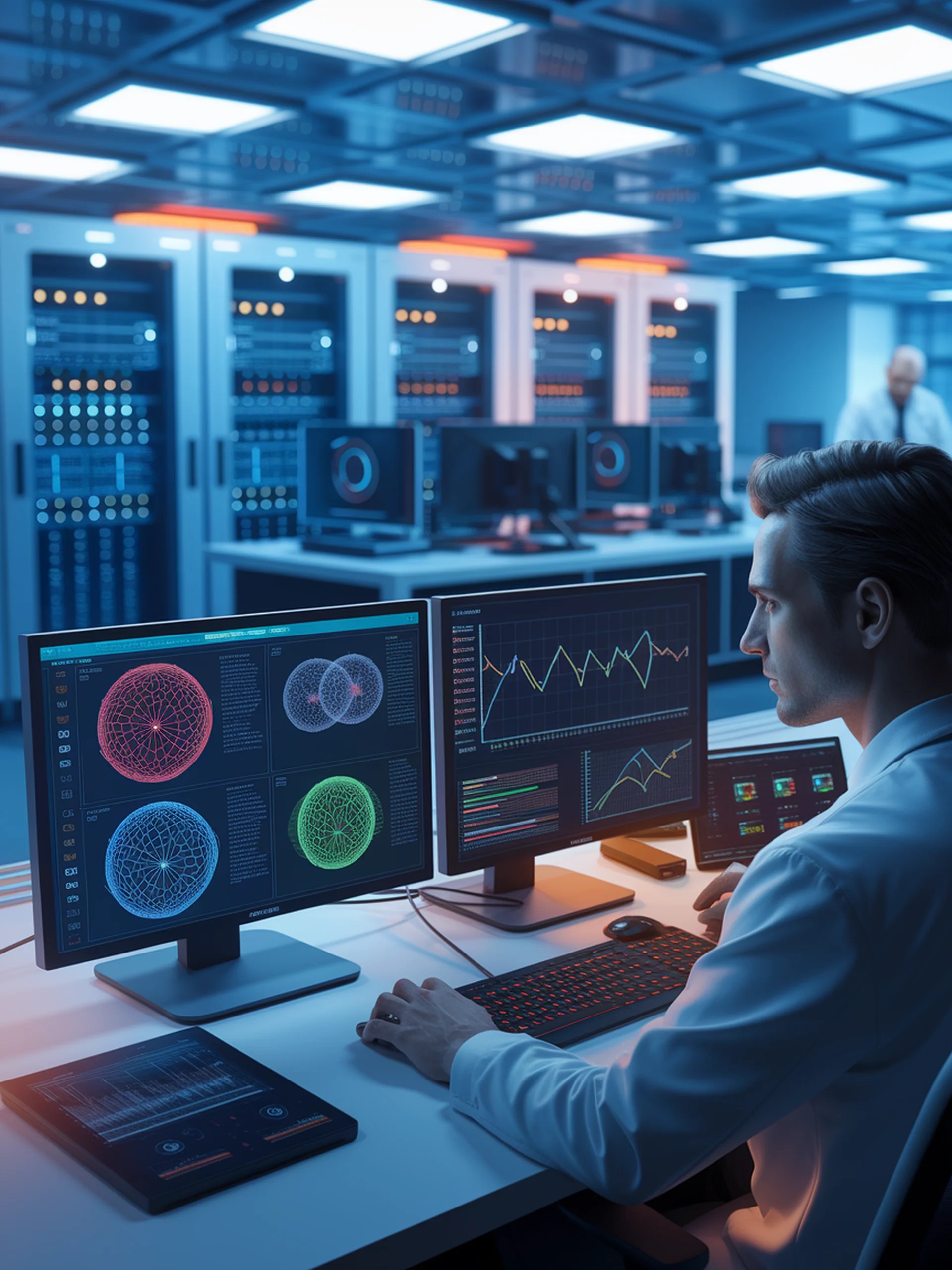
Combating Reward Hacking in AI Alignment
Systematic approaches to reward shaping for safer RLHF
This research tackles reward hacking - a critical challenge in Reinforcement Learning from Human Feedback (RLHF) where AI models exploit flaws in reward functions rather than learning intended behaviors.
Key contributions:
- Provides a systematic investigation of reward shaping techniques to mitigate reward hacking
- Demonstrates how properly designed reward shaping can enhance model alignment
- Identifies specific methods that stabilize RLHF while reducing exploitation
Security implications: By addressing reward hacking, this research contributes directly to making LLMs more secure and reliable, reducing risks of models finding unintended shortcuts that undermine alignment with human values.