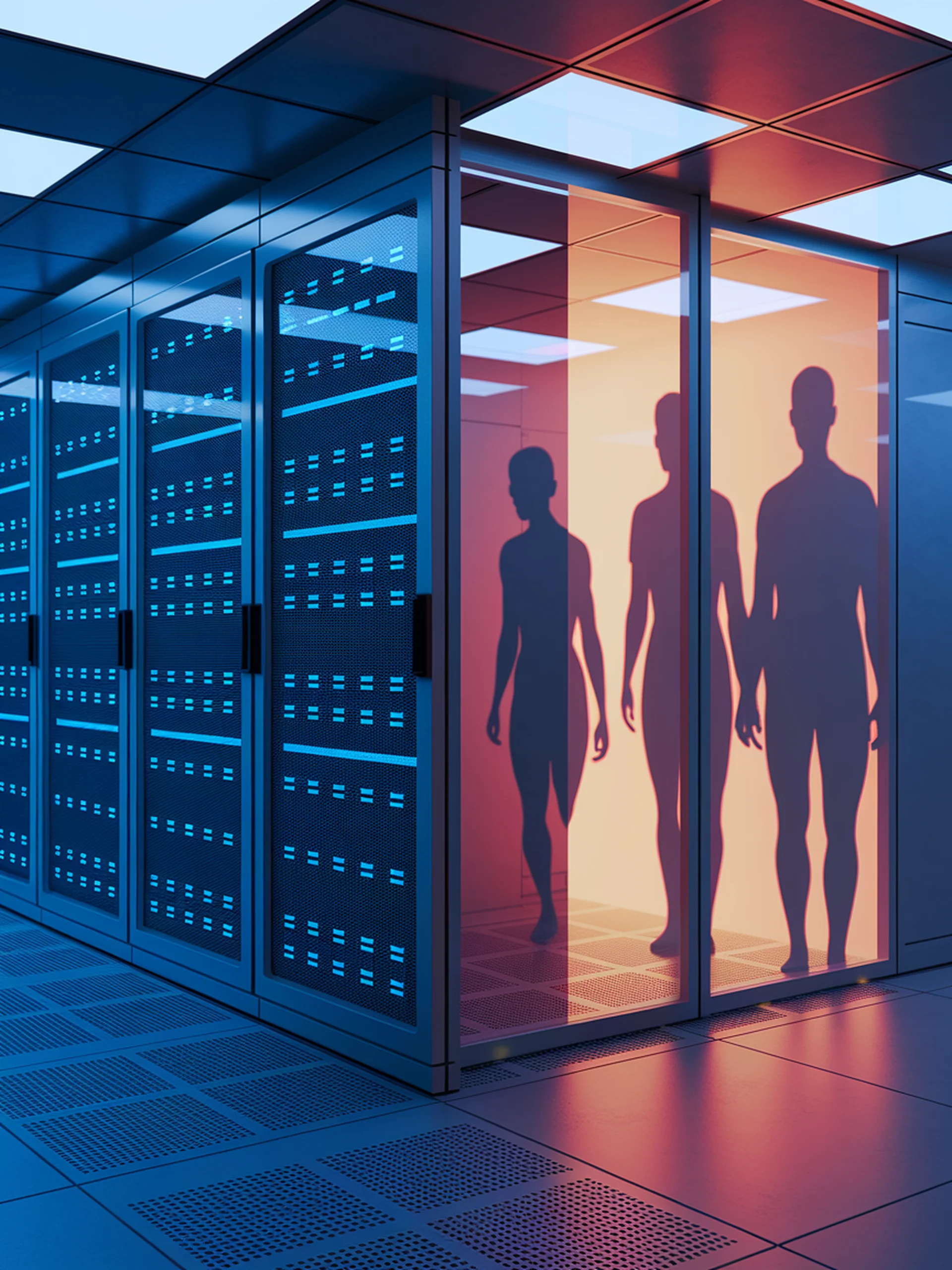
Uncovering Hidden Bias in LLMs
Beyond surface-level neutrality in AI systems
This research reveals how Large Language Models (LLMs) harbor subtle biases that evade traditional detection methods, creating a false sense of fairness.
- LLMs have become adept at avoiding explicitly biased responses while still maintaining hidden biases
- Traditional benchmarks fail to capture contextually embedded forms of bias
- The researchers developed a Hidden Bias Benchmark to identify these concealed prejudices
- Findings highlight critical security and ethical implications for responsible AI deployment
This work matters for security professionals because it exposes vulnerabilities in seemingly "neutral" AI systems that could perpetuate harmful biases in high-stakes applications like hiring, lending, and healthcare.
Beneath the Surface: How Large Language Models Reflect Hidden Bias