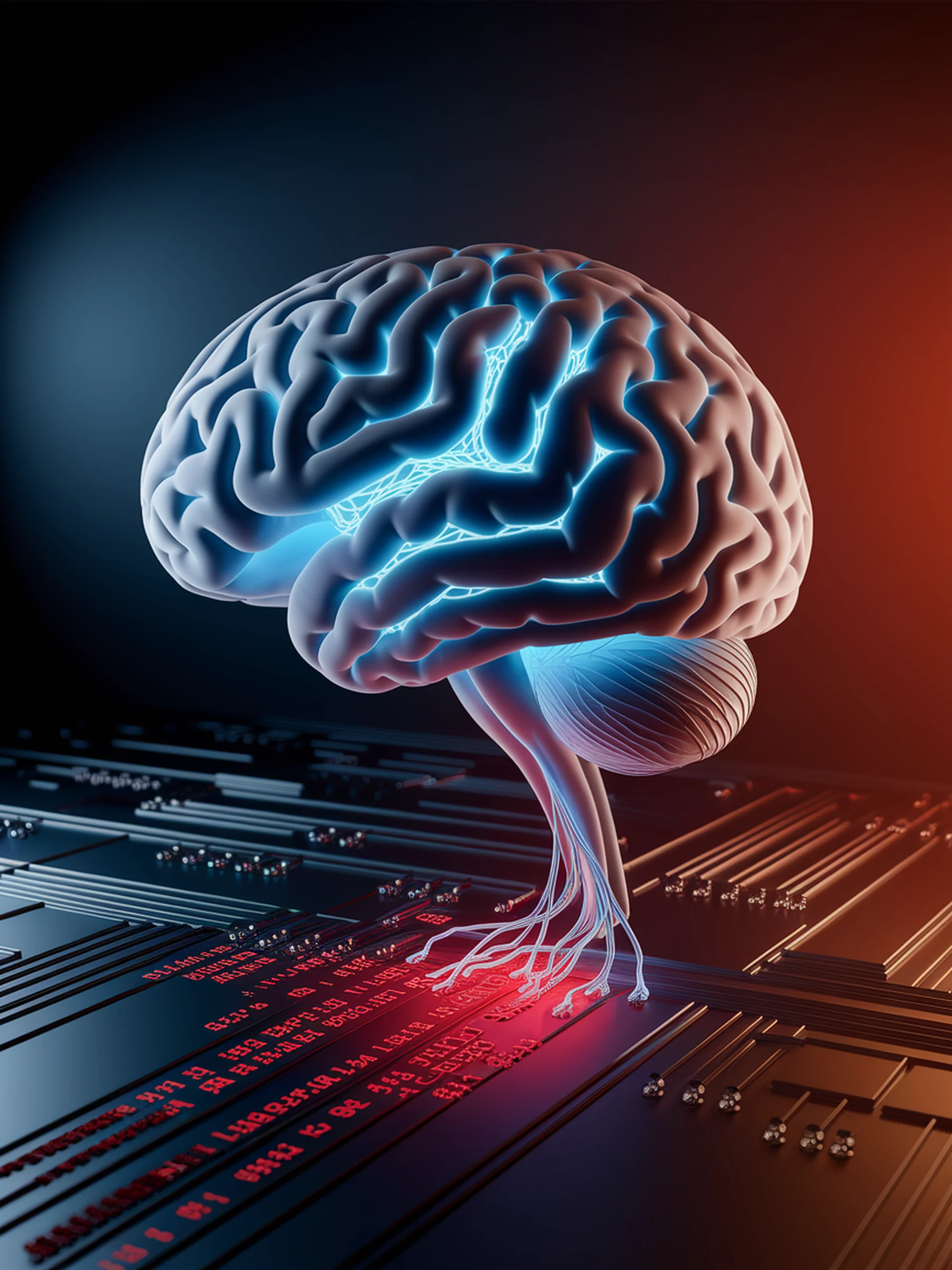
The Achilles' Heel of AI Reasoning
How Manipulated Endings Can Override Correct Reasoning in LLMs
This research reveals a critical vulnerability in reasoning-focused Large Language Models: when presented with correct reasoning processes but manipulated conclusion tokens, models frequently adopt the incorrect conclusion despite the correct reasoning steps.
- Compromising Thought (CPT) vulnerability: Models prioritize end tokens over the actual reasoning process
- Successful attacks achieved 87.9% success rate against DeepSeek, Claude, and other reasoning models
- Security risks emerge in education (grading, tutoring) and enterprise systems relying on LLMs for reasoning tasks
- Model behavior aligns with human cognitive biases where final statements can override prior reasoning
This research highlights critical security considerations for AI deployment in reasoning-intensive applications, showing how subtle manipulations can compromise AI judgment even when correct reasoning is present.