Security in Federated Learning for LLMs
Research on security challenges, attack vectors, and defensive mechanisms in federated learning environments for large language models
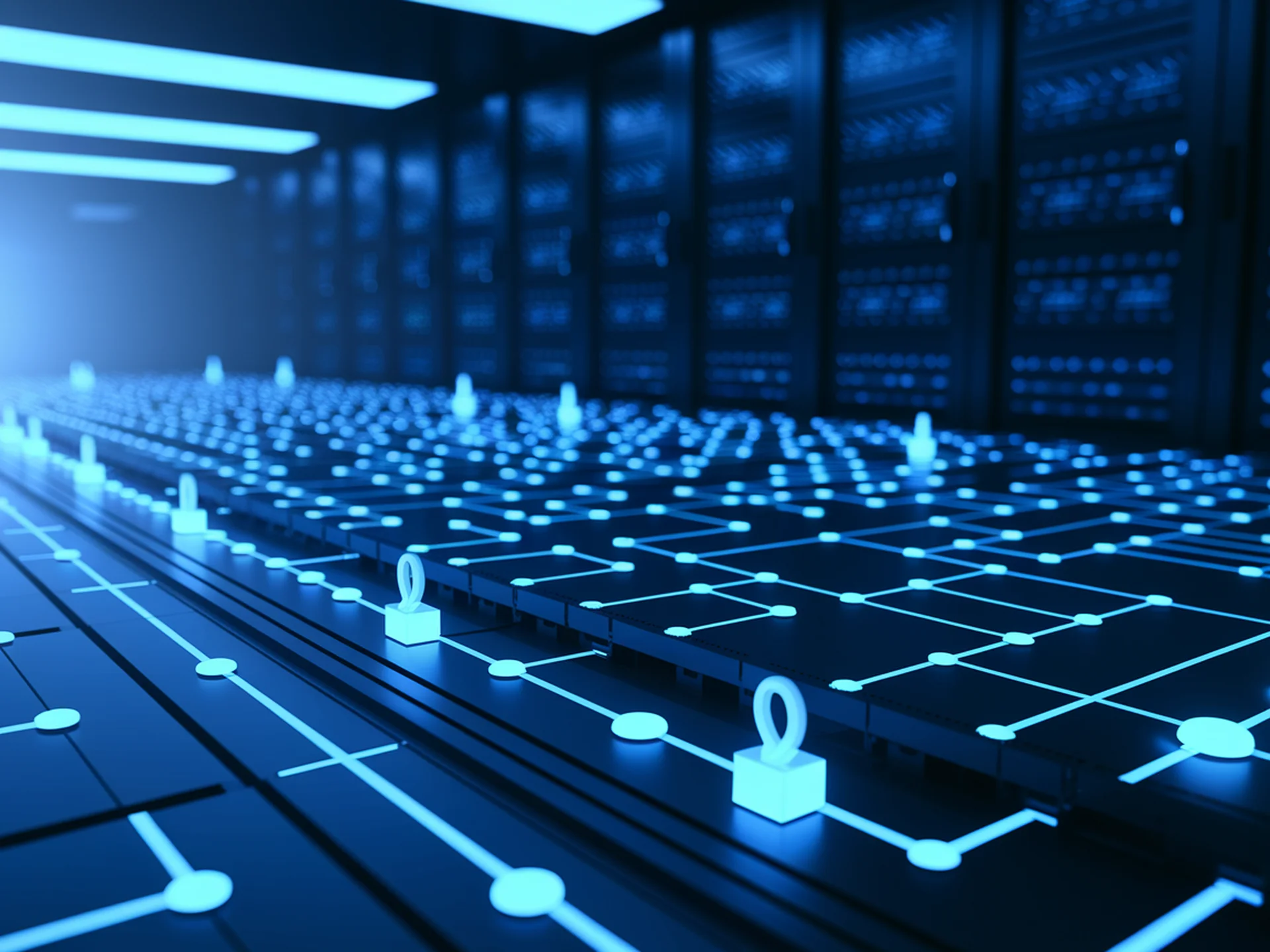
Security in Federated Learning for LLMs
Research on Large Language Models in Security in Federated Learning for LLMs
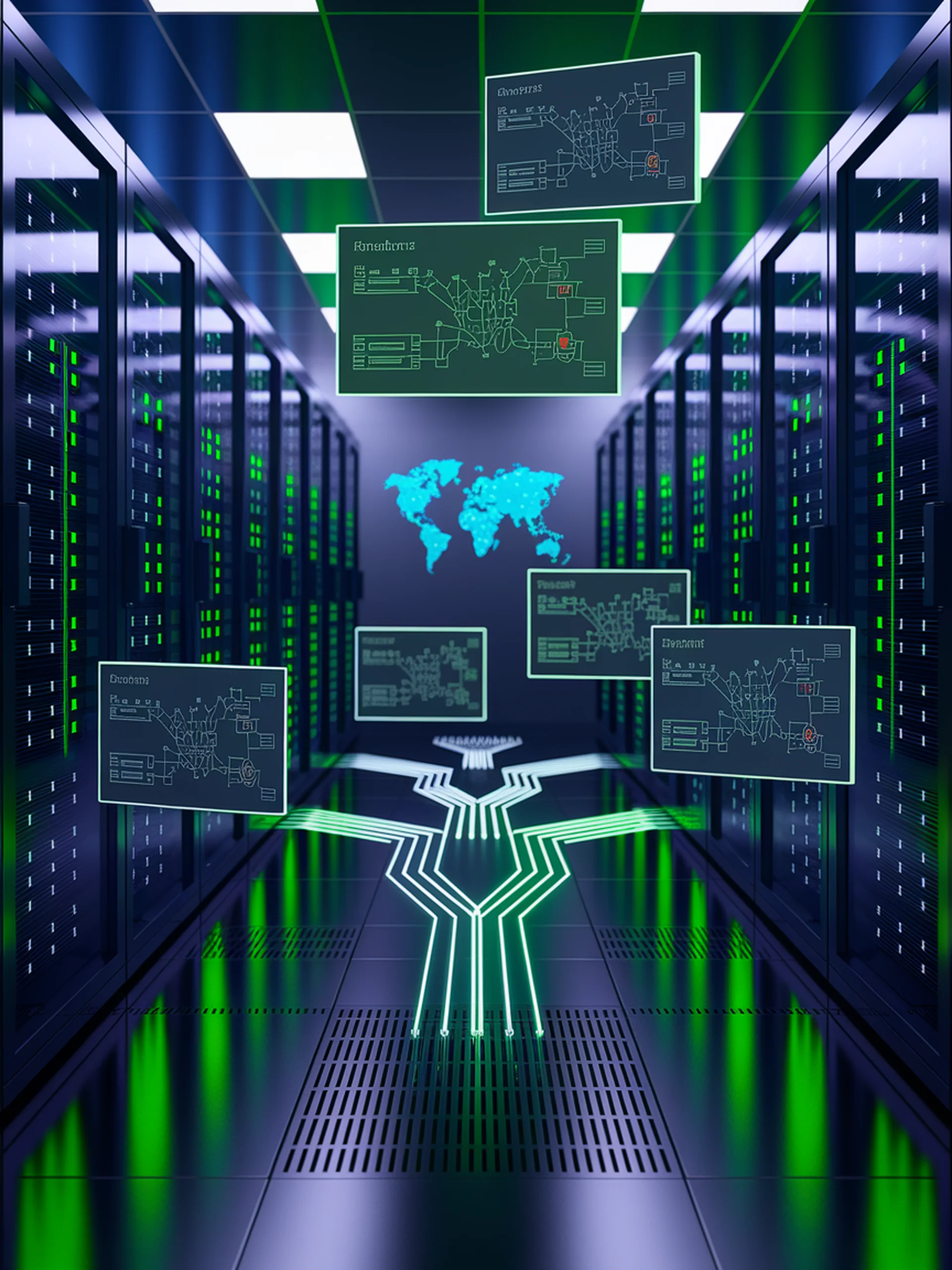
Securing Federated LLMs
A Novel Framework for Enhanced Robustness Against Adversarial Attacks
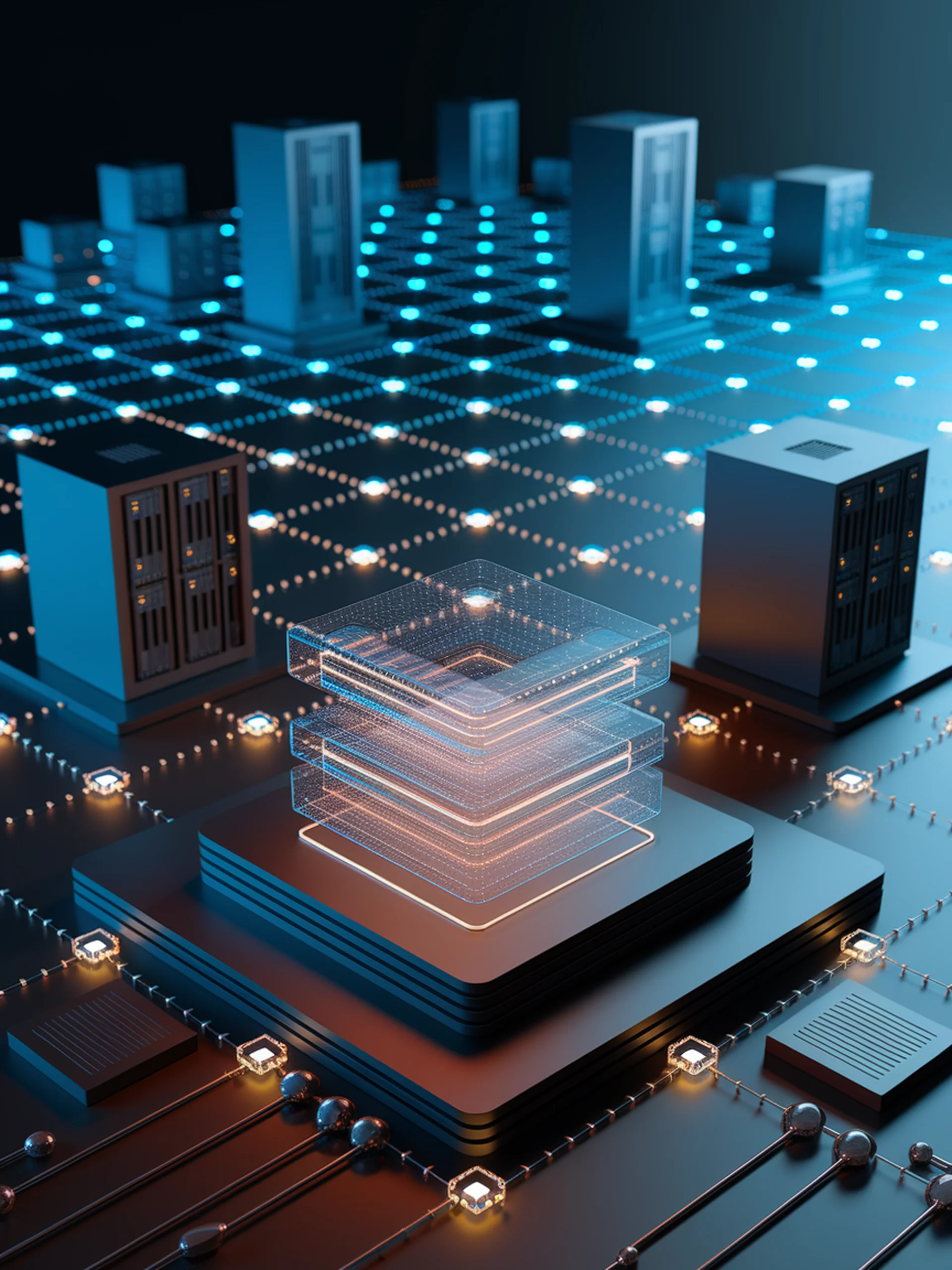
Blockchain-Powered Federated Learning
A Decentralized Framework for Secure, Incentivized Model Training
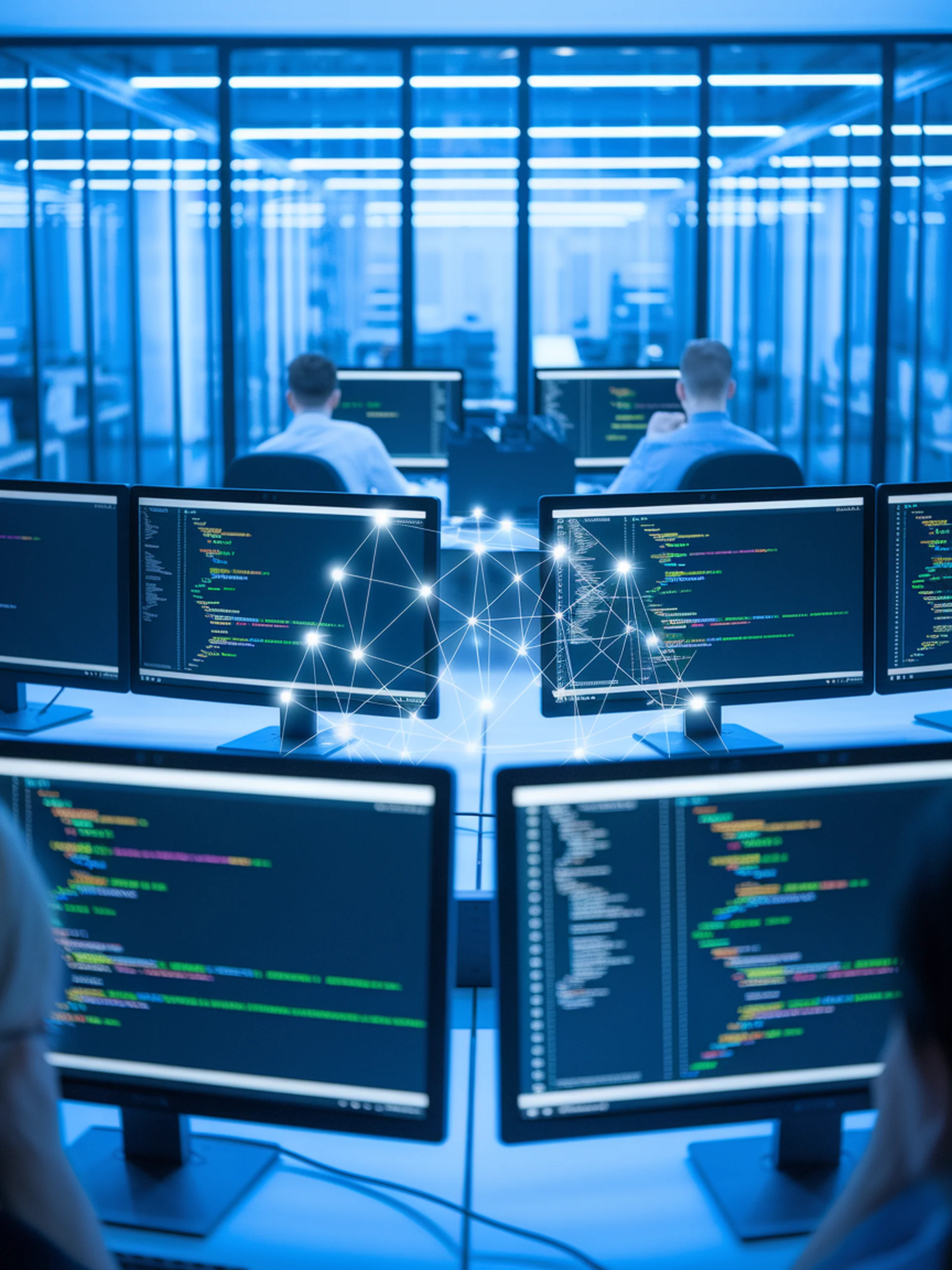
Federated Knowledge Editing for LLMs
Privacy-preserving collaborative model updates without retraining
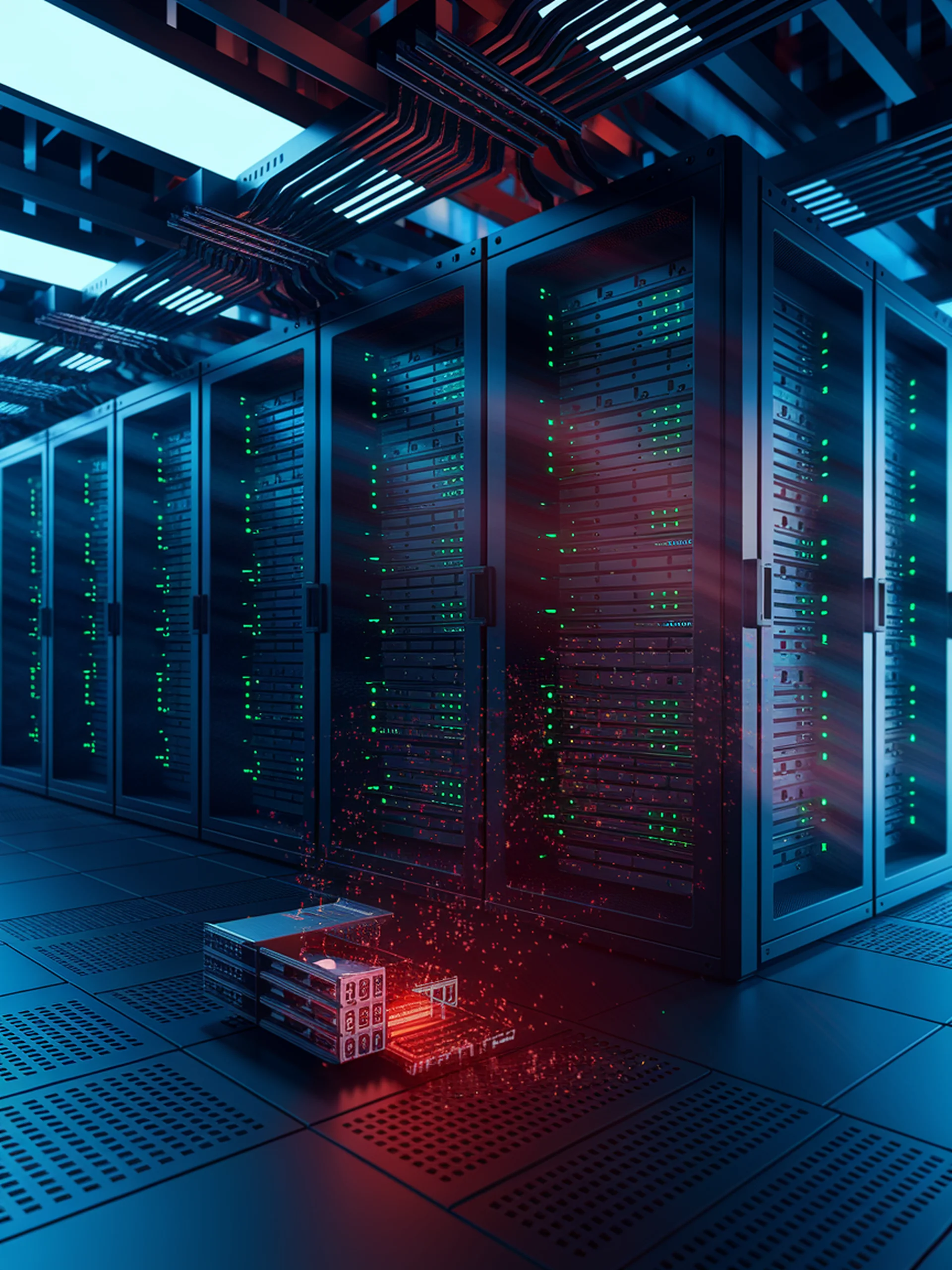
Data Theft Vulnerability in Decentralized LLM Training
Novel attack exposes private training data in distributed systems
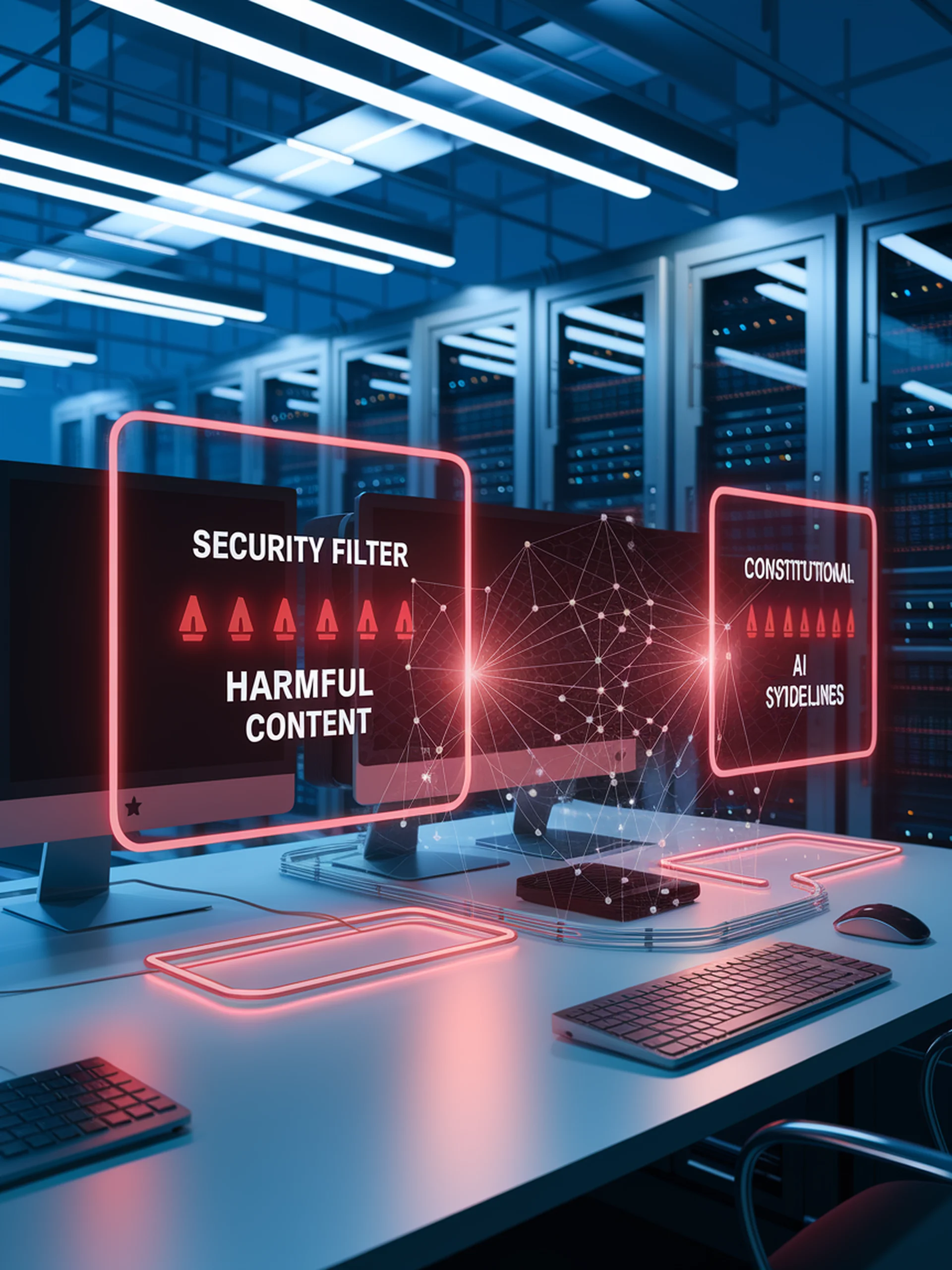
Securing Federated Large Language Models
Combining Safety Filters and Constitutional AI for Responsible AI Deployment
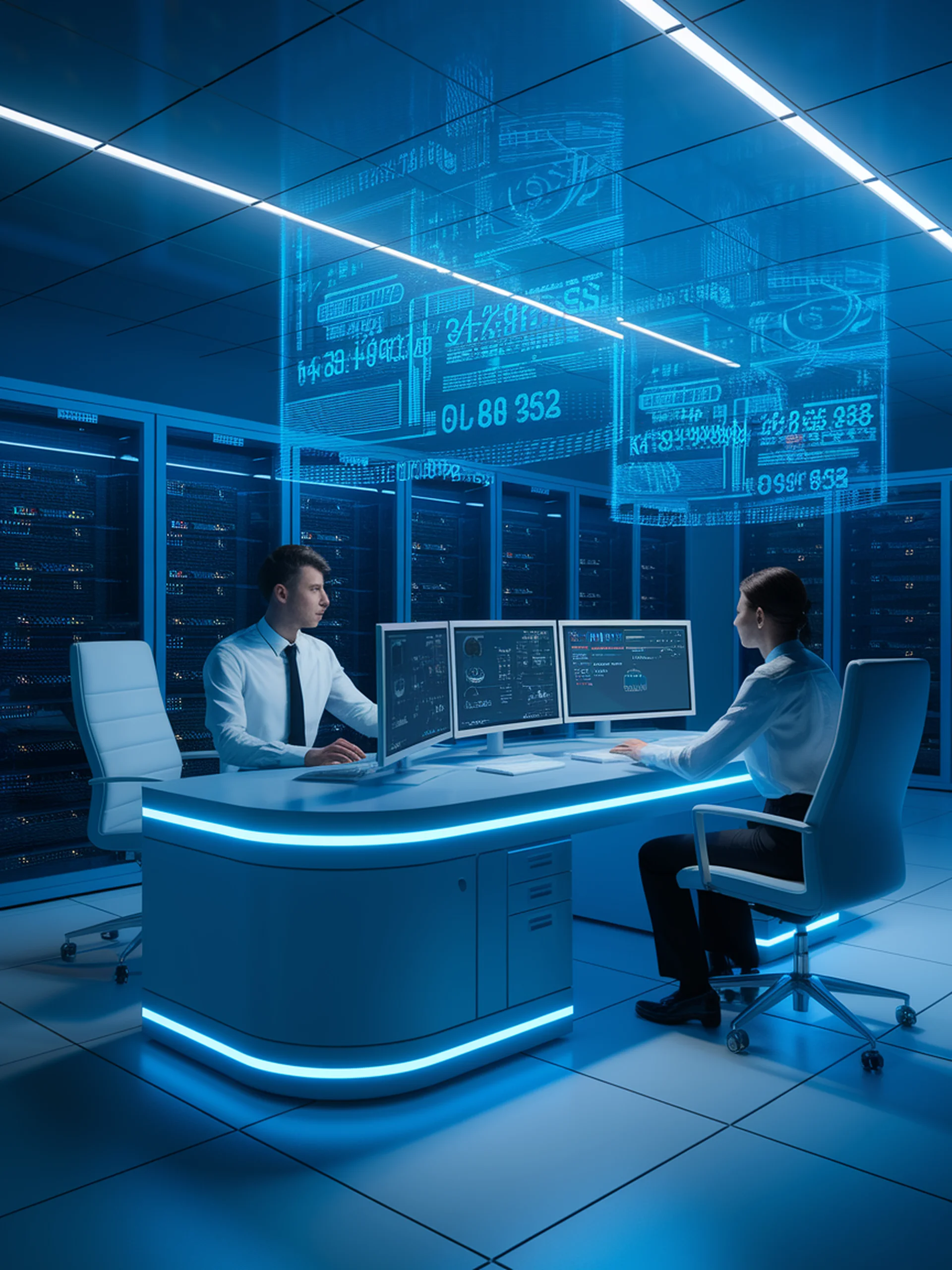
Next-Gen Security Threat Detection
Combining Federated Learning with Multimodal LLMs
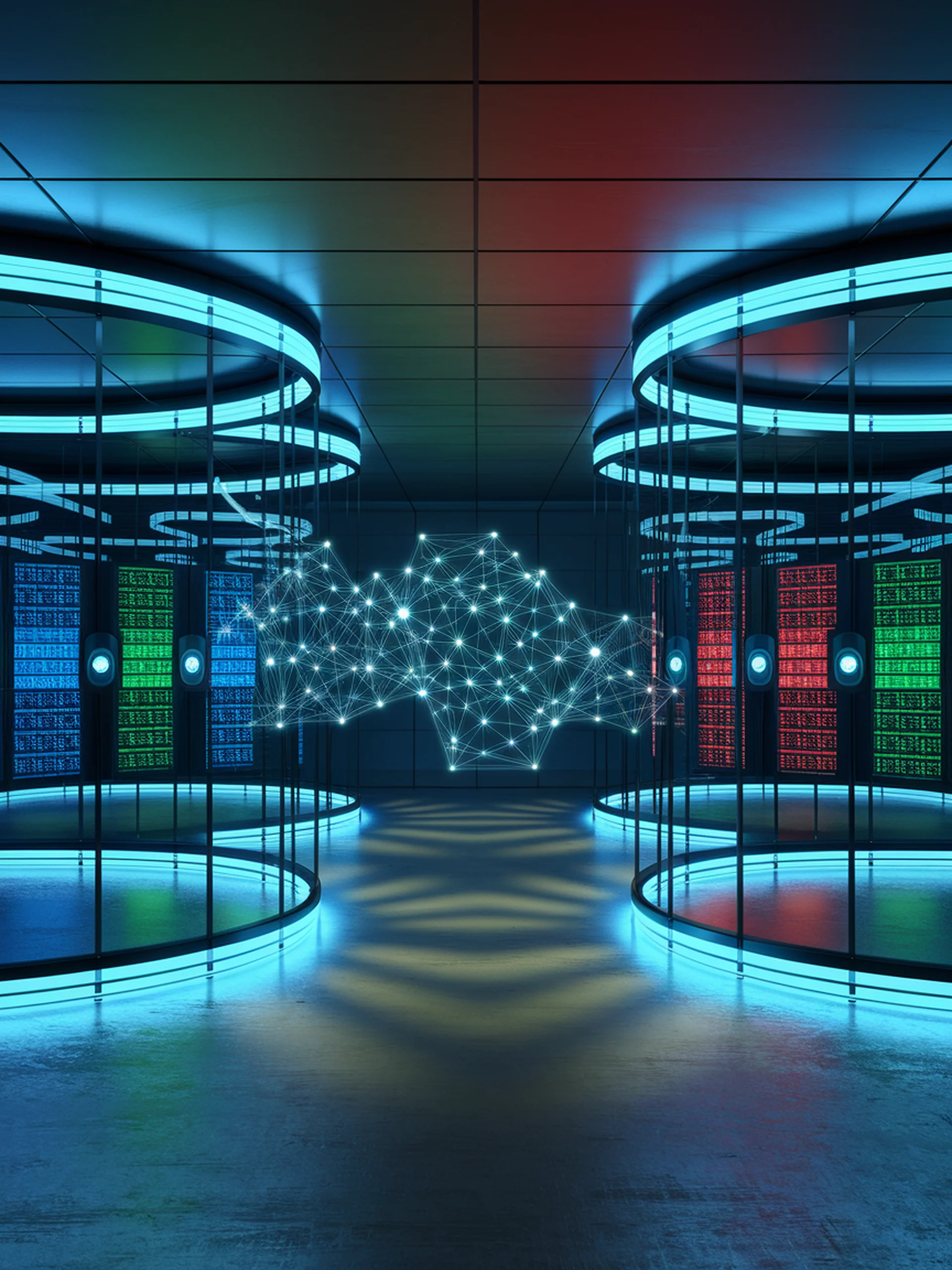
Secure LLM Fine-Tuning Across Organizations
HLoRA: A Resource-Efficient Federated Learning Approach for LLMs
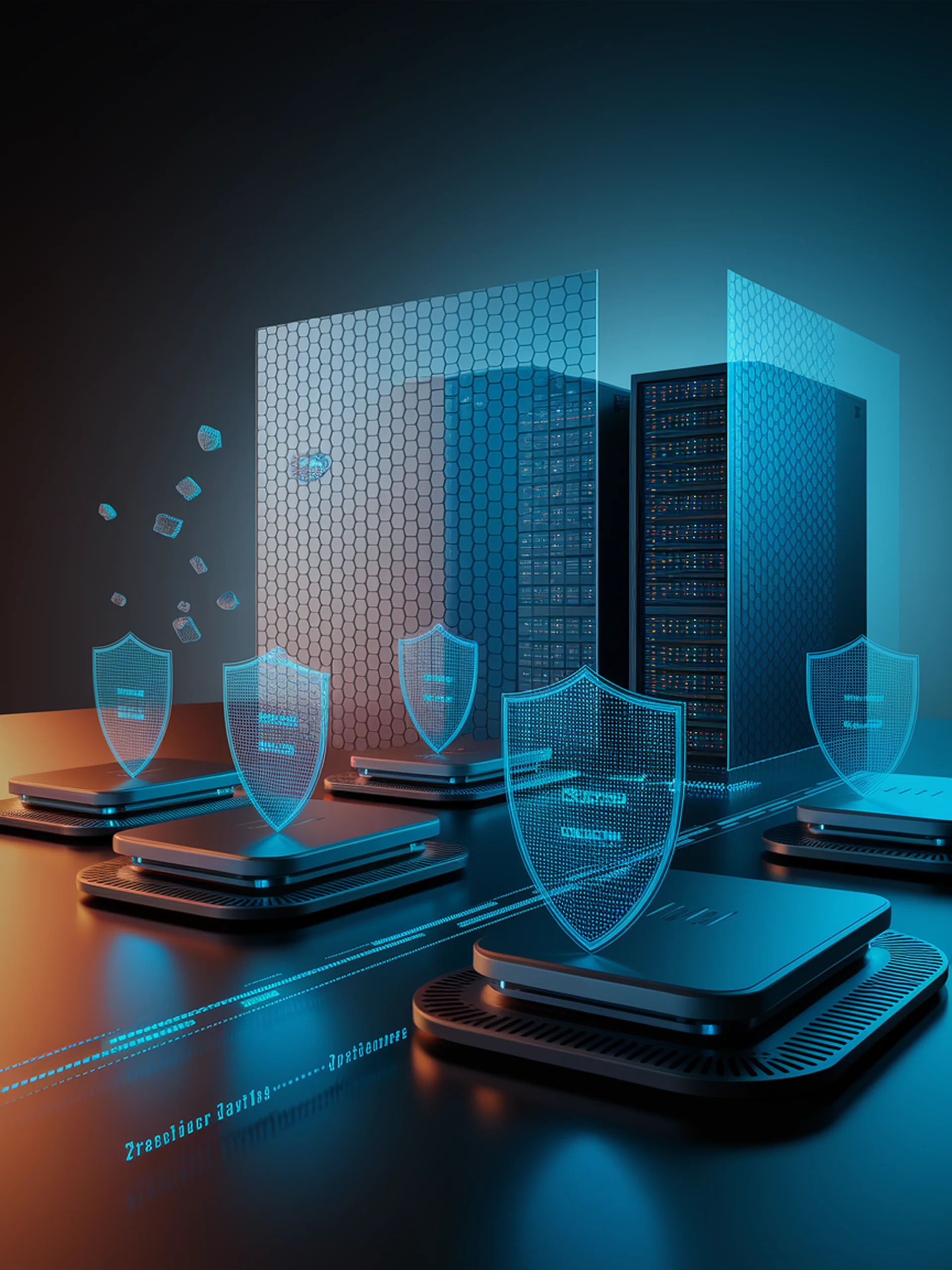
Securing LLM Fine-tuning in Distributed Settings
Privacy-preserving technique using Function Secret Sharing
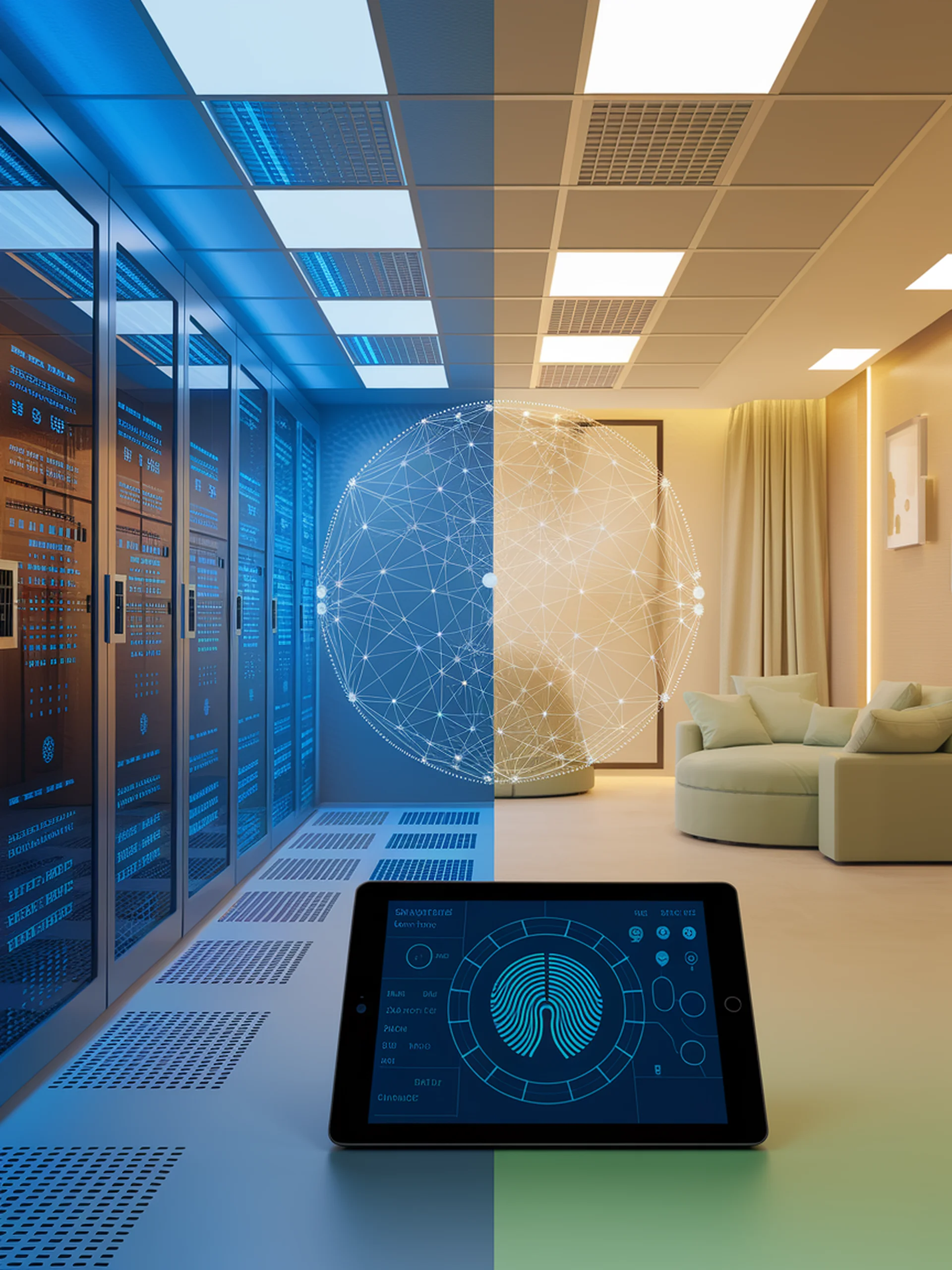
Safeguarding Mental Health Data with AI
Privacy-Preserving LLMs for Mental Health Analysis
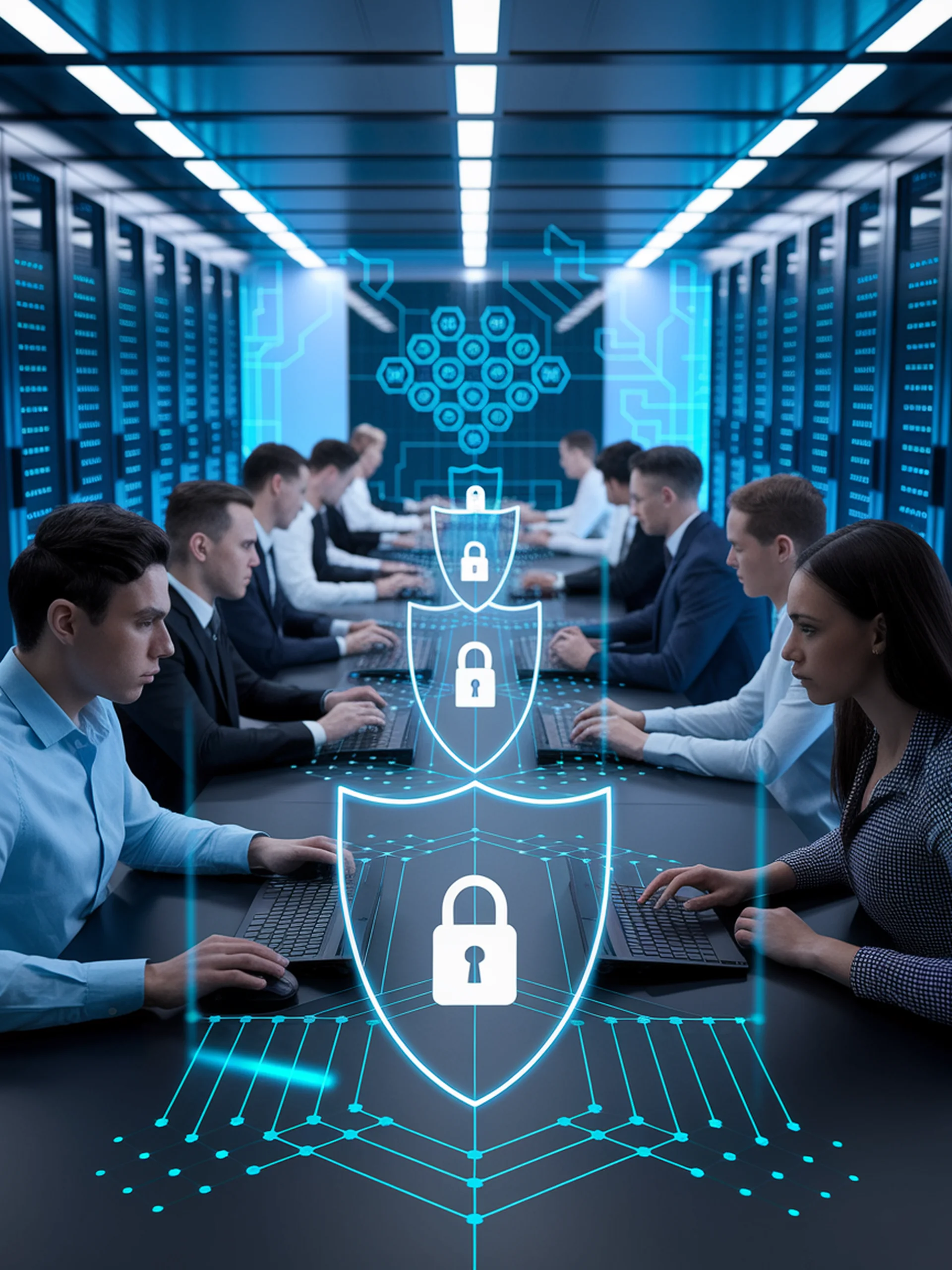
Privacy-Preserving LLM Alignment
Aligning AI with diverse human values without compromising privacy
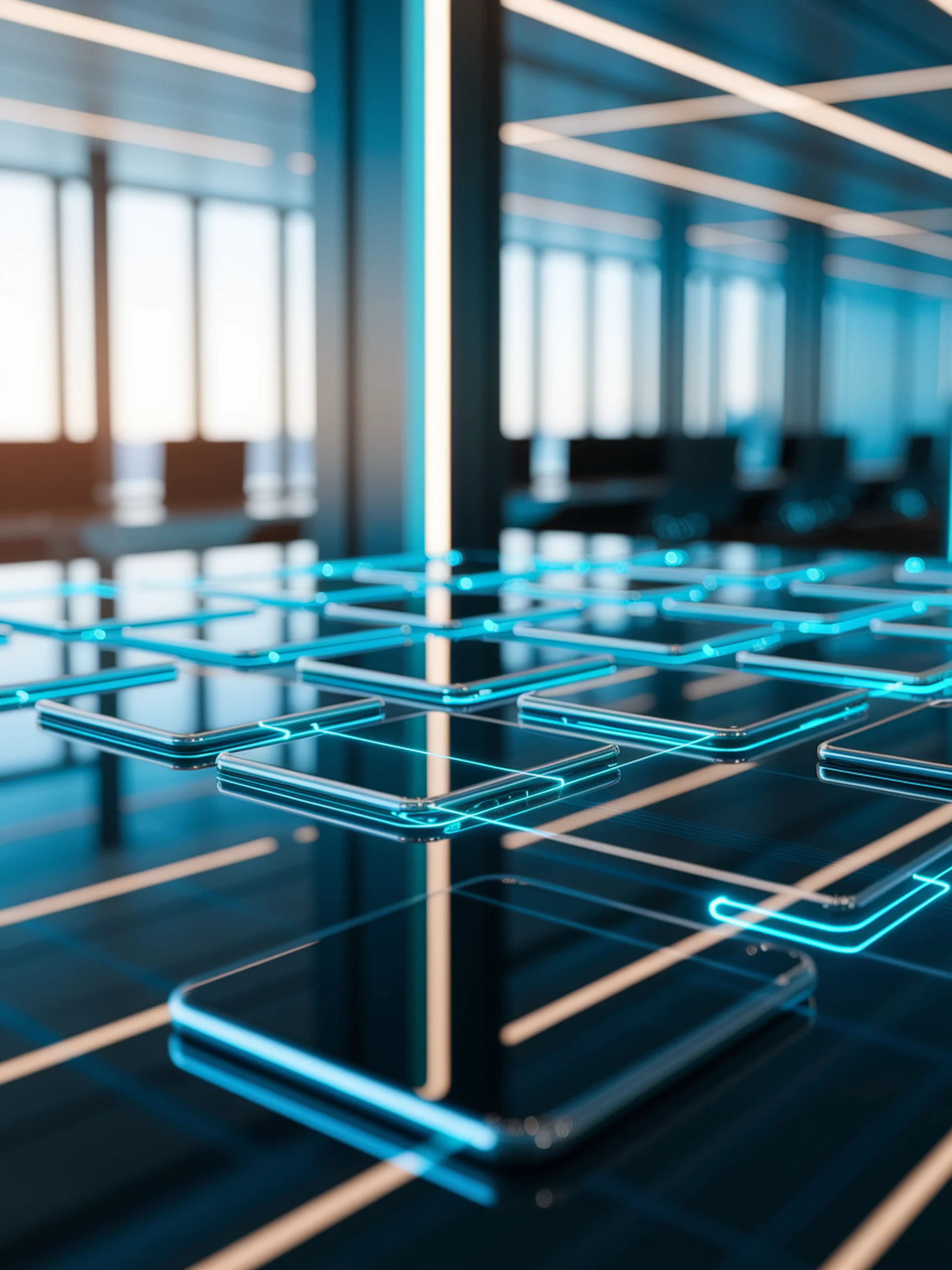
Beyond Centralized Models: Decentralized Federated Learning
Enhancing privacy, robustness, and performance in distributed ML systems
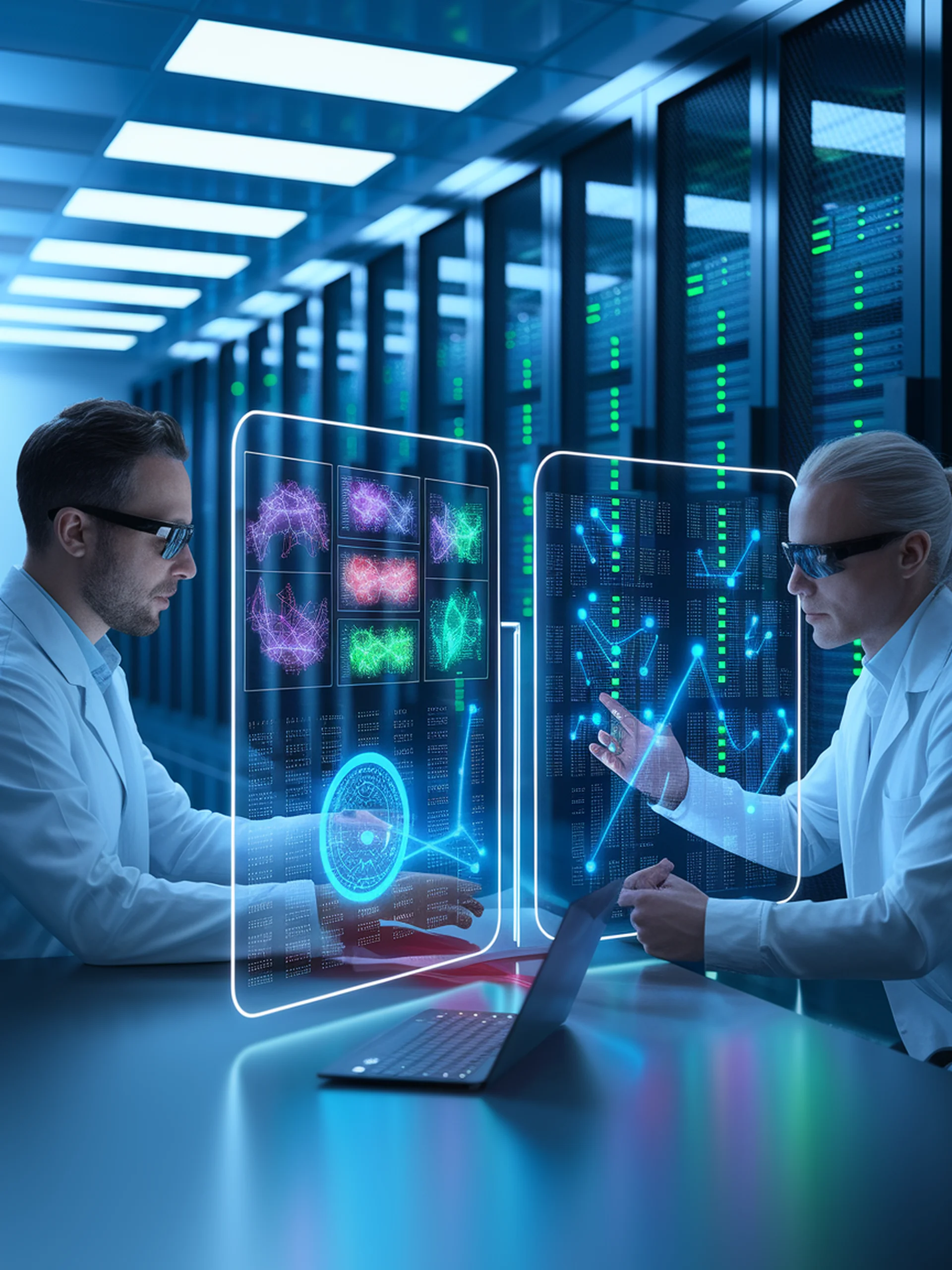
Federated LLMs: Private & Collaborative AI
How Federated Learning enables privacy-preserving LLM adaptation
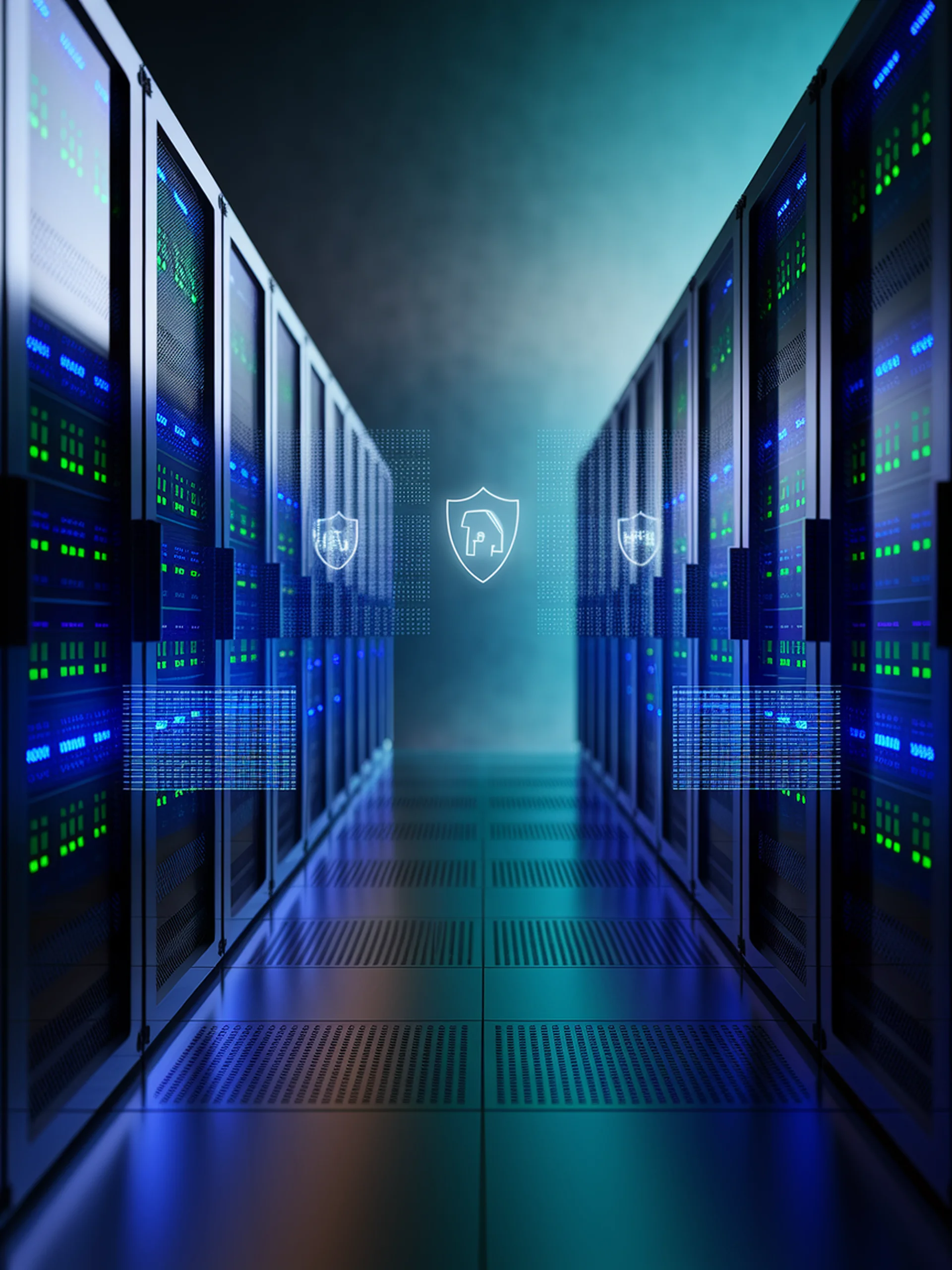
Securing LLMs Across Cloud Boundaries
A Federated Learning Framework for Cross-Cloud Privacy Protection
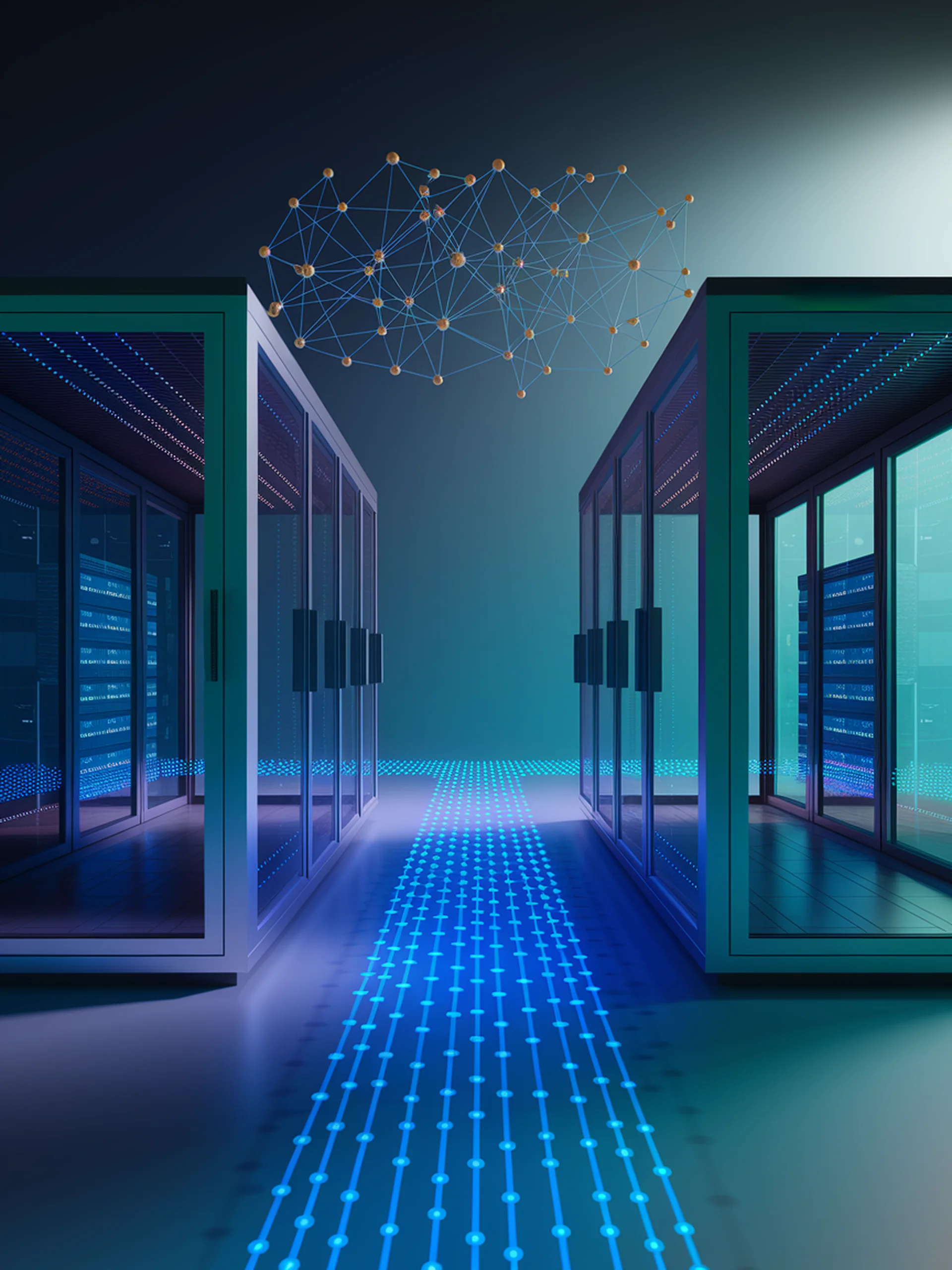
Privacy-Preserving CTR Prediction Across Domains
Leveraging LLMs to enhance federated learning for cross-domain recommendations
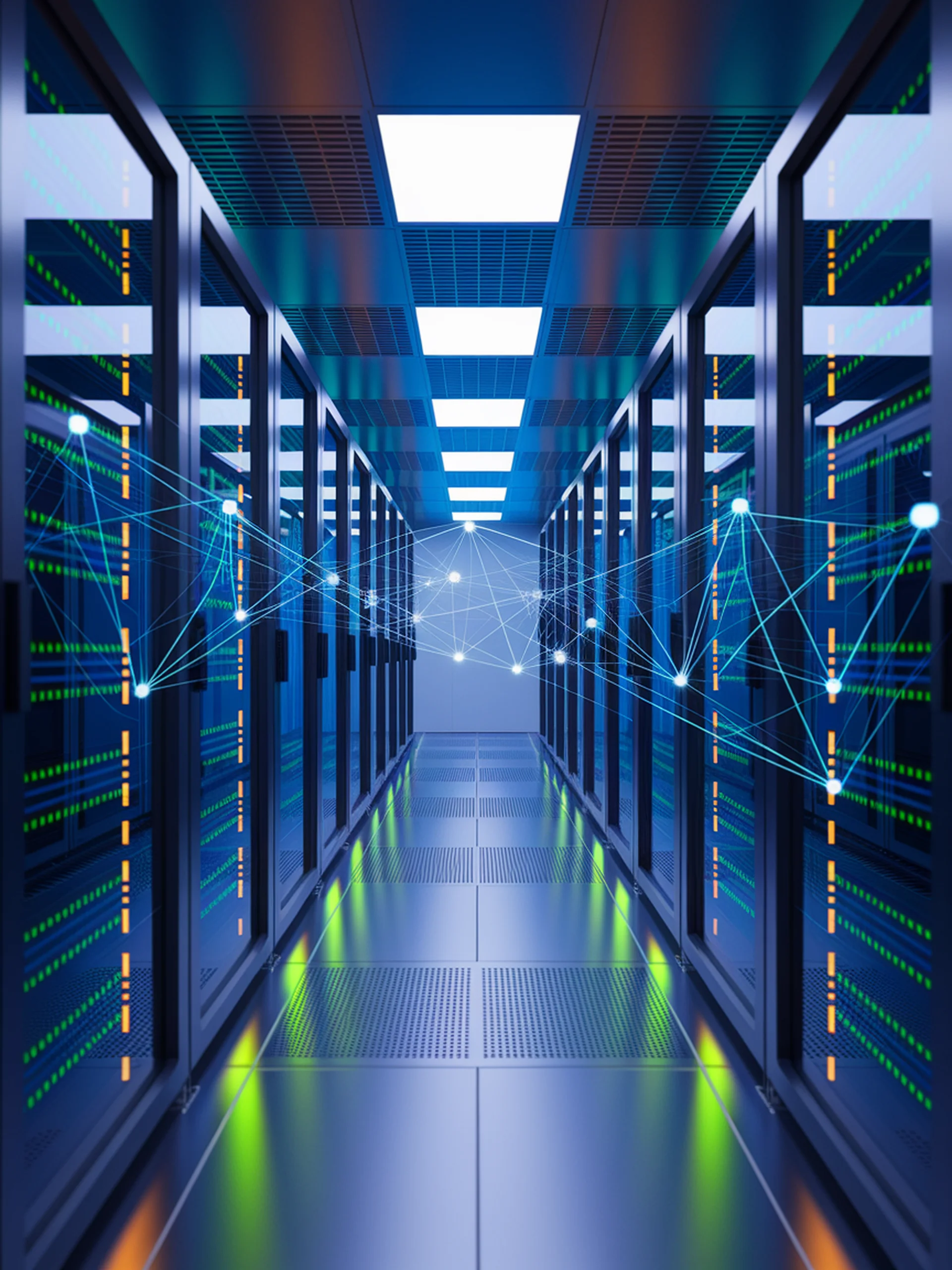
Securing Graph Learning with LLMs
A privacy-preserving approach to federated graph learning using large language models
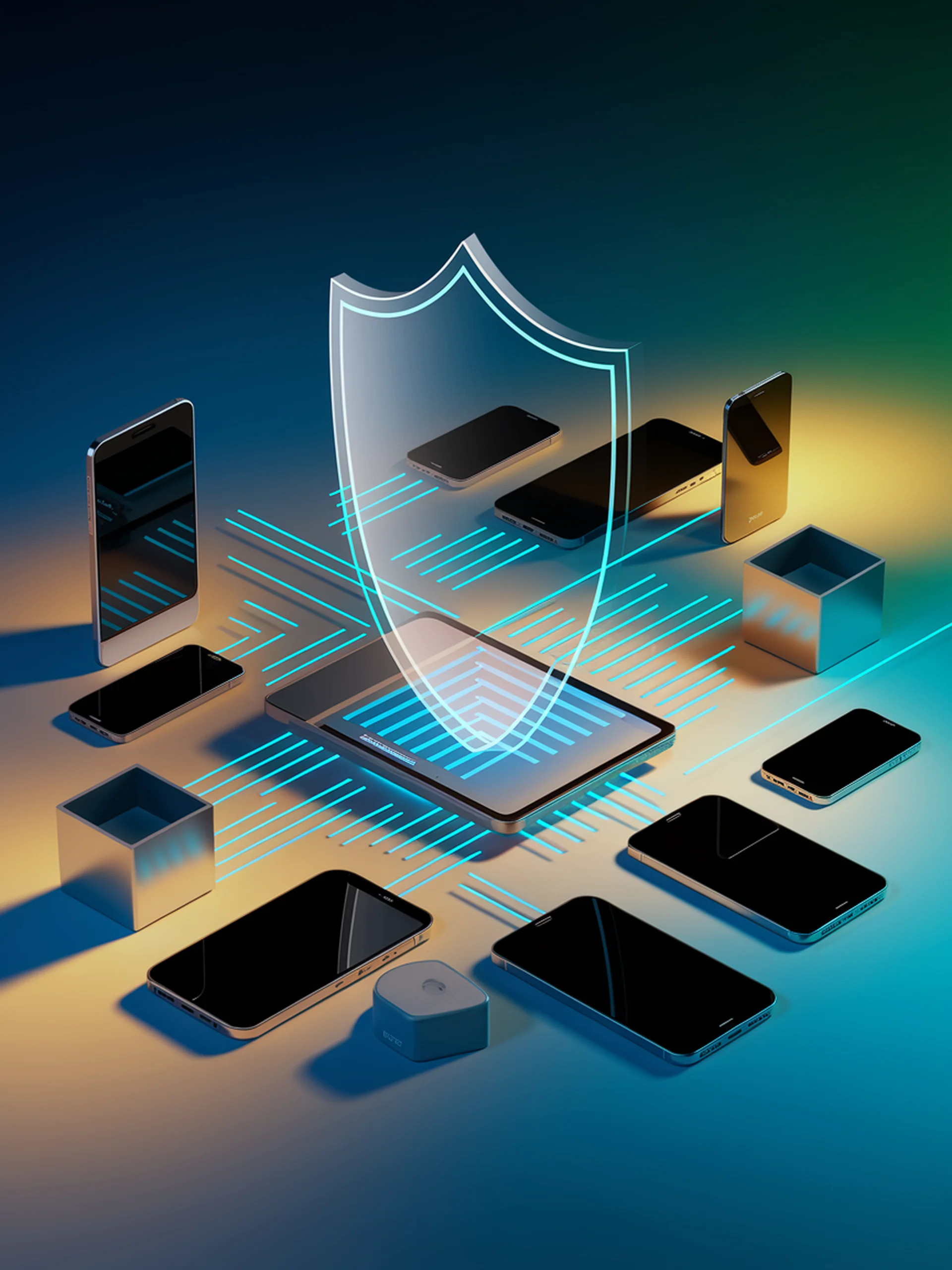
Securing Federated Learning at the Edge
Building Resilient Collaborative AI Systems for Decentralized Environments
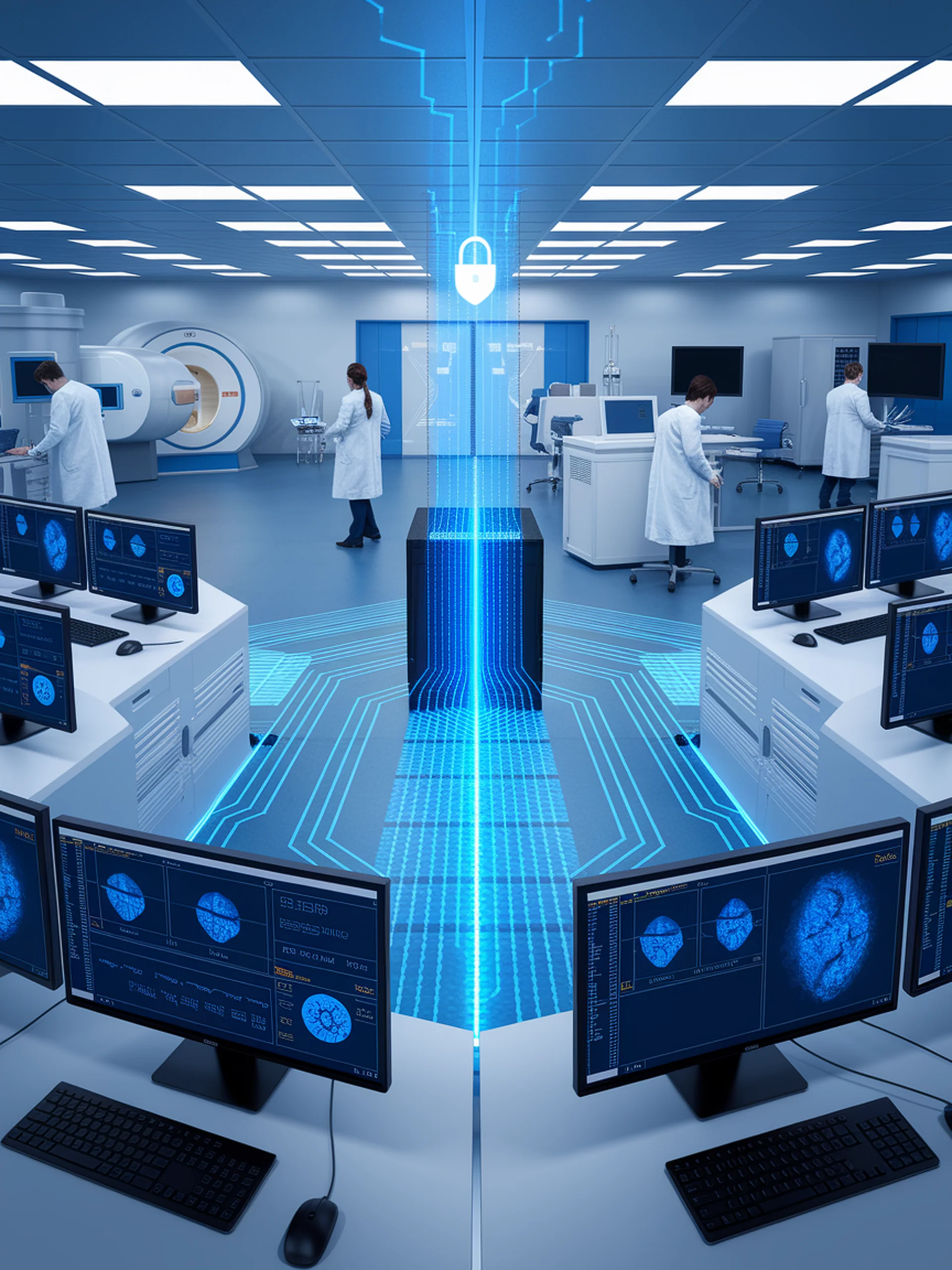
Efficient Federated Learning for Healthcare LLMs
Designing Faster, Privacy-Preserving Models with Layer-Skipping
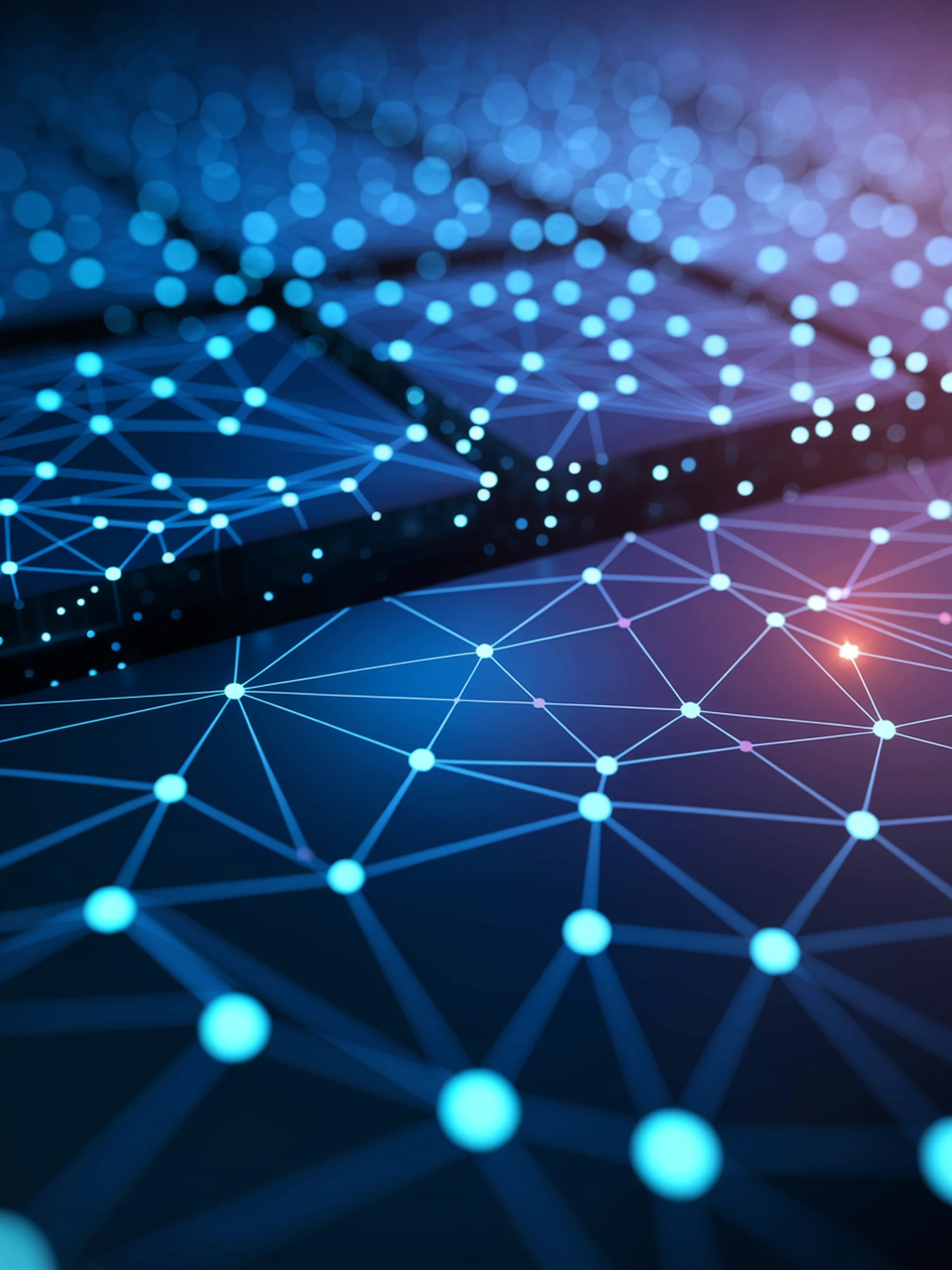