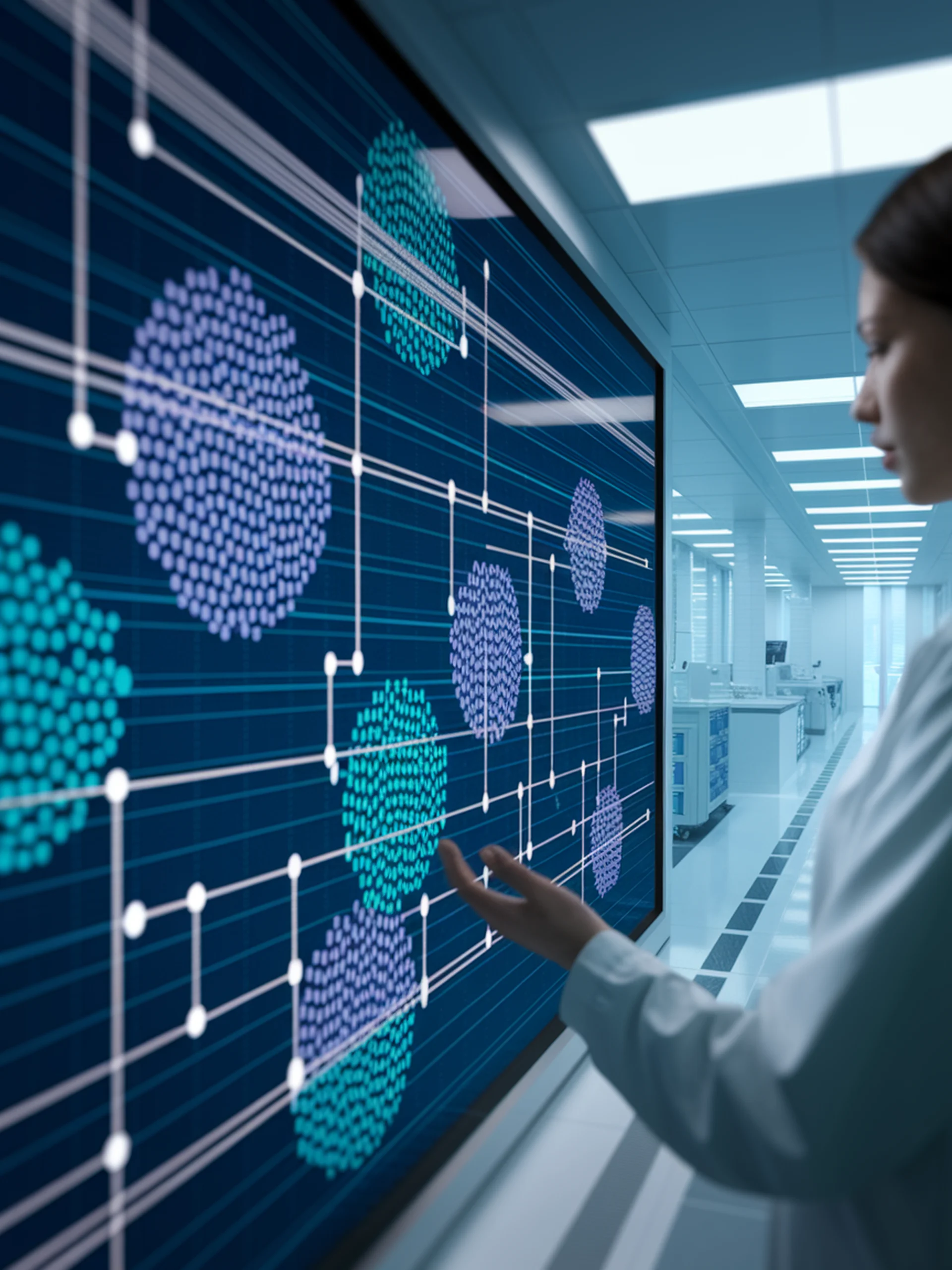
Synthetic Tabular Data with Real-World Logic
Preserving Inter-column Relationships in Generated Data
LLM-TabFlow introduces a novel approach to generating synthetic tabular data that maintains logical relationships between columns, addressing a key challenge in data synthesis.
- Leverages Large Language Model reasoning to capture complex relationships in tabular data
- Enables high-quality synthetic data generation for privacy-sensitive domains
- Preserves inter-column logical relationships better than existing generative models
- Applicable across healthcare, finance, and supply chain contexts
For healthcare organizations, this research enables realistic patient data generation without compromising privacy, supporting both AI model training and data sharing while maintaining HIPAA compliance.
LLM-TabFlow: Synthetic Tabular Data Generation with Inter-column Logical Relationship Preservation