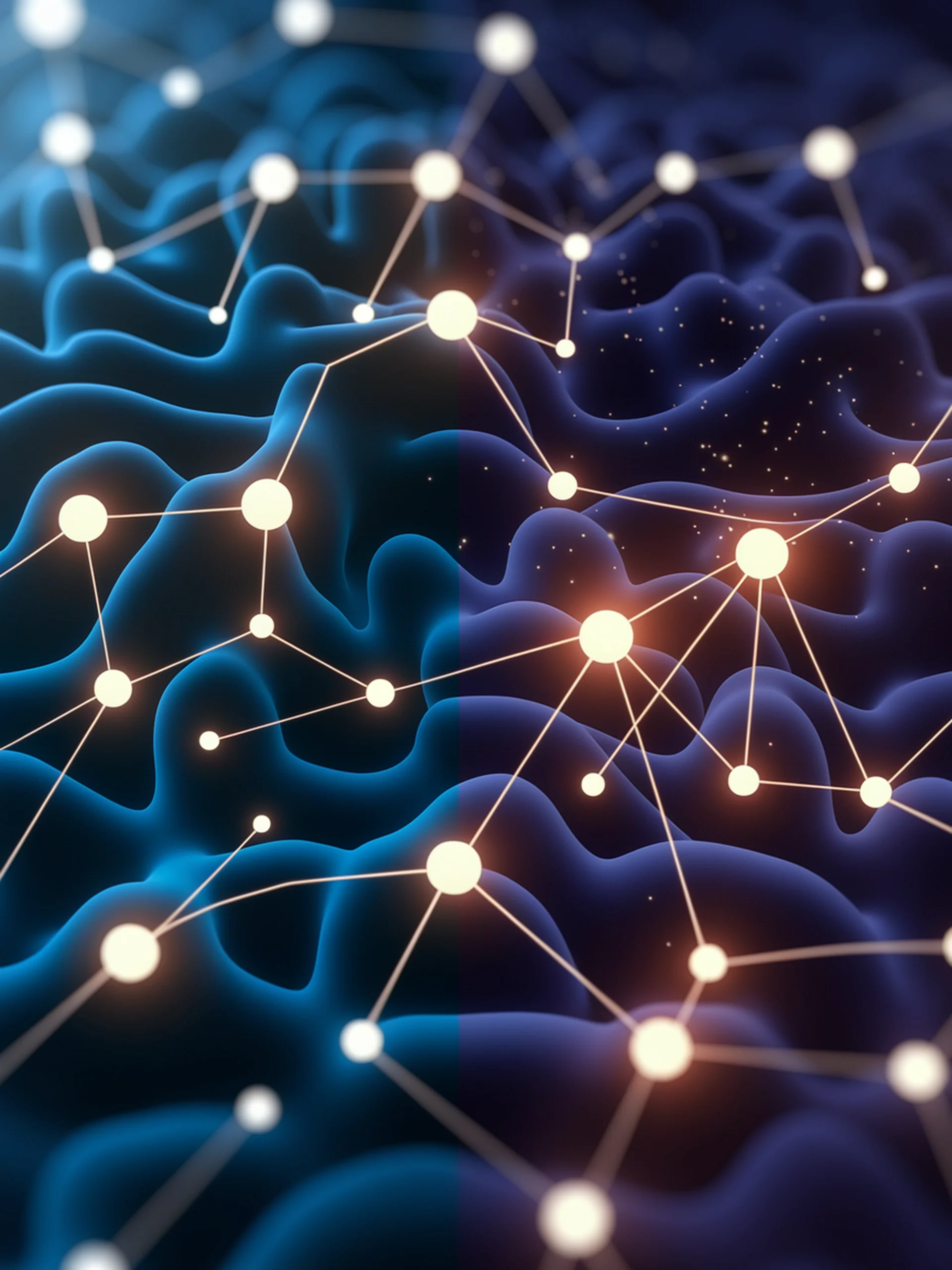
Repairing Bias in Language Models
A Novel Approach to Fairness Through Attention Pruning
This research introduces an efficient post-processing technique to mitigate bias in LLMs by selectively pruning attention heads, reducing gender bias by up to 40% without significantly impacting model performance.
Key Findings:
- Automated attention pruning offers a cost-effective alternative to retraining for bias mitigation
- Uses surrogate simulated annealing to identify which attention heads contribute most to bias
- Achieves 40% reduction in gender bias while maintaining 95% of original performance
- Provides practical tools for AI developers to improve fairness without access to training data
This work addresses critical security and ethical concerns by offering practical methods to reduce harmful biases in AI systems that are increasingly deployed in sensitive social contexts.
Attention Pruning: Automated Fairness Repair of Language Models via Surrogate Simulated Annealing