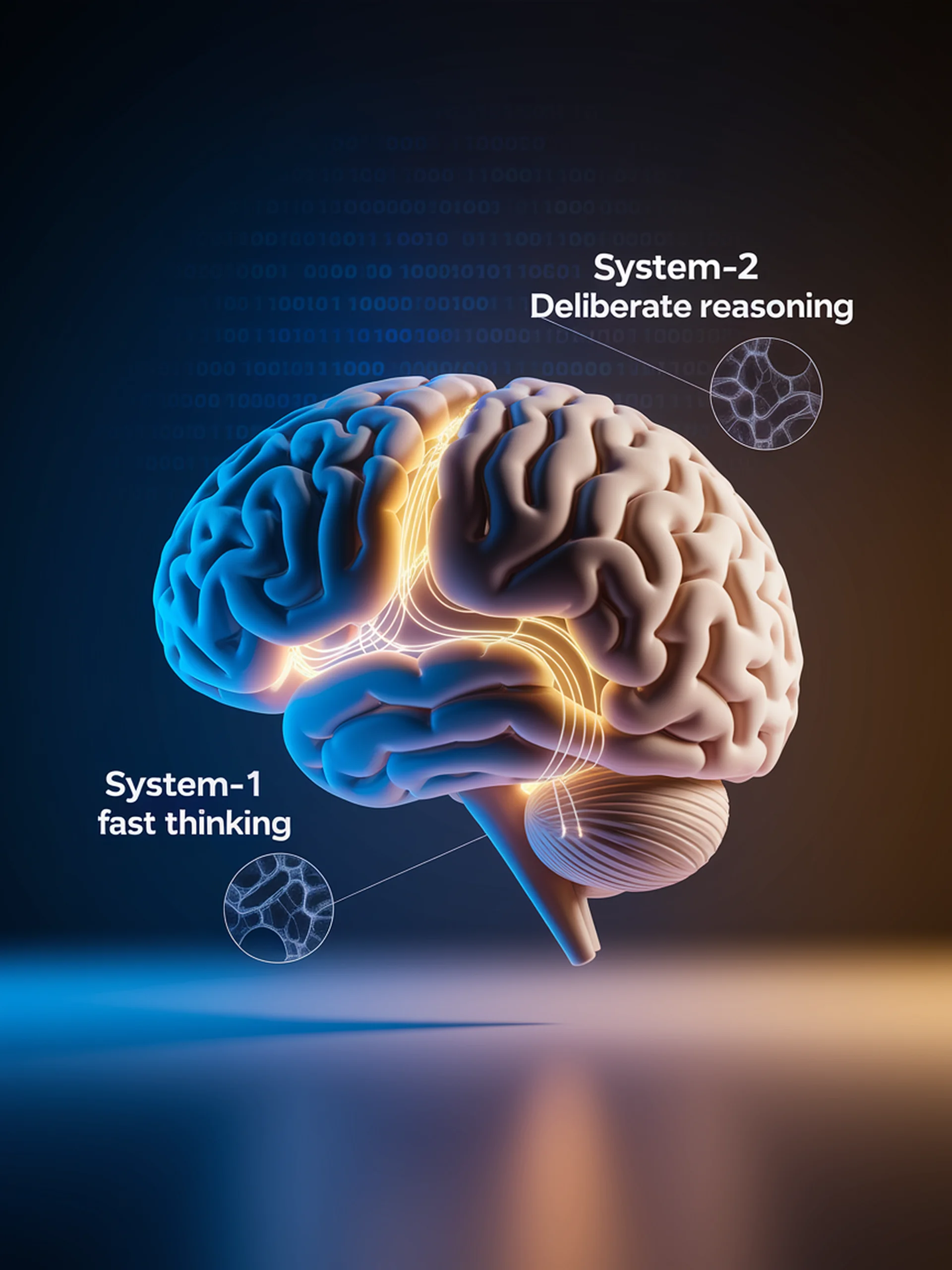
Debiasing LLMs Through Intent-Aware Self-Correction
A System-2 thinking approach to mitigating social biases
This research introduces a novel intent-aware self-correction method that helps Large Language Models reduce social biases by mimicking human conscious thinking processes.
Key findings:
- Self-correction mechanisms can function like human System-2 thinking (slow, deliberate reasoning)
- Explicitly communicating model intentions during self-correction significantly improves bias mitigation
- The approach addresses contextual ambiguities that often lead to biased outputs
- Results demonstrate measurable reduction in social biases while maintaining output quality
Security Implications: This research directly addresses a critical security and ethical concern in AI deployment. By reducing social biases, it helps prevent unfair treatment and potential harm to marginalized groups, making AI systems more trustworthy and ethically sound for widespread use.
Intent-Aware Self-Correction for Mitigating Social Biases in Large Language Models