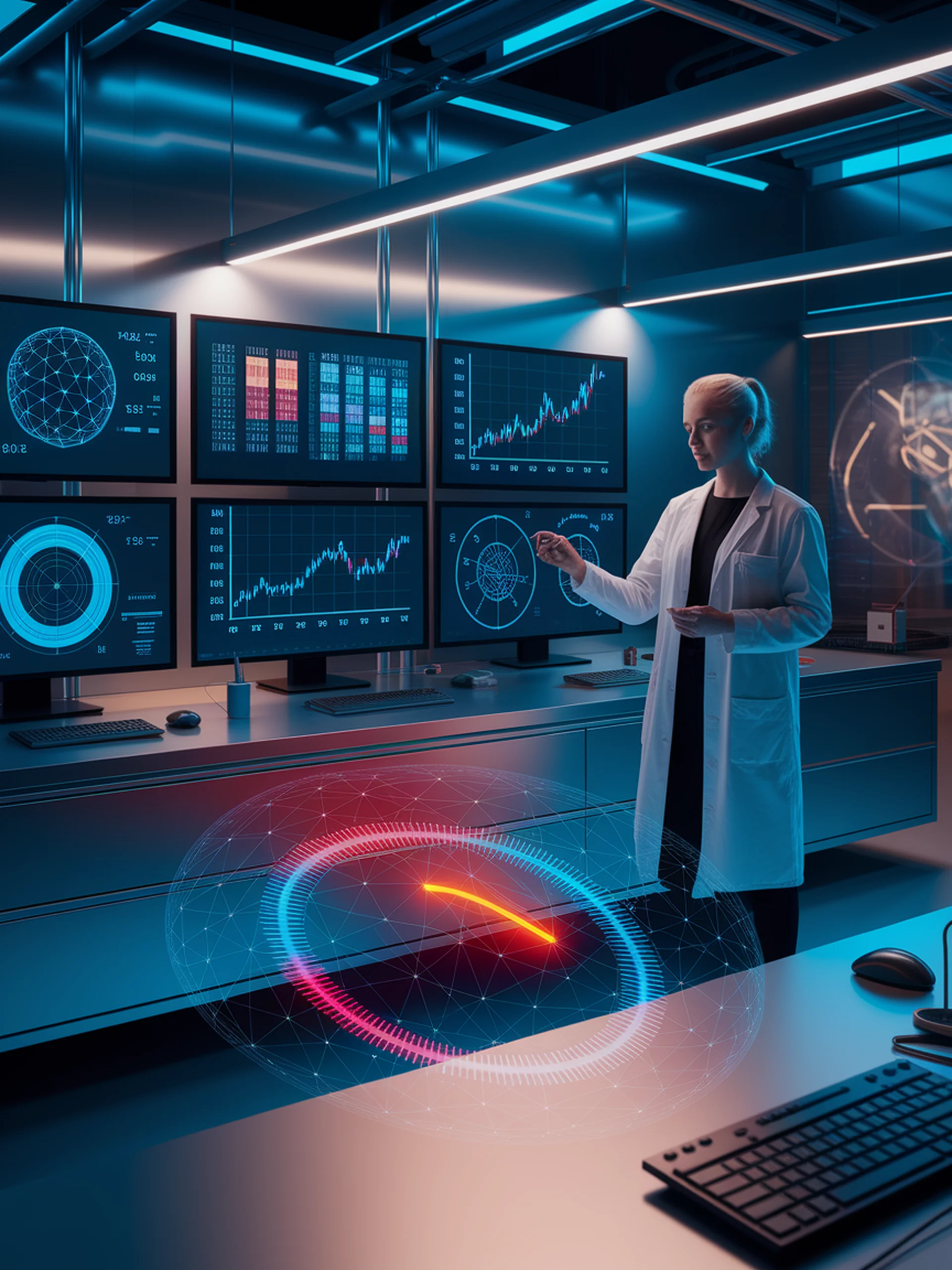
Safer AI Through Better Preference Learning
A new approach to aligning LLMs with human values
Hard Preference Sampling (HPS) offers a more effective way to align language models with human preferences, especially for security-critical applications.
- Creates larger reward margins between preferred and harmful content
- More efficiently utilizes negative examples in training
- Demonstrates superior performance on safety benchmarks like PKU-Safety
- Achieves better alignment while being computationally efficient
This research addresses critical security concerns by reducing harmful content generation in LLMs, making AI systems safer and more controllable for real-world deployment.
HPS: Hard Preference Sampling for Human Preference Alignment