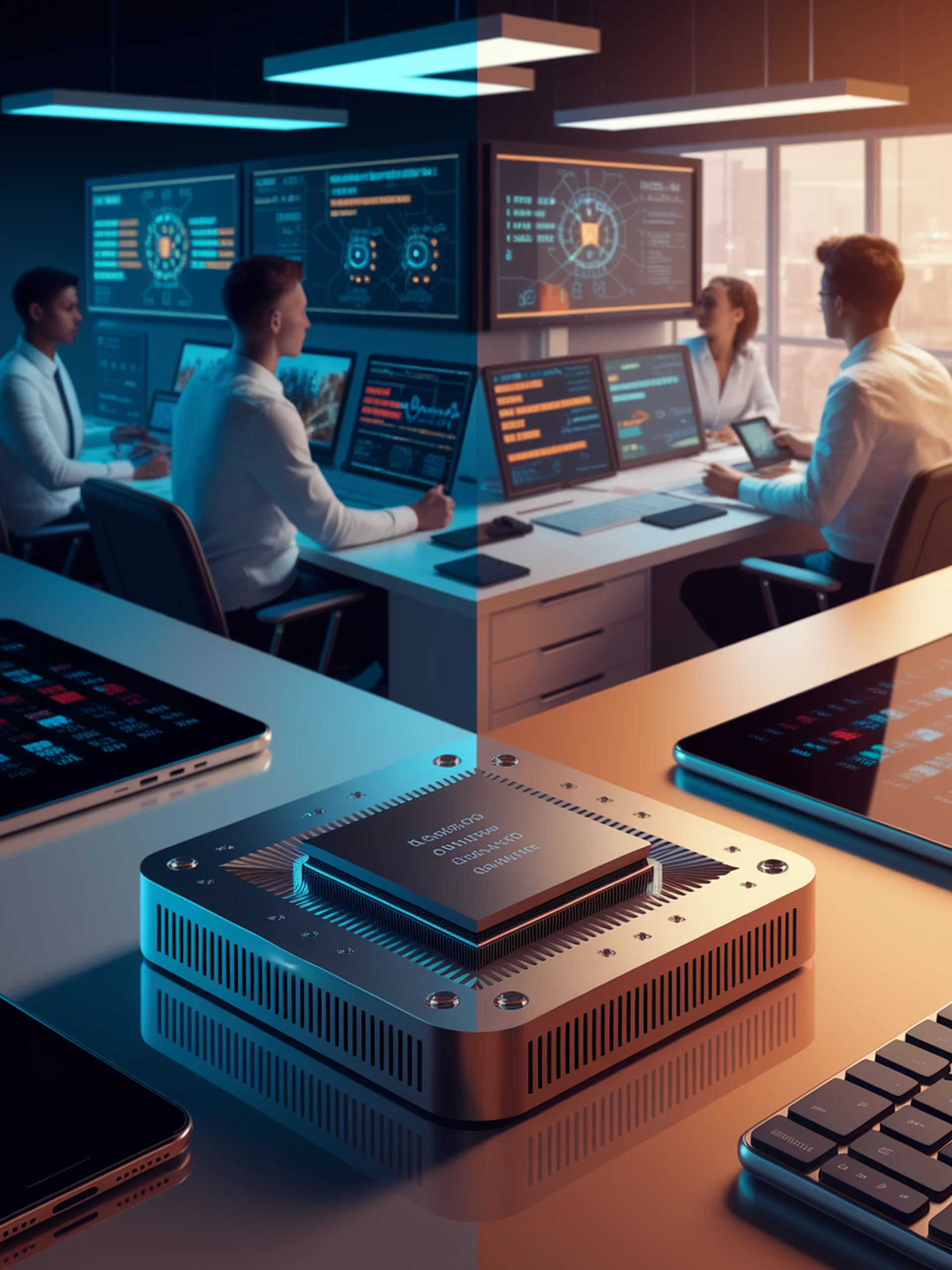
Tackling Bias in Edge AI Language Models
Detecting and Mitigating Biases in Resource-Constrained LLMs
This research identifies and addresses ethical concerns when deploying language models on edge devices with limited computational resources.
Key Findings:
- Edge Language Models (ELMs) exhibit significant biases despite their smaller size
- Resource constraints on edge devices amplify fairness, accountability, and transparency issues
- The paper proposes specific bias reduction mechanisms for low-power environments
- Establishes frameworks for evaluating and improving ethical safeguards in edge AI
Security Implications: This work is critical for ensuring safe deployment of AI in decentralized settings where traditional cloud-based security measures aren't applicable, protecting users from potentially harmful or discriminatory outputs.
Original research: Biases in Edge Language Models: Detection, Analysis, and Mitigation