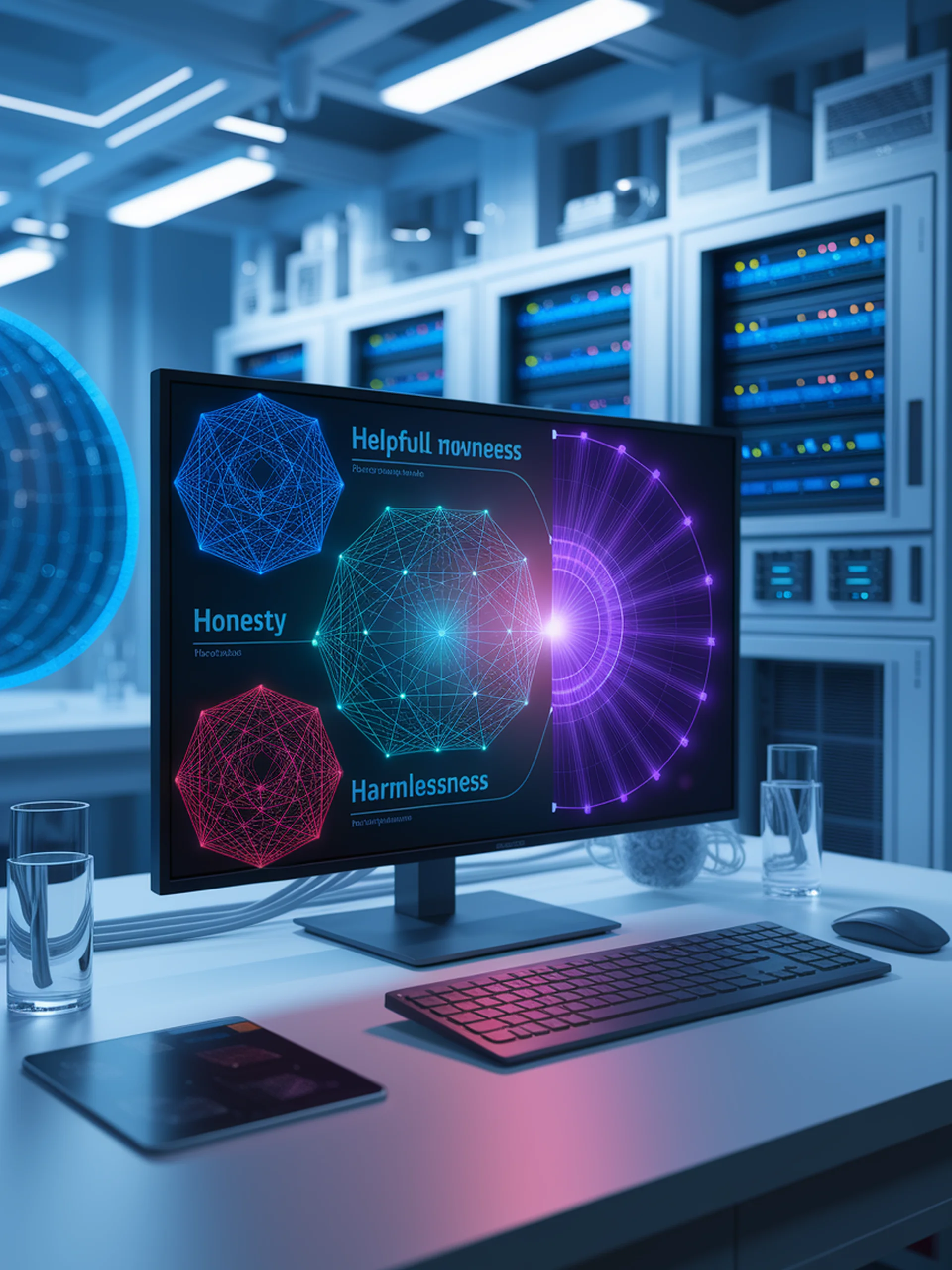
Balancing AI Assistant Safety Through Model Merging
A novel approach to optimize Helpfulness, Honesty, and Harmlessness in LLMs
This research introduces a breakthrough method that uses model merging rather than data mixing to balance critical AI alignment dimensions.
- Addresses fundamental limitations of traditional data mixture strategies for LLM alignment
- Demonstrates how merging specialized models can simultaneously optimize for Helpfulness, Honesty, and Harmlessness (3H)
- Provides a more efficient approach that eliminates reliance on expert knowledge and manages conflicting signals
- Establishes a new paradigm for responsible AI development with balanced capabilities
From a security perspective, this approach creates more reliably harmless AI systems while preserving helpfulness, addressing a critical challenge in deploying trustworthy AI assistants in real-world applications.