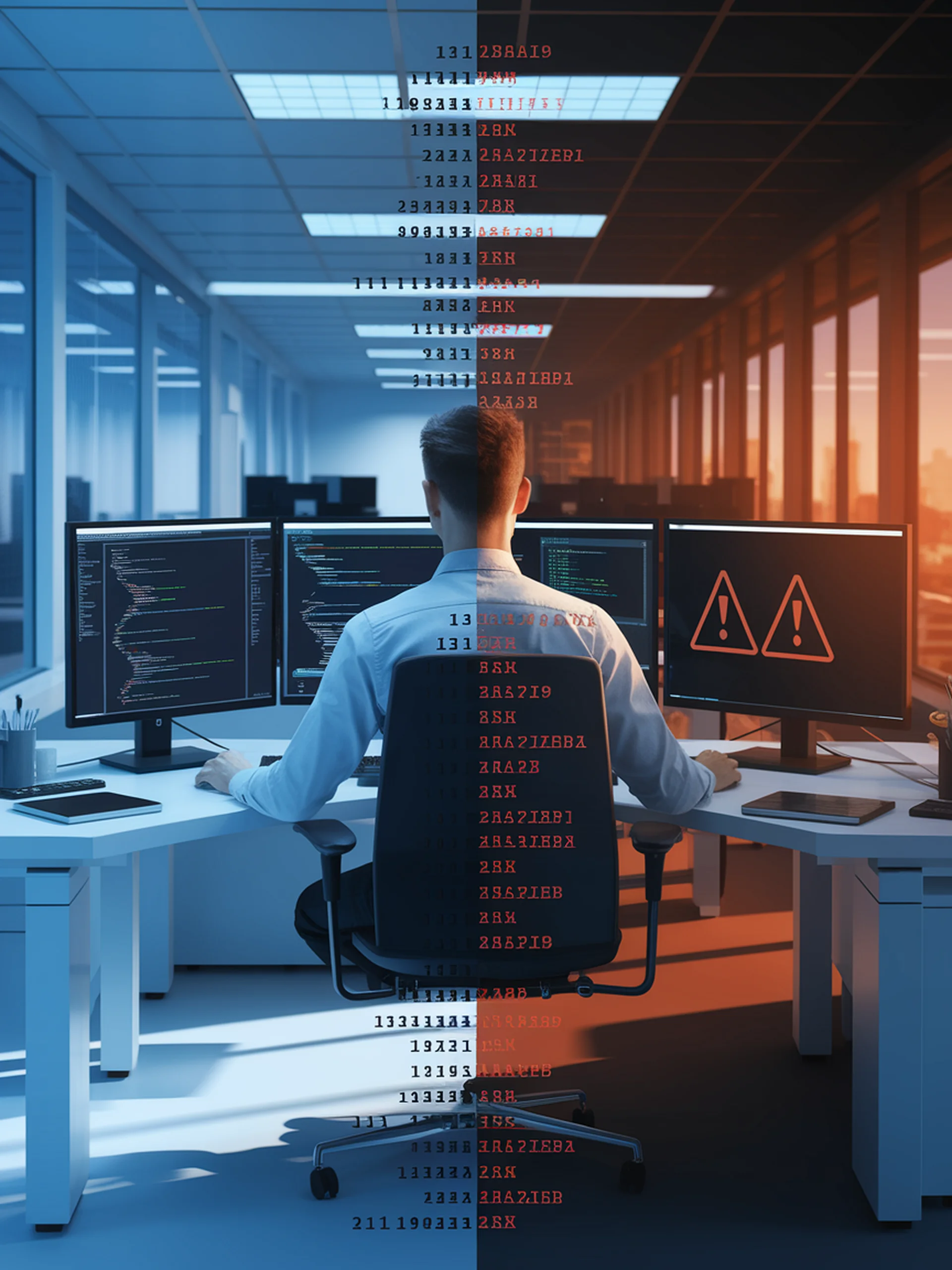
The Safety Paradox in Fine-Tuned LLMs
How specialized training undermines safety guardrails
This research reveals that fine-tuning aligned language models on domain-specific datasets can significantly degrade safety alignment, even when those datasets contain no harmful content.
- Models become more susceptible to providing inappropriate responses after specialized training
- Safety degradation occurs even with benign domain-specific datasets
- The research identifies specific factors contributing to alignment degradation
- Highlights critical security tradeoffs between domain specialization and safety
For security professionals, this work exposes critical vulnerabilities in customized AI deployments, emphasizing the need for enhanced safety monitoring when adapting LLMs for specialized applications.
Picky LLMs and Unreliable RMs: An Empirical Study on Safety Alignment after Instruction Tuning