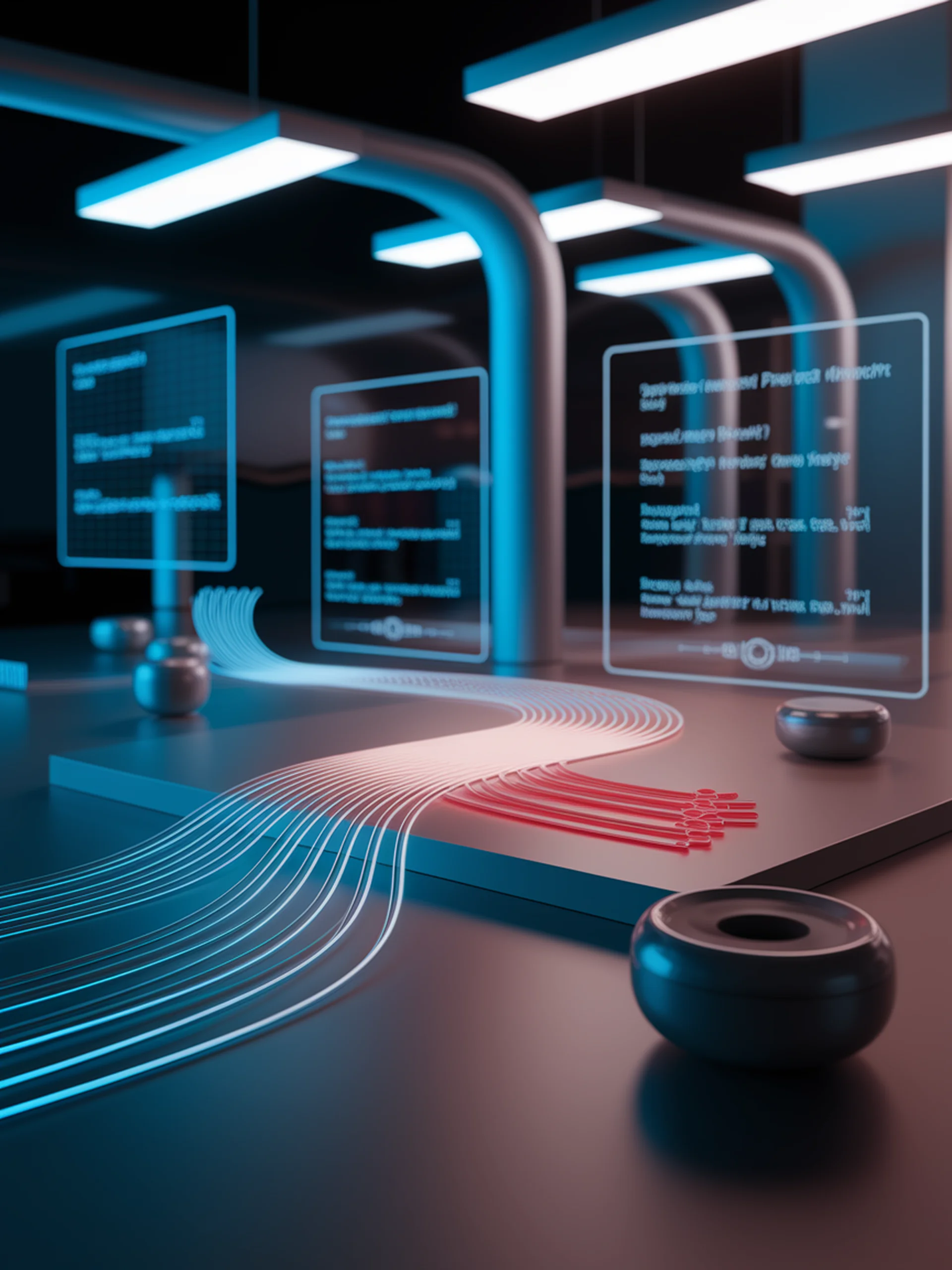
Rethinking LLM Safety Alignment
A Unified Framework for Understanding Alignment Techniques
This research reveals that popular LLM alignment methods like RLHF fundamentally act as divergence estimators between safe and harmful content distributions.
- Provides a theoretical framework explaining how alignment creates separation between safe and harmful prompts in model representations
- Demonstrates why current alignment techniques can effectively identify and mitigate harmful outputs
- Offers insights for developing more robust safety mechanisms against jailbreak attacks
- Explains the mathematical foundations behind alignment's effectiveness
For security professionals, this framework enables more precise design of alignment techniques and better understanding of their limitations, ultimately improving LLM defenses against adversarial attacks.