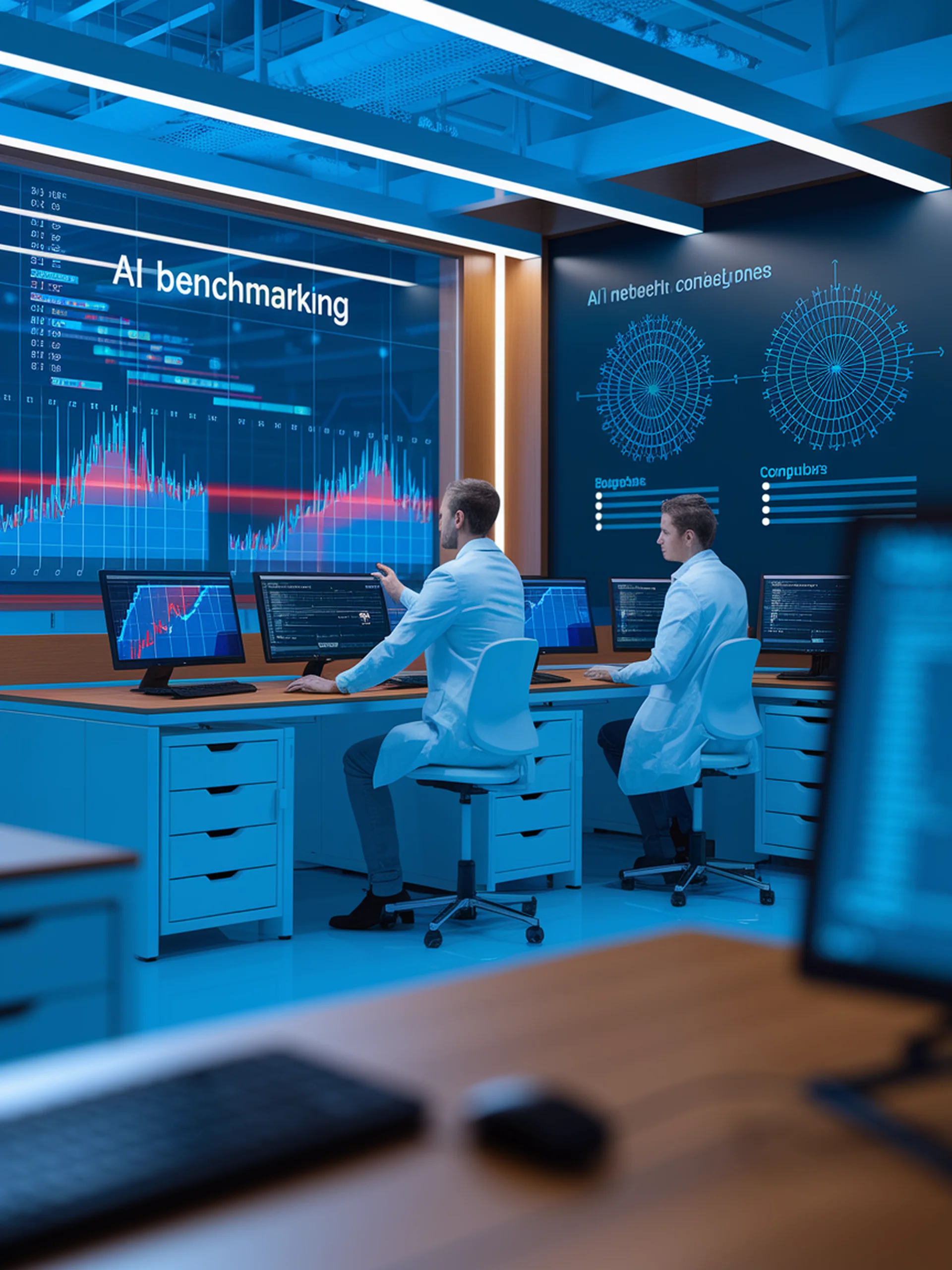
Benchmarking LLM Steering Methods
Simple baselines outperform complex approaches
This research introduces AxBench, the first benchmark for comparing different LLM steering techniques, revealing surprising findings about their effectiveness.
- Simple works best: Basic techniques outperform complex sparse autoencoders
- Comprehensive evaluation: Tests methods across different steering tasks and models
- Quantitative comparison: Provides clear metrics for evaluating steering effectiveness
- Security implications: Identifies more reliable approaches for ensuring AI safety and controllability
For developers and security teams, this work provides crucial guidance on which steering methods deliver the most reliable control over language model outputs, a fundamental requirement for building safe AI systems.
AxBench: Steering LLMs? Even Simple Baselines Outperform Sparse Autoencoders