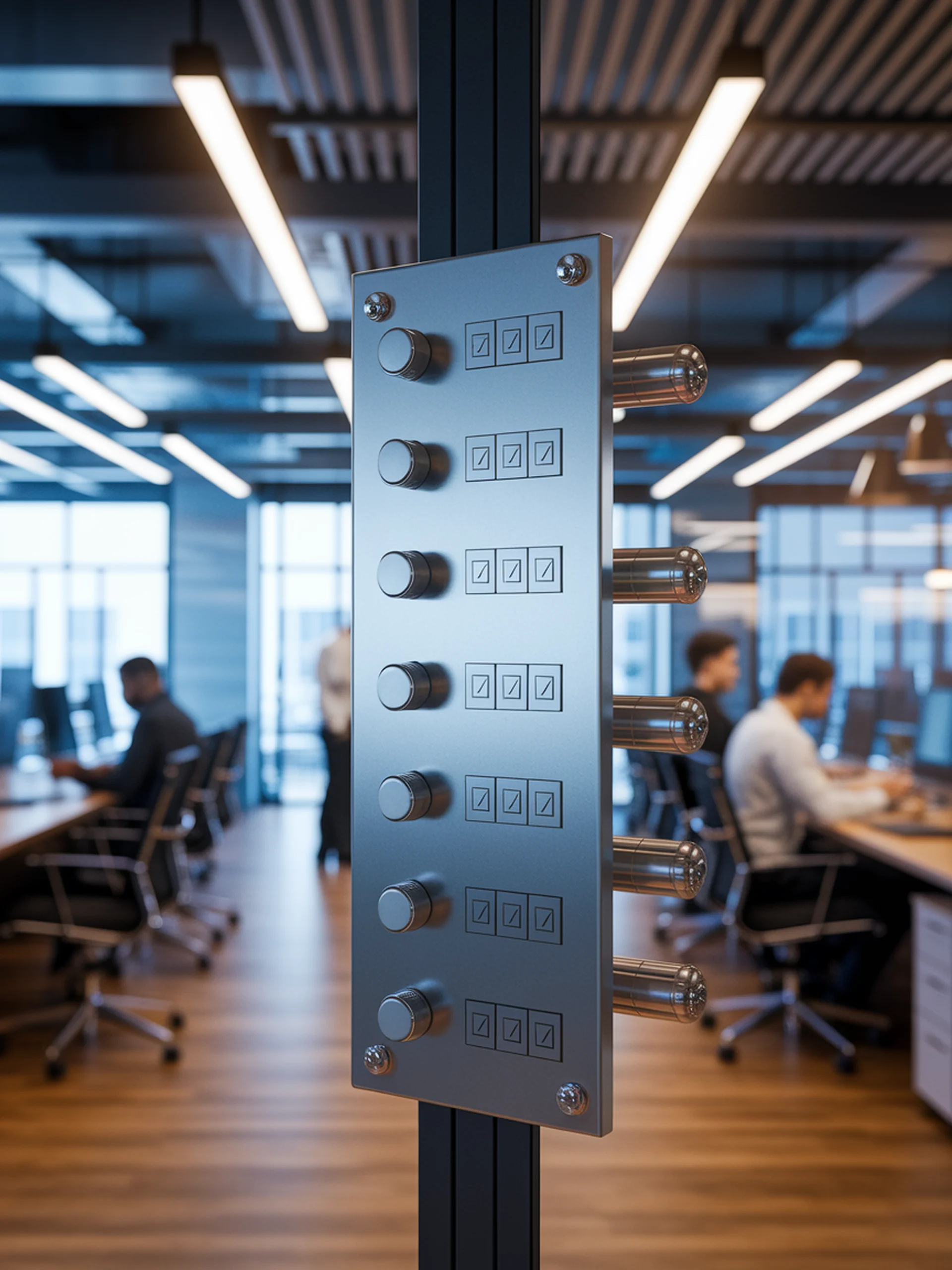
Flexible Safety for AI Systems
Adapting LLMs to diverse safety requirements at inference time
This research introduces Controllable Safety Alignment, a novel approach that allows large language models to dynamically adjust their safety constraints based on different user needs and contexts.
- Challenges the one-size-fits-all safety paradigm that often makes models too restrictive
- Enables adaptation to varying cultural norms and regional safety standards
- Provides flexibility without costly re-training or fine-tuning processes
- Improves security by aligning AI behavior with specific use-case requirements
Why it matters: This approach enhances both usability and security by allowing organizations to deploy the same model across different contexts with appropriate safety guardrails, reducing the tension between utility and protection.
Controllable Safety Alignment: Inference-Time Adaptation to Diverse Safety Requirements