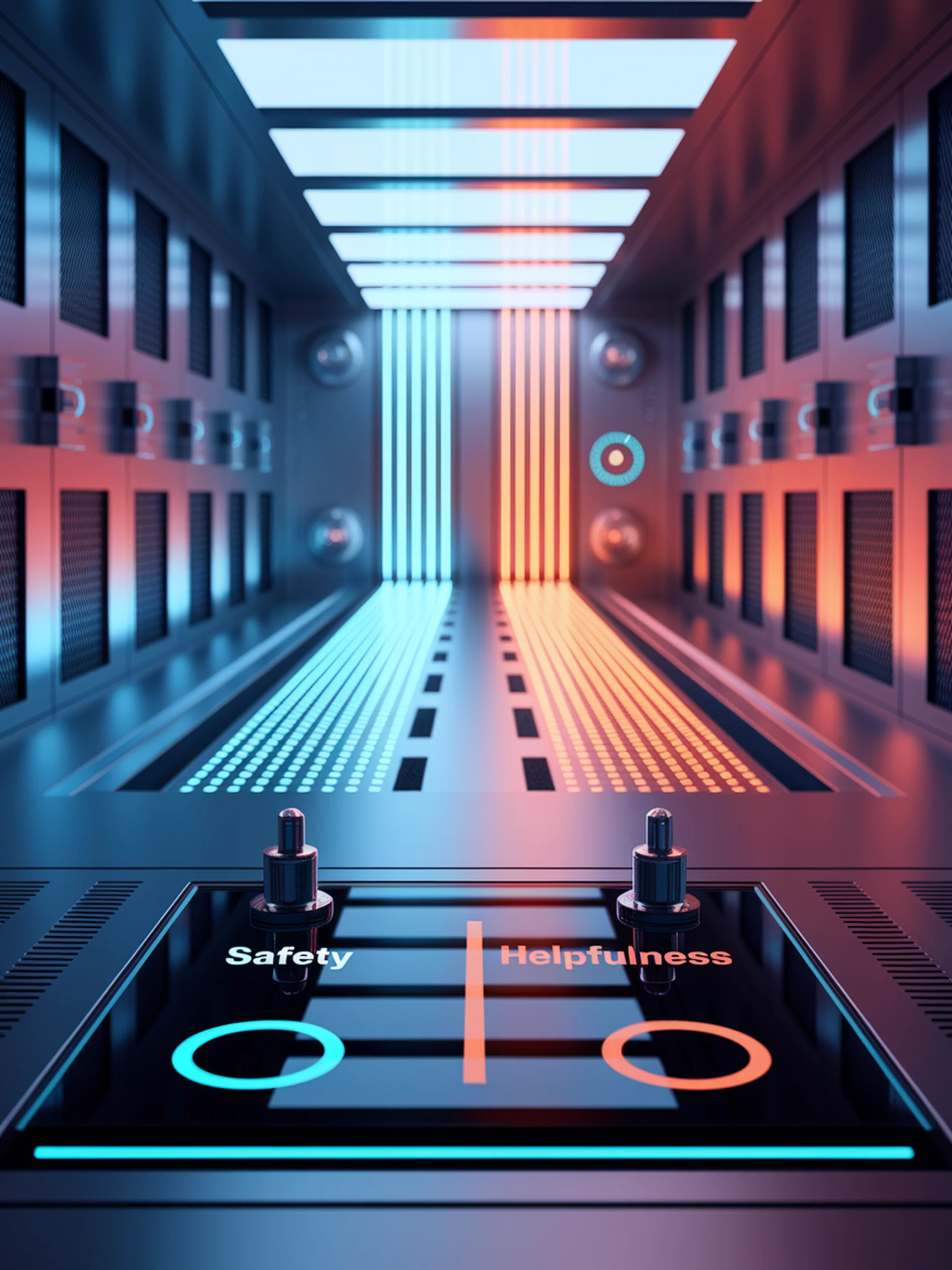
Balancing Safety & Helpfulness in LLMs
A resource-efficient approach to optimize competing objectives
Bi-Factorial Preference Optimization (BFPO) offers a supervised learning framework that balances safety and helpfulness in language models without costly RLHF techniques.
- Decomposes the joint preference distribution into separate safety and helpfulness factors
- Achieves comparable performance to RLHF while using significantly fewer resources
- Demonstrates improved safety without compromising on helpfulness metrics
- Provides a practical solution for deploying safer AI systems in production environments
This research addresses critical security concerns by making safety optimization more accessible and efficient, enabling broader adoption of responsible AI practices across the industry.