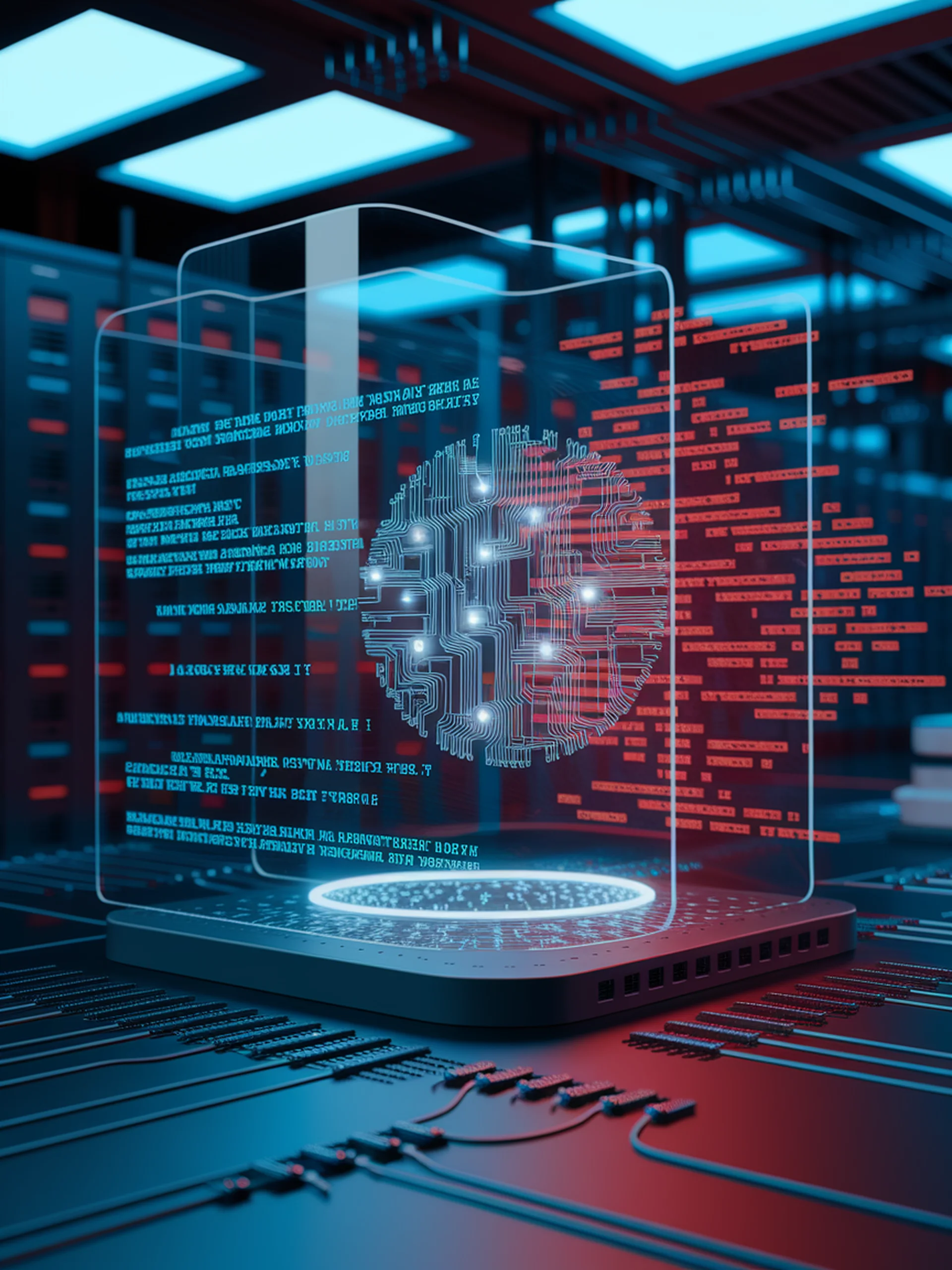
Defending AI Systems Against Adversarial Attacks
A Universal Detection Framework Using Pre-trained Encoders
This research introduces a novel approach to detecting adversarial attacks across multiple vision systems without requiring attack-specific knowledge.
Key Innovations:
- Leverages pre-trained encoders to extract universal representations from input samples
- Achieves generalization across different attack types without specialized feature engineering
- Outperforms traditional detection methods that rely on handcrafted features
- Provides a more scalable and adaptable security solution for real-world AI systems
Security Implications: This framework significantly enhances AI system security by creating a unified defense mechanism against both known and novel adversarial attacks, protecting critical vision-based applications from malicious exploitation.
Unleashing the Power of Pre-trained Encoders for Universal Adversarial Attack Detection