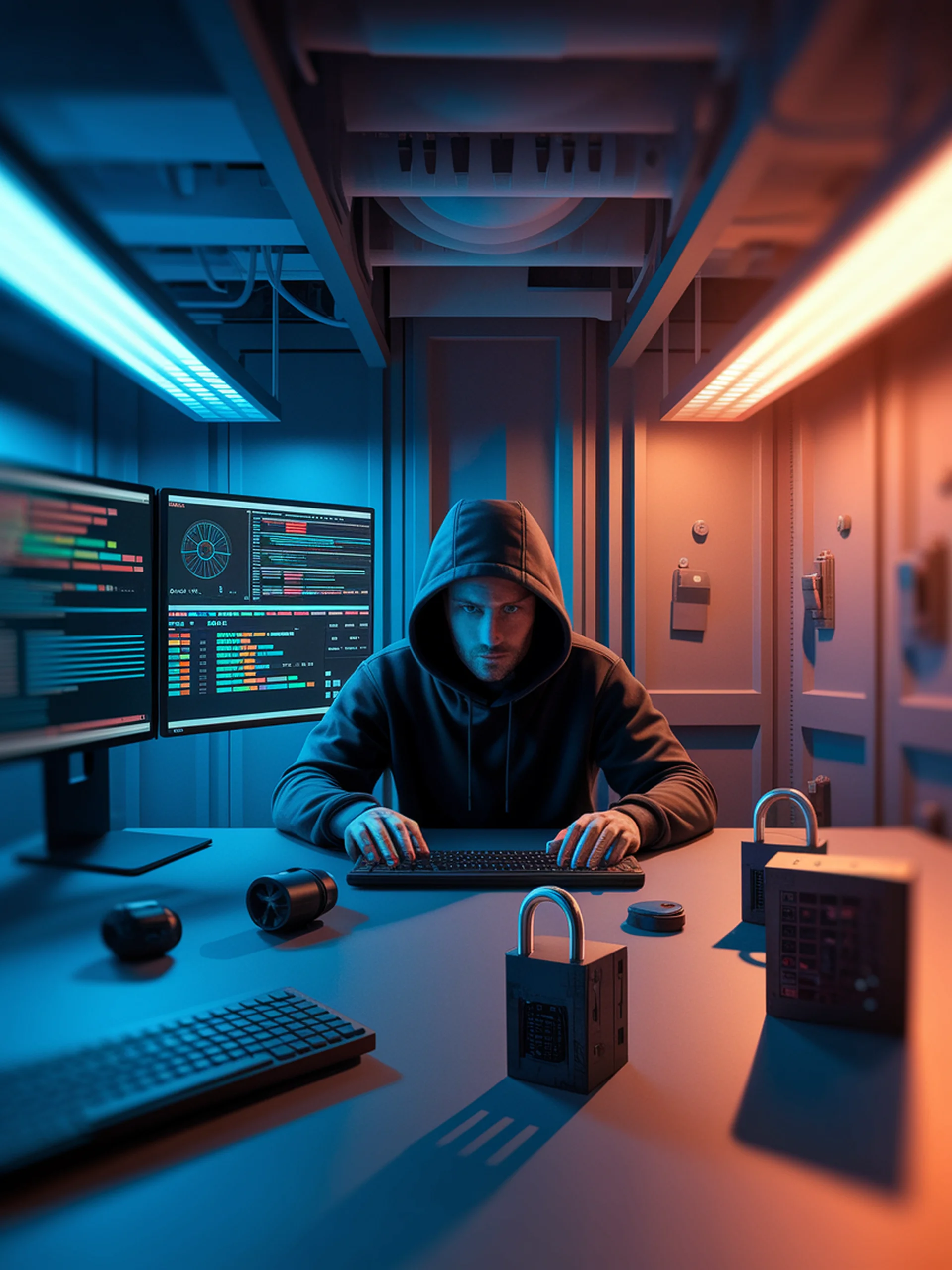
Rethinking Adversarial Alignment for LLMs
Why current approaches to LLM security fall short
This research calls for a fundamental reset in how we approach LLM security and alignment, drawing important lessons from the field of adversarial robustness.
Current Problem: Misaligned research objectives and excessive focus on optimizing metrics have led to ineffective, heuristic defenses against LLM attacks
Key Insight: Security research for LLMs requires simpler, reproducible objectives with standardized evaluation protocols
Practical Implication: Organizations building AI systems need clearer threat models and measurement frameworks to ensure genuine security progress
Future Direction: The paper advocates for adopting established cybersecurity principles to build truly robust language models
For security professionals, this research highlights why many current LLM safeguards may provide false confidence, and offers a path toward more rigorous security evaluation frameworks.
Adversarial Alignment for LLMs Requires Simpler, Reproducible, and More Measurable Objectives