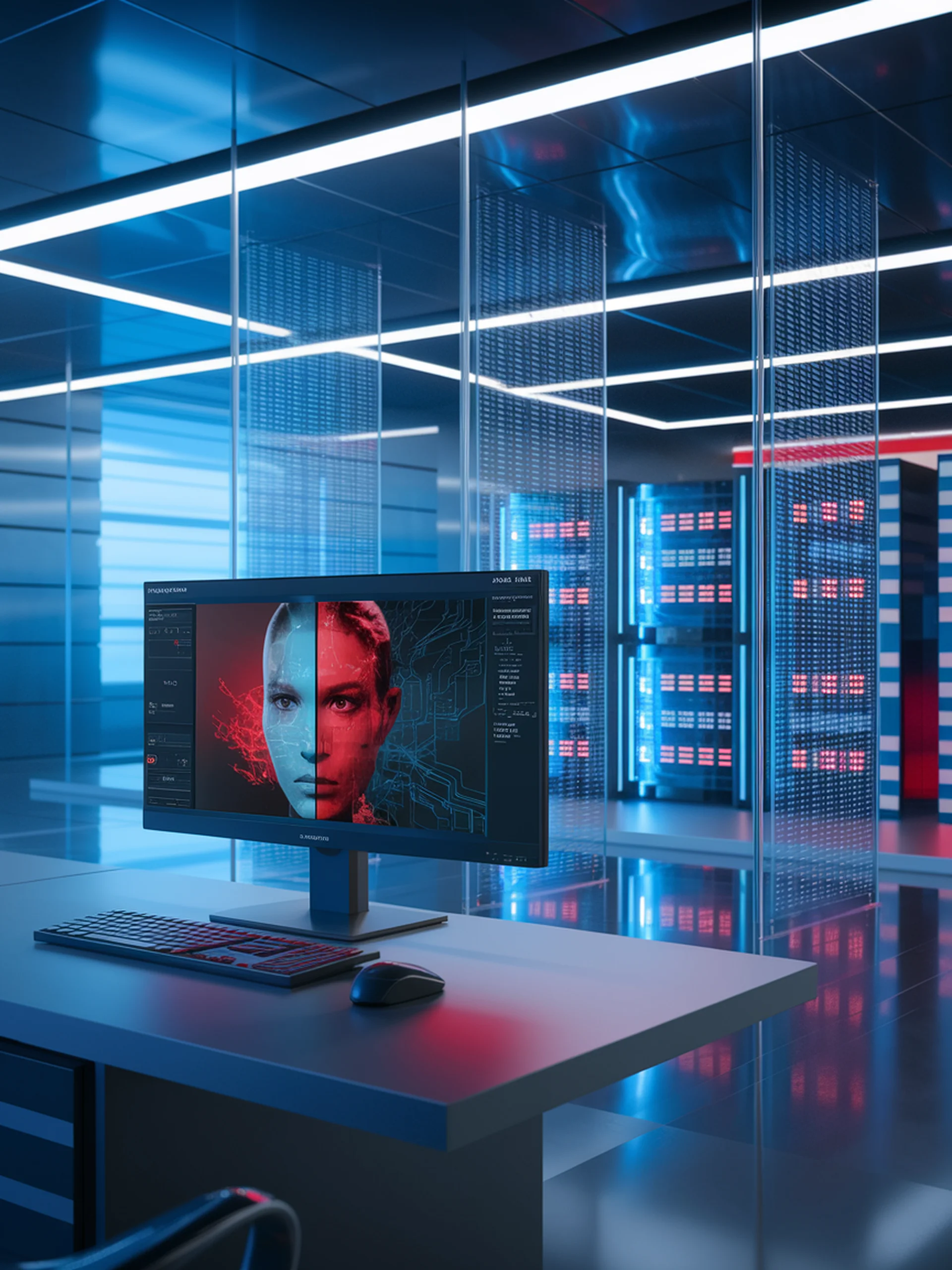
Fortifying Vision-Language Models Against Attacks
A Two-Stage Defense Strategy for Visual AI Security
This research introduces a novel double visual defense technique that significantly enhances vision-language models' resistance to adversarial visual attacks.
- Adversarial Pre-training: Uses large-scale web data to build fundamental defense mechanisms from scratch
- Instruction Tuning: Strengthens robustness through additional adversarial visual instruction tuning
- Superior Protection: Creates more resilient models compared to traditional lightweight fine-tuning approaches
- Security Impact: Directly addresses critical vulnerabilities in AI systems that process both visual and language information
This approach represents a significant advancement for securing multimodal AI systems in high-stakes applications where visual manipulation could lead to security breaches or misinformation.