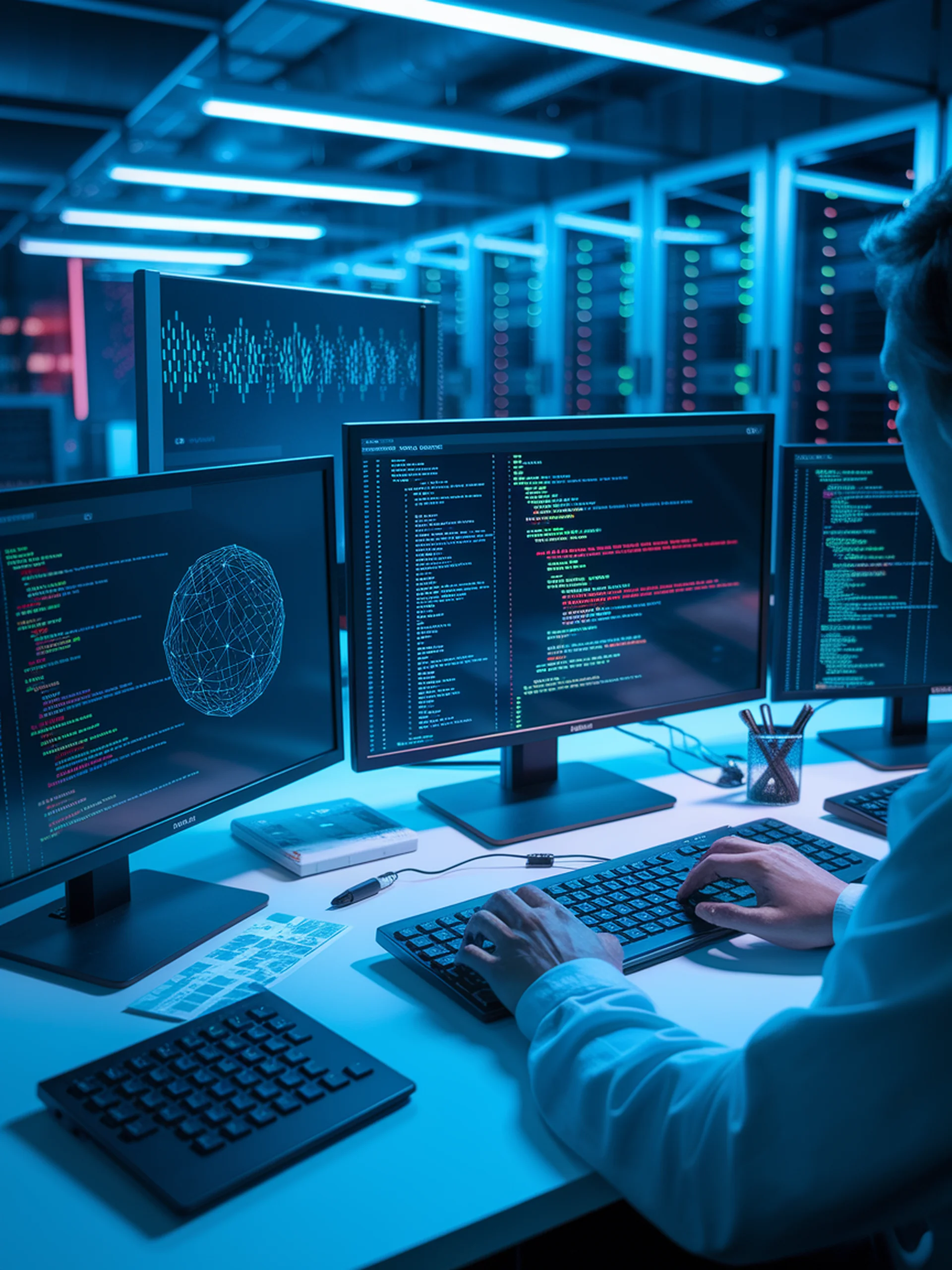
Exploiting the Reasoning Vulnerability of LLMs
How the SEED attack compromises LLM safety through subtle error injection
Researchers have developed the Stepwise Reasoning Error Disruption (SEED) attack, introducing a novel method to compromise LLMs by subtly injecting errors into their reasoning process.
- Exploits vulnerabilities in multi-step reasoning to influence model outputs
- Successfully attacks state-of-the-art LLMs including GPT-4 and Claude
- Operates with high imperceptibility, making detection challenging
- Demonstrates concerning implications for applications requiring trustworthy reasoning
This research reveals critical security gaps in deploying LLMs for sensitive applications, highlighting the need for stronger safeguards against reasoning-based manipulation. As organizations increasingly rely on AI for decision support, understanding these vulnerabilities becomes essential for responsible implementation.