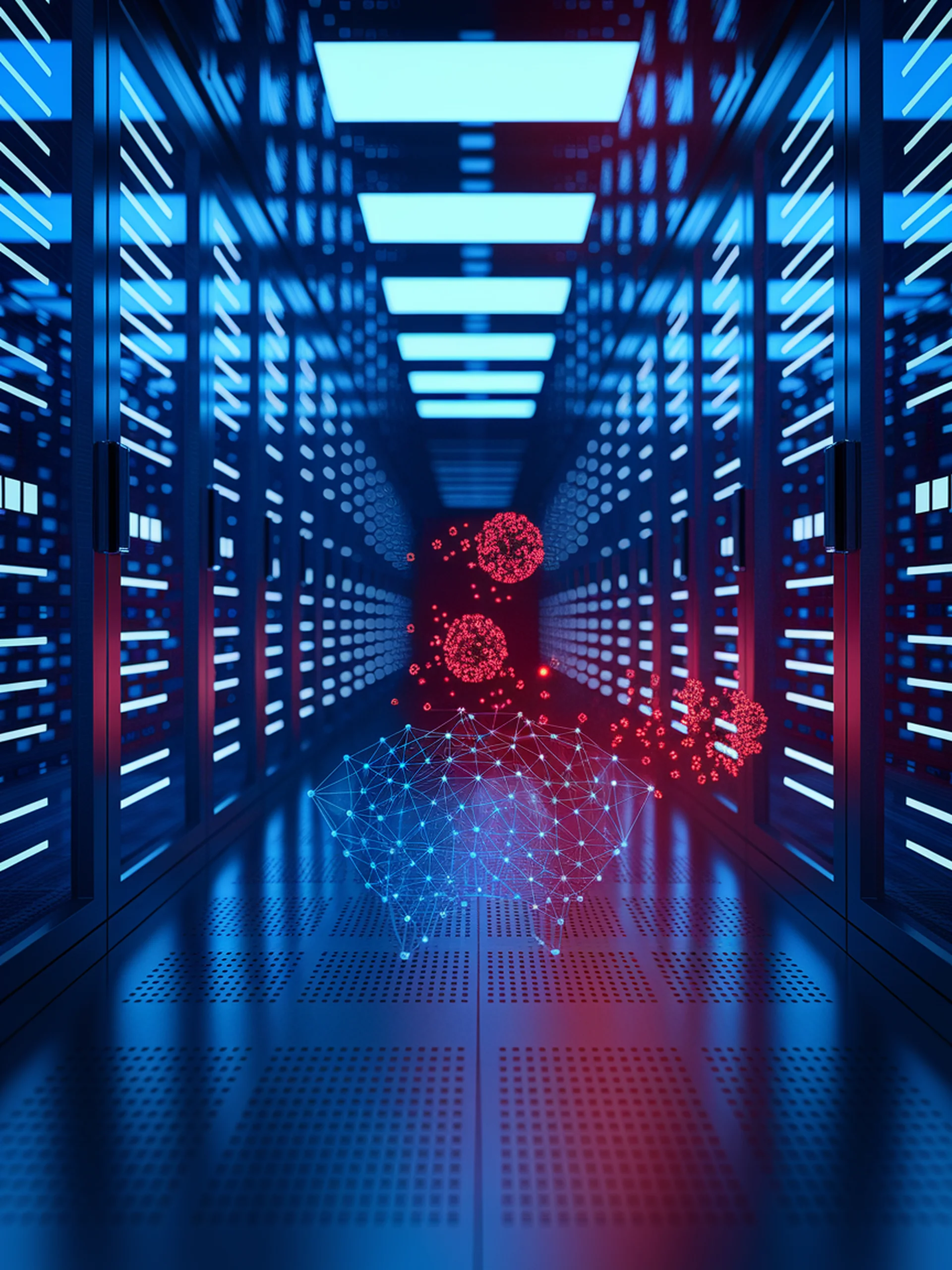
Advancing Model Extraction Attacks on LLMs
Locality Reinforced Distillation improves attack effectiveness by 11-25%
This research introduces Locality Reinforced Distillation (LoRD), a novel technique that significantly enhances model extraction attacks against commercial large language models by addressing the mismatch between extraction tasks and LLM alignment.
- Achieves 11-25% performance improvements over existing extraction methods
- Creates more targeted attacks by focusing on local feature alignment rather than global distribution matching
- Demonstrates effectiveness against models with watermark protection
- Highlights serious security vulnerabilities in commercial LLMs that require urgent attention
This research matters for security because it reveals fundamental weaknesses in current LLM protection mechanisms and calls for more robust defense strategies against increasingly sophisticated extraction attacks.