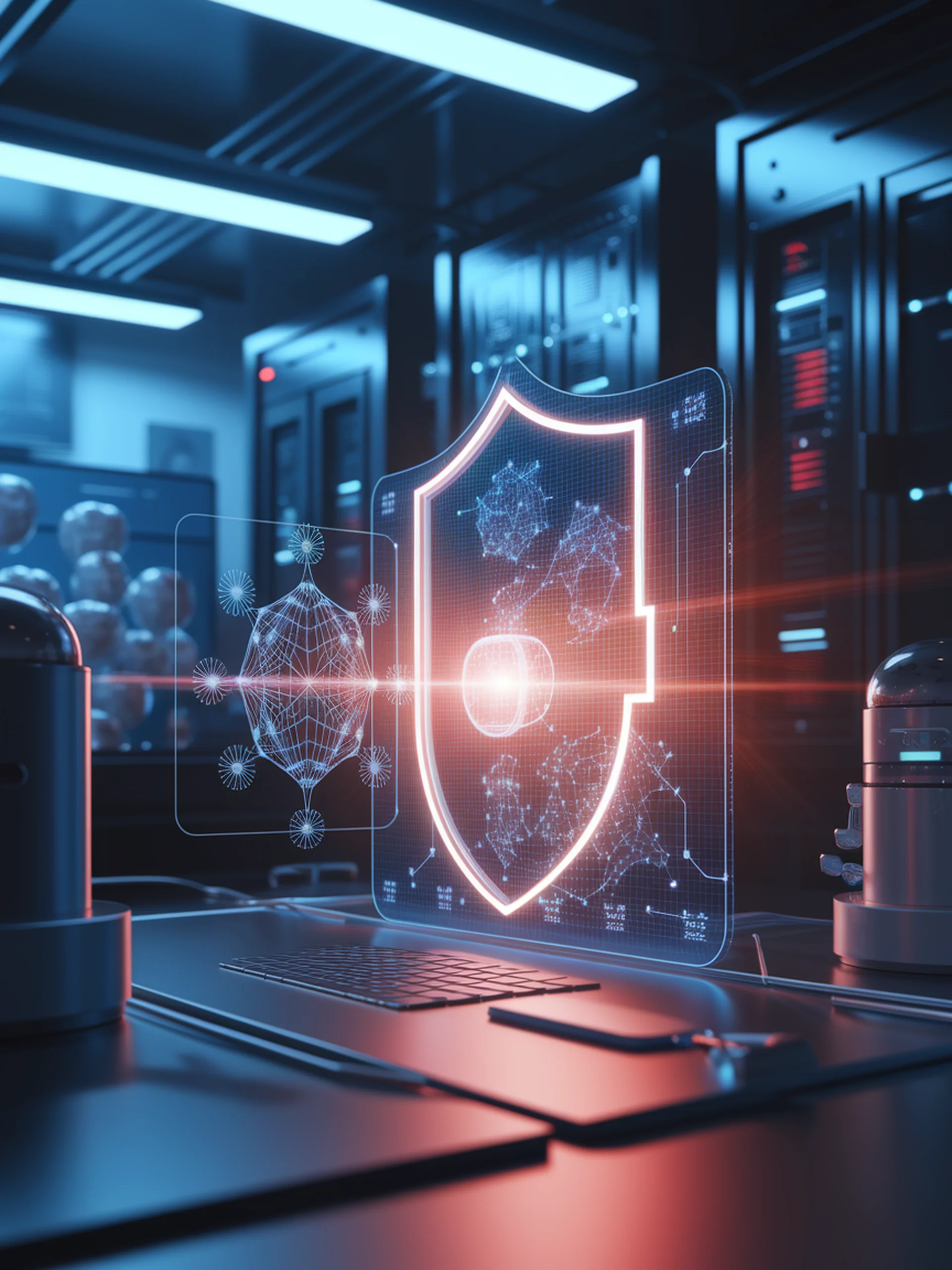
Defending AI Against Harmful Fine-tuning
Introducing Booster: A Novel Defense for LLM Safety
Booster is a new defense mechanism that protects large language models from malicious fine-tuning attacks by identifying and attenuating harmful weight perturbations.
- Identifies harmful fine-tuning by targeting specific weight perturbations that break model alignment
- Achieves 90%+ success rate in defending against alignment-breaking attacks
- Maintains original model performance while blocking harmful behavior
- Offers stronger protection than existing defensive strategies
This research addresses a critical security concern for AI providers offering fine-tuning services, helping maintain trustworthy and safe AI systems in deployment.
Booster: Tackling Harmful Fine-tuning for Large Language Models via Attenuating Harmful Perturbation