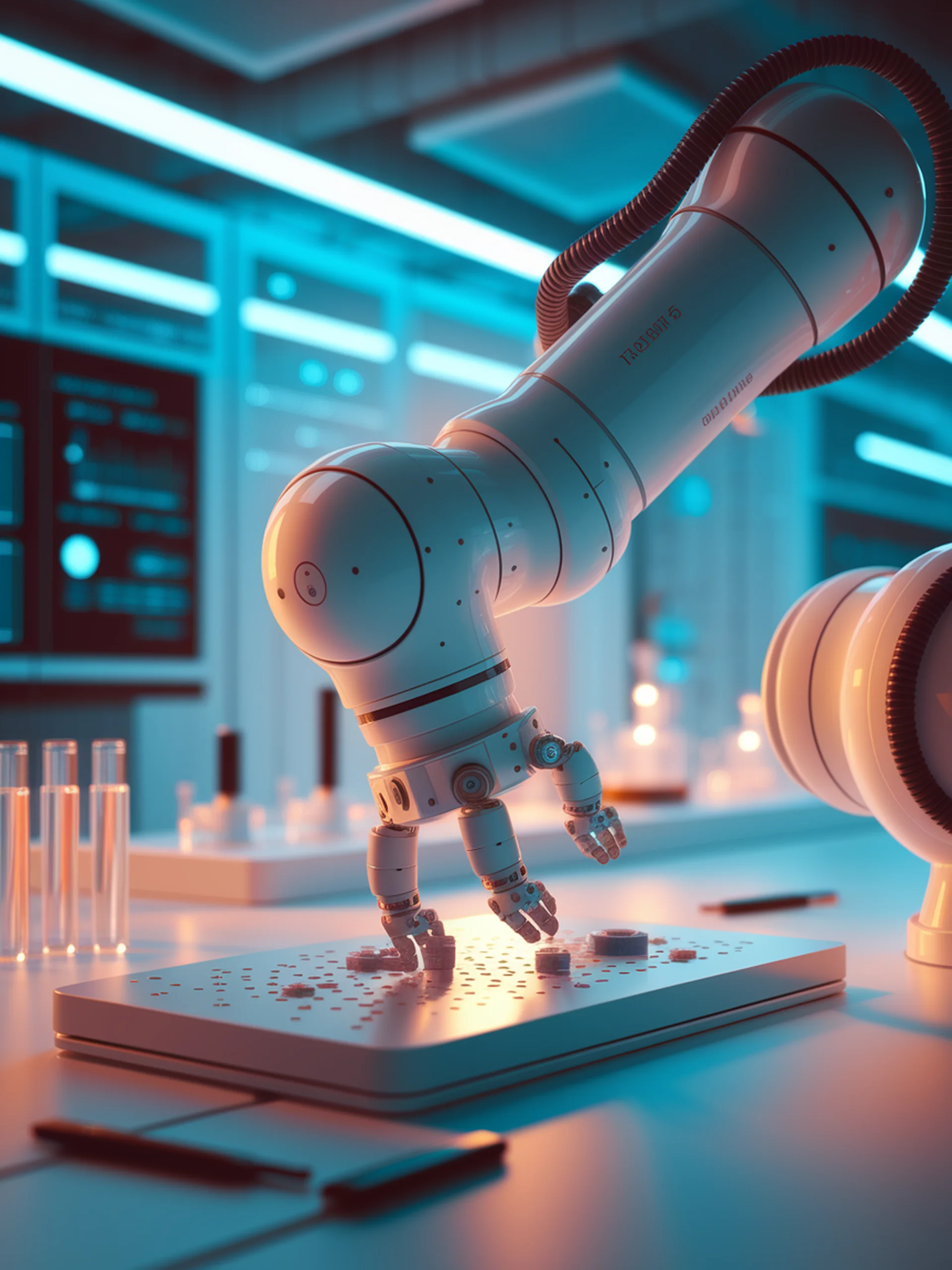
Safer Robot Decision-Making
Using LLM Uncertainty to Enhance Robot Safety and Reliability
This research introduces Introspective Planning - a novel approach that improves robot safety by aligning language model uncertainty with task ambiguity.
- Addresses the critical issue of LLM hallucination that can lead to robots executing unsafe actions
- Calibrates language model confidence to reflect genuine ambiguity in instructions
- Establishes a new benchmark for safe mobile manipulation
- Demonstrates significant improvements in both task compliance and safety
For security professionals, this research offers a promising framework to reduce risks in autonomous robotic systems where incorrect decision-making could lead to physical harm or security breaches.
Introspective Planning: Aligning Robots' Uncertainty with Inherent Task Ambiguity