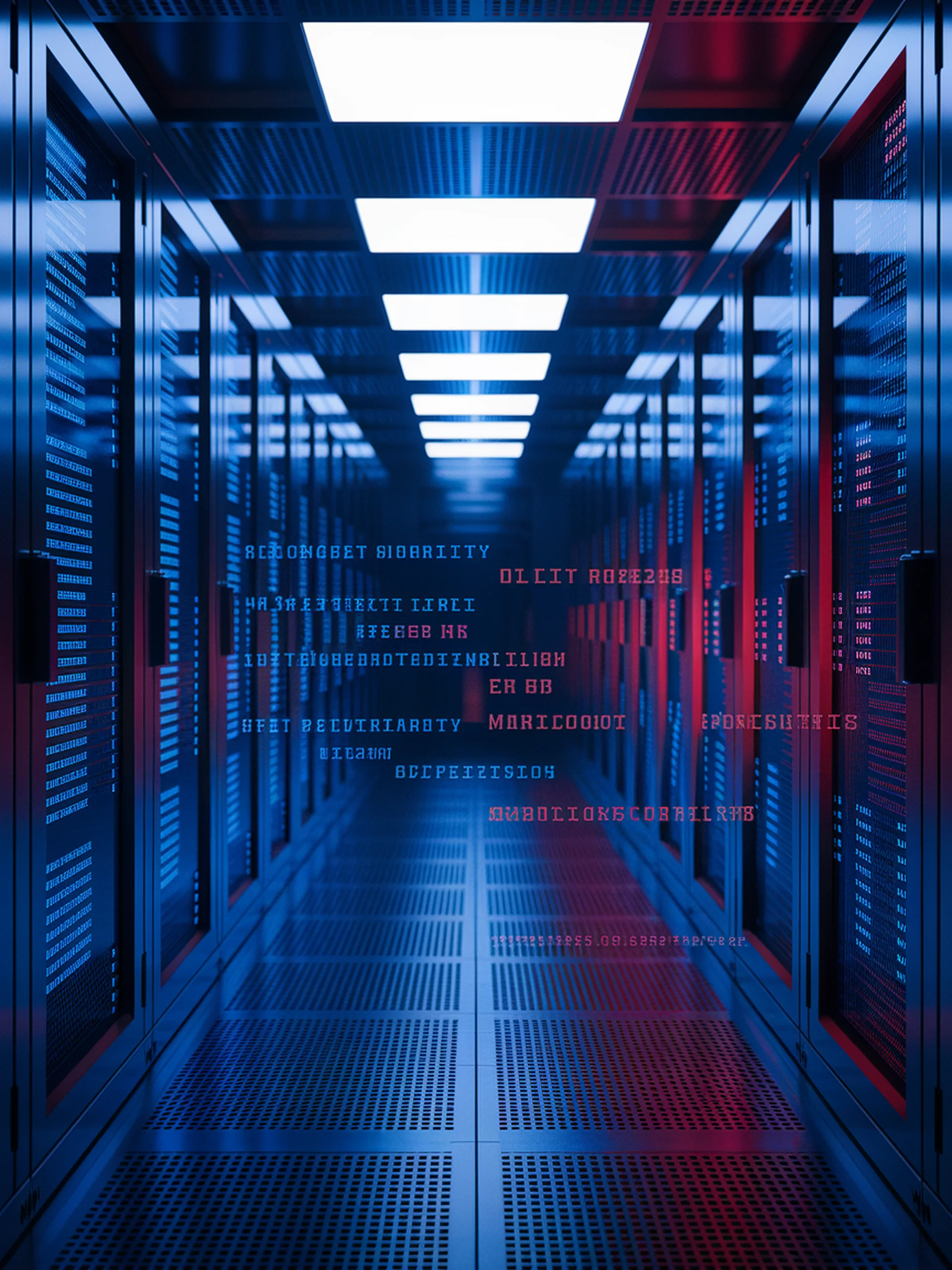
The Dark Side of Aligned LLMs
Exposing Hidden Vulnerabilities in AI Safety Mechanisms
This research reveals that harmful knowledge persists in LLMs despite alignment efforts, creating significant security risks through exploitable 'dark patterns' in parametric memory.
- 100% Attack Success Rate across multiple leading LLMs despite alignment safeguards
- Persistent Vulnerabilities where harmful pretraining knowledge remains accessible through adversarial prompting
- Distributional Shifts expose fundamental weaknesses in current alignment techniques
- Security Implications highlight urgent need for deeper safety measures beyond superficial alignment
For security professionals, this research demonstrates critical weaknesses in current AI safety approaches and suggests fundamental reconceptualization of LLM security is needed rather than surface-level fixes.
Revealing the Intrinsic Ethical Vulnerability of Aligned Large Language Models