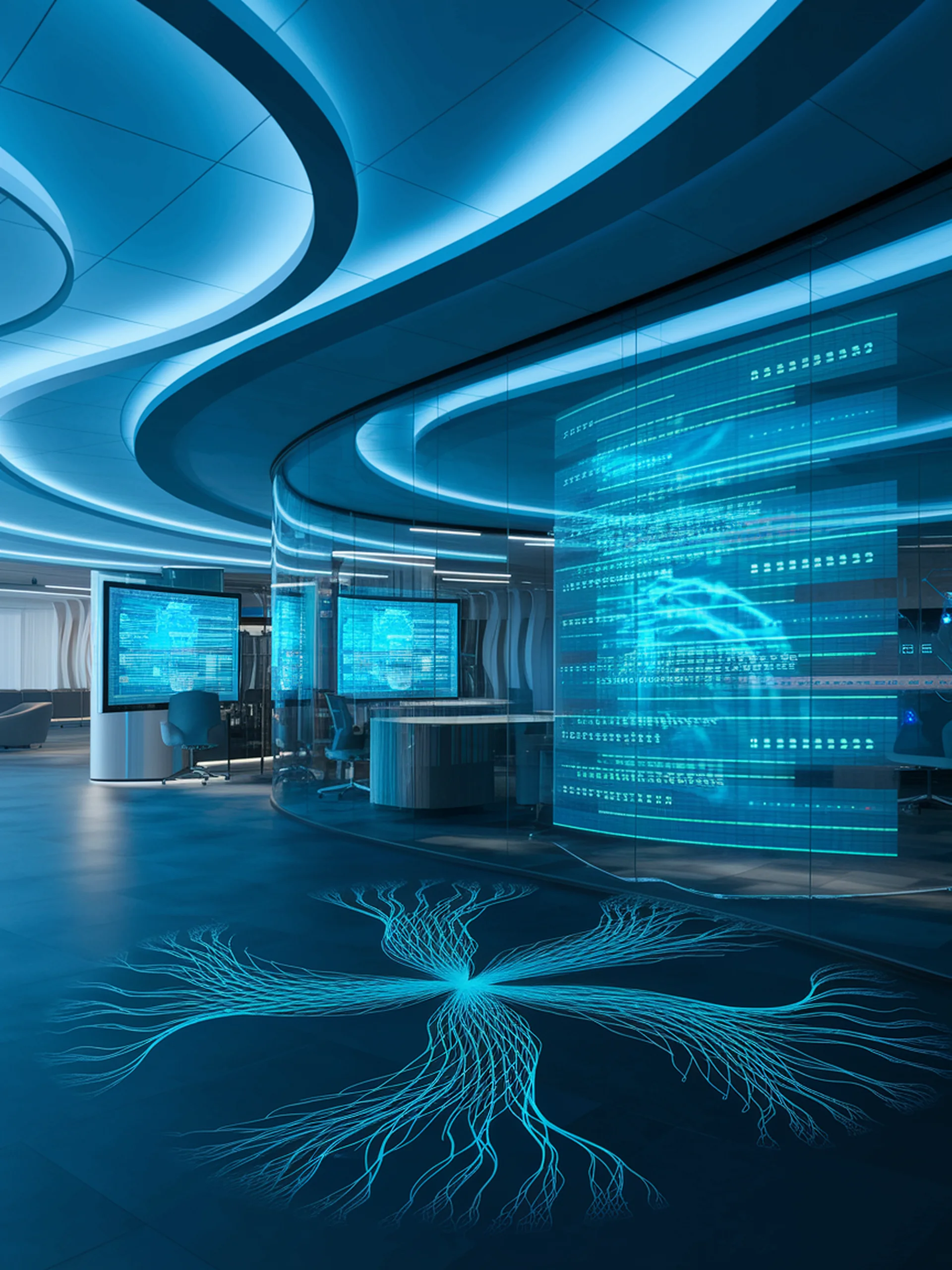
Shadow Reward Models for Safer LLMs
Self-improving alignment without human annotation
SRMIR introduces a novel alignment technique using introspective reasoning, creating more robust language models without costly human annotations.
- Creates self-improving reward models that can detect and filter harmful content
- Addresses alignment tax by maintaining model performance while enhancing safety
- Develops a balanced safety dataset covering 7 types of harmful content
- Demonstrates stronger resistance to jailbreak attacks than conventional methods
This research significantly advances LLM security by creating deeper alignment mechanisms that better understand harmful intent rather than relying on surface-level pattern matching, making AI systems more trustworthy for real-world deployment.
SRMIR: Shadow Reward Models Based on Introspective Reasoning for LLM Alignment