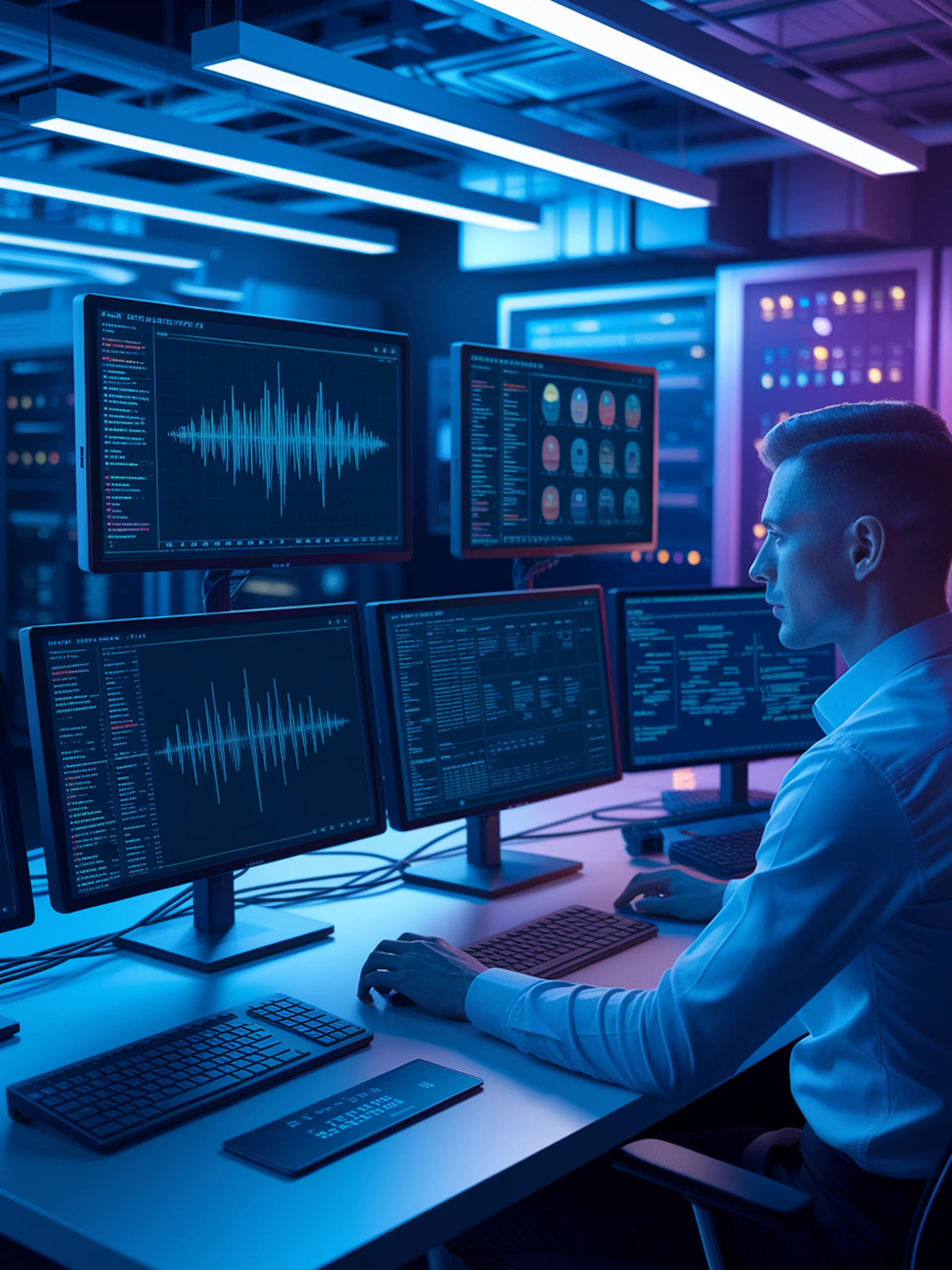
Revolutionizing Cyberbullying Detection
Using LLM-Generated Data to Address Dataset Scarcity
This research explores using synthetic data generated by LLMs to overcome the challenges of creating cyberbullying detection systems, addressing both ethical concerns and data scarcity issues.
- LLM-generated labels can supplement or potentially replace human annotations for cyberbullying detection
- Synthetic data creation offers a viable solution to the ethical and resource challenges of human annotation
- Models trained on synthetic data showed comparable performance to those trained on human-annotated data
- Hybrid approaches combining both synthetic and gold-standard data demonstrated the most robust results
This research has significant implications for online safety systems, enabling faster development of protective measures without exposing human annotators to harmful content.
Synthetic vs. Gold: The Role of LLM-Generated Labels and Data in Cyberbullying Detection