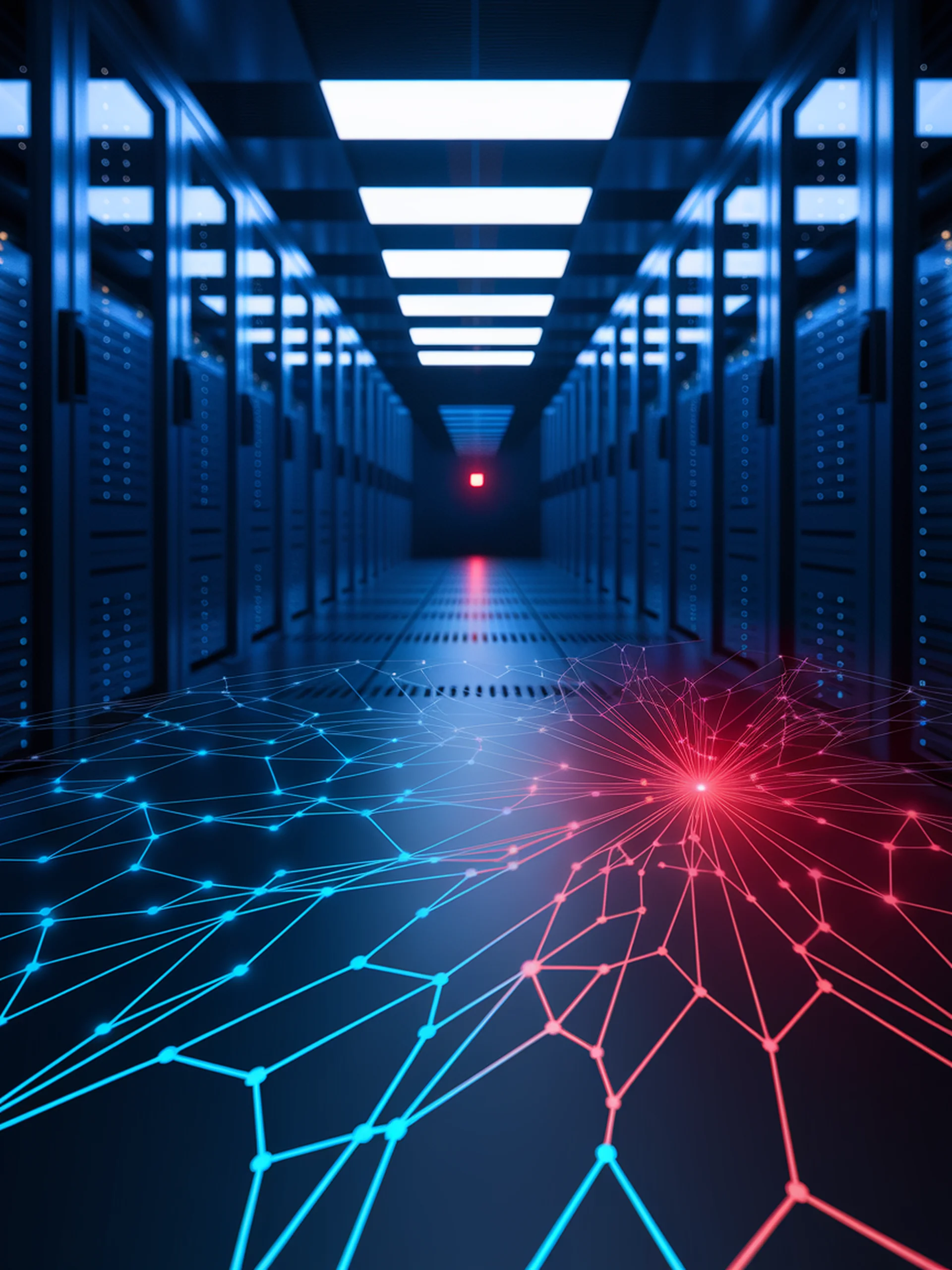
The Hidden Danger in LLM Alignment
How minimal data poisoning can compromise AI safety guardrails
This research demonstrates the alarming vulnerability of modern LLMs to data poisoning attacks during alignment training, requiring as little as 0.5% of manipulated data to bypass safety mechanisms.
- Poisoning attacks against Direct Policy Optimization (DPO) can make models generate harmful content despite safety training
- Both backdoor and non-backdoor attacks prove effective with minimal poisoned data
- Models aligned with DPO show particular susceptibility compared to other methods
- Existing defenses provide limited protection against these sophisticated attacks
For security teams, this research highlights critical vulnerabilities in current alignment techniques that could be exploited to bypass content safeguards in deployed AI systems. Organizations must implement robust data validation processes and monitoring for alignment training.
Is poisoning a real threat to LLM alignment? Maybe more so than you think