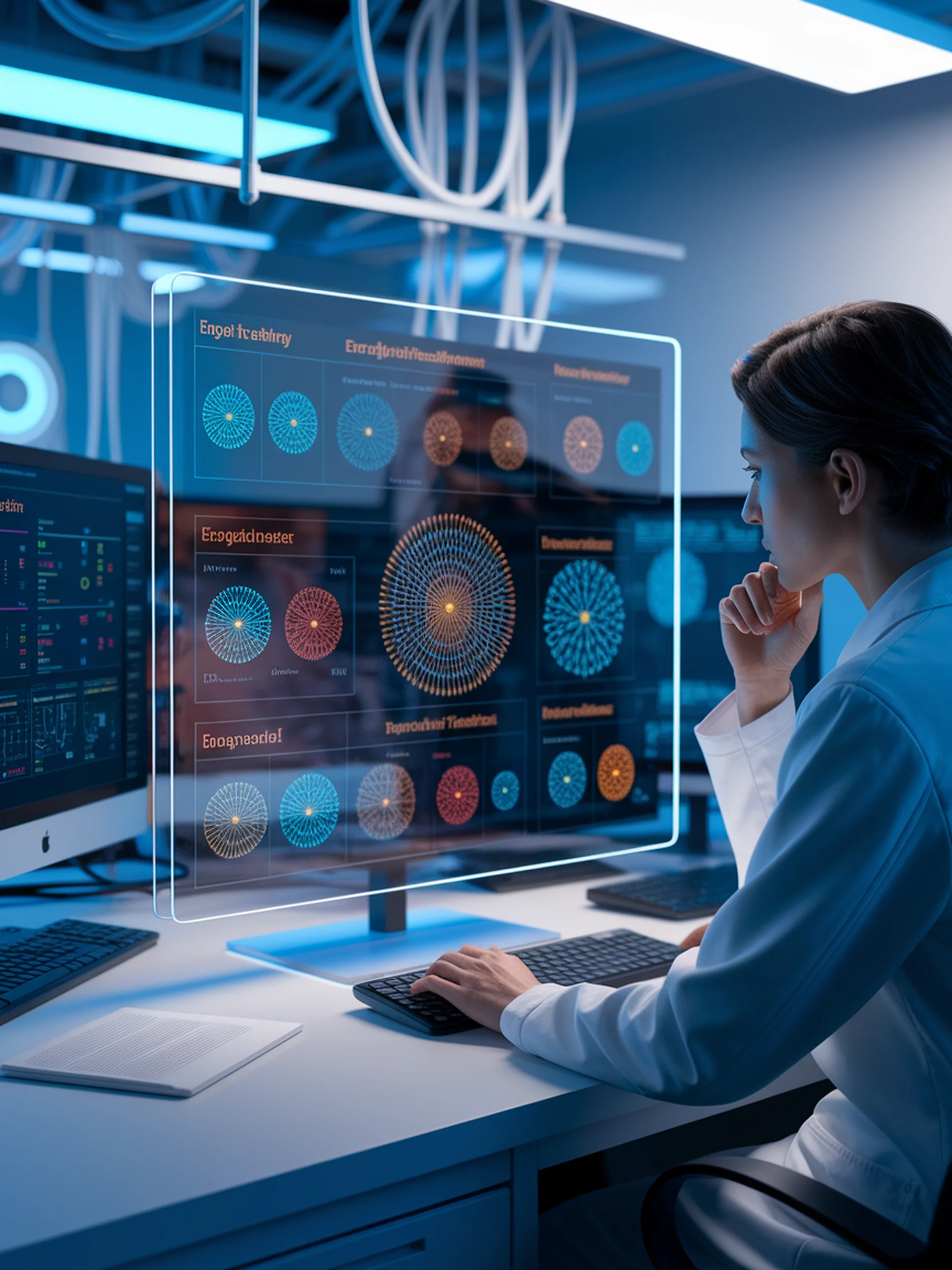
Preserving Alignment While Fine-tuning LLMs
How to maintain ethical boundaries without sacrificing performance
This research addresses the critical challenge of alignment loss that occurs when fine-tuning large language models for specific applications.
- Identifies that fine-tuning can inadvertently increase harmful response rates by up to 30%
- Proposes Multi-view Parameter Fine-tuning (MPF) that selectively updates parameters to maintain alignment
- Demonstrates how to preserve ethical guardrails while optimizing for task performance
- Shows successful application across multiple model sizes and fine-tuning scenarios
For security professionals, this research provides practical techniques to ensure AI systems remain safe and aligned with human values even after customization for specific business needs.