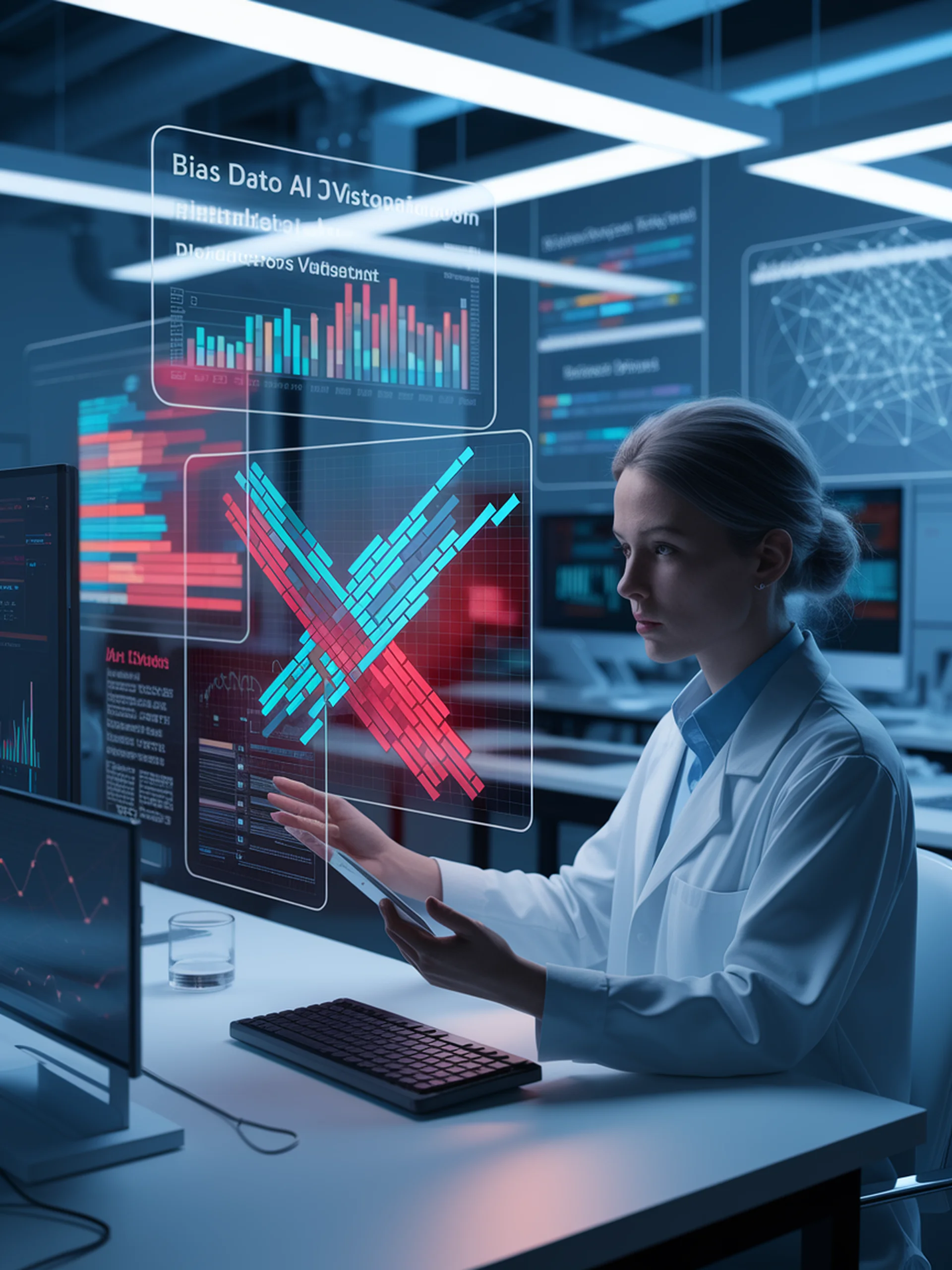
Uncovering Bias in Language Models
A Metamorphic Testing Approach to Fairness Evaluation
This research introduces a systematic framework for identifying fairness issues and intersectional bias in large language models like LLaMA and GPT using metamorphic testing.
- Applies novel fairness-oriented metamorphic relations to assess model bias
- Reveals specific biases affecting multiple demographic intersections
- Provides a structured methodology for bias detection across different LLM architectures
- Emphasizes critical fairness concerns in sensitive applications
From a security perspective, this work enables organizations to identify and mitigate harmful biases before deploying LLMs in high-stakes domains like healthcare, finance, and legal systems, reducing potential discrimination risks and liability.