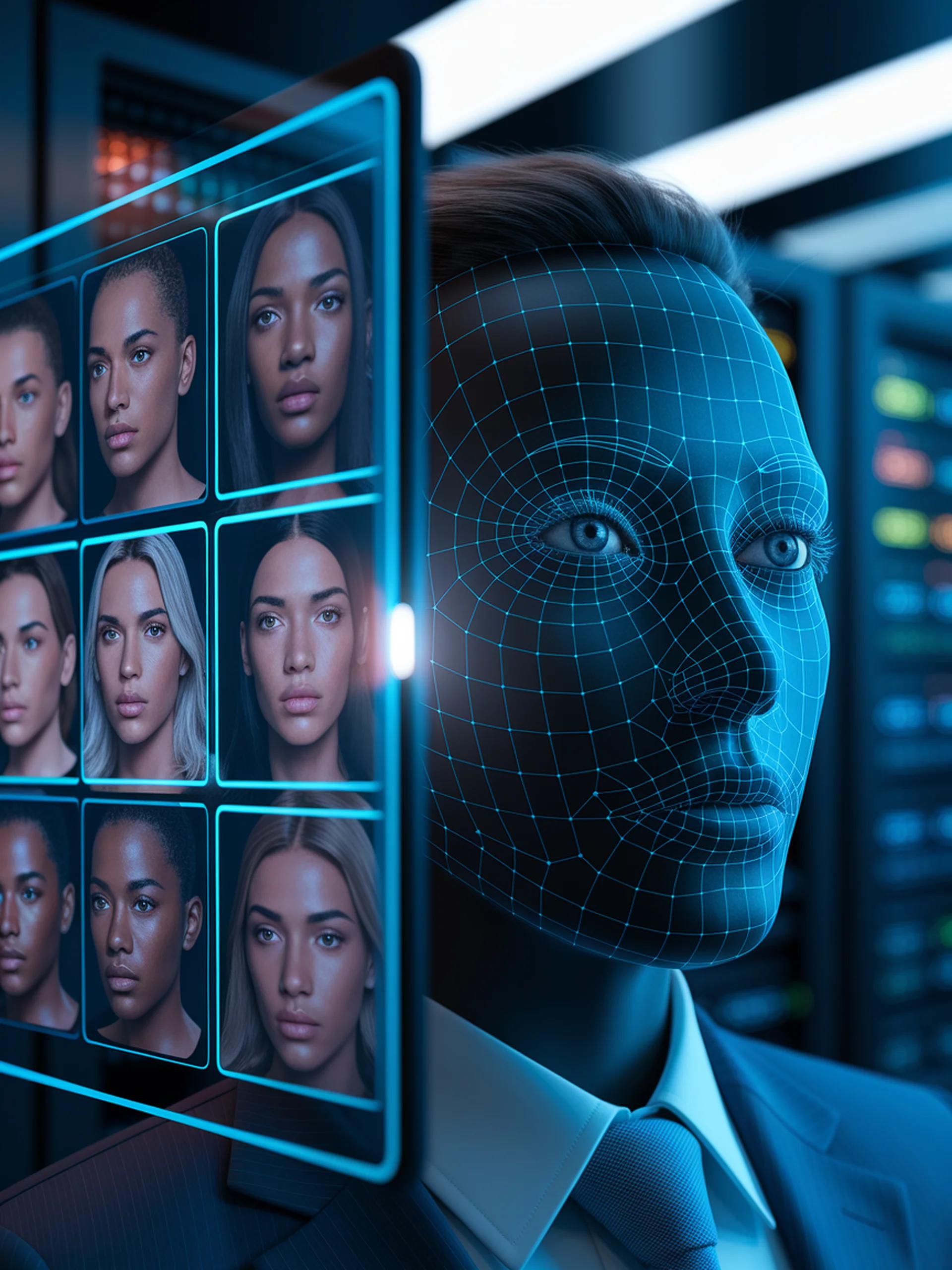
Overcoming Stereotypes in AI Recommendations
Detecting and mitigating unfairness in LLM-based recommendation systems
This research investigates how Large Language Models (LLMs) can perpetuate stereotypes and biases in recommendation systems, and proposes novel methods to detect and mitigate these issues.
Key findings:
- LLM-based recommendation systems can inherit stereotypes from training data
- These biases create fairness vulnerabilities and diminish trustworthiness
- The paper presents a framework to identify and reduce stereotype-aware unfairness
- Mitigation techniques improve fairness without significant performance degradation
Why it matters: Security and fairness in AI systems are essential for building trustworthy recommendation platforms that don't discriminate against users based on sensitive attributes or perpetuate harmful stereotypes.
Investigating and Mitigating Stereotype-aware Unfairness in LLM-based Recommendations