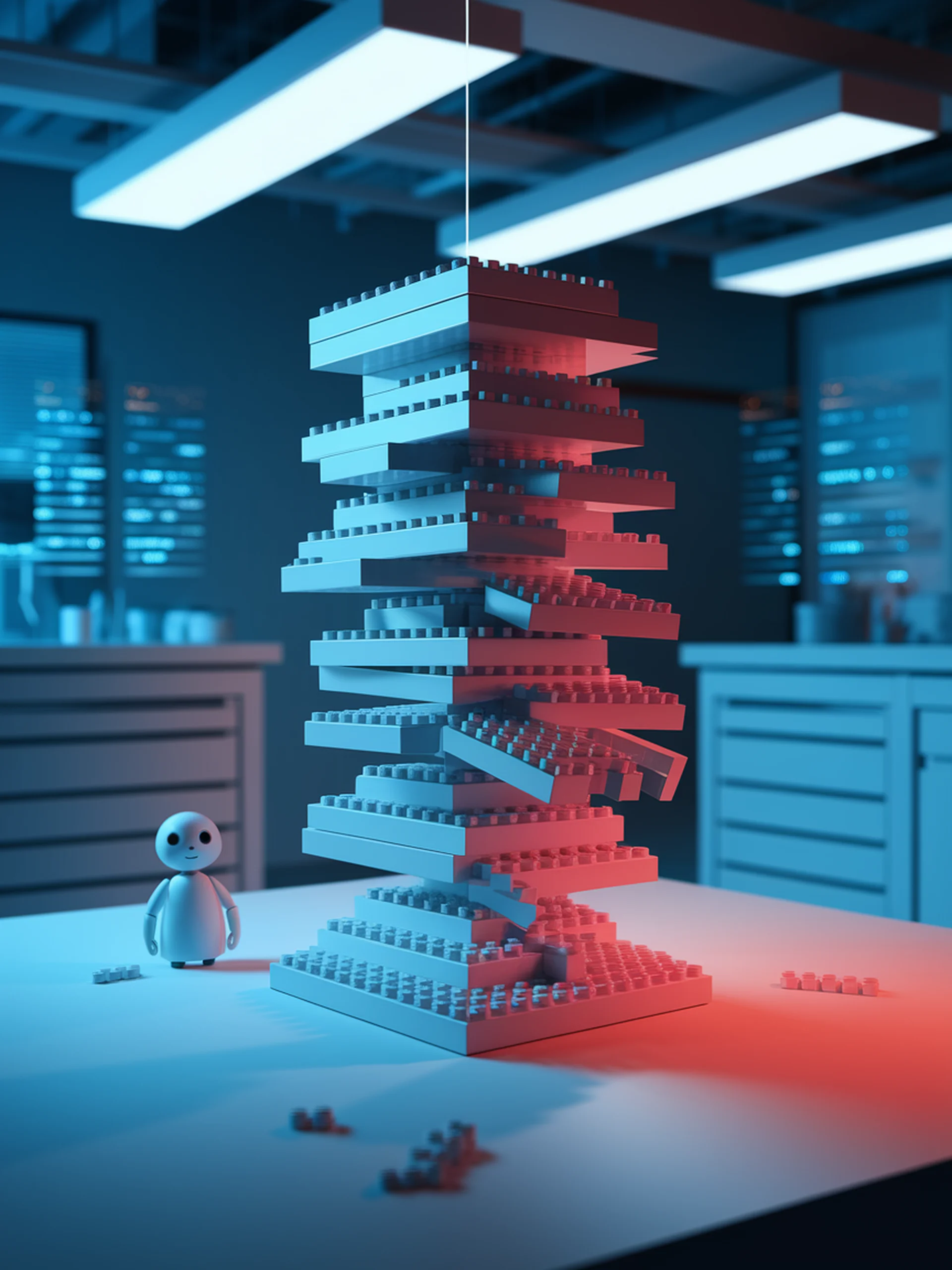
The Safety Paradox in LLM Alignment
Why multi-model synthetic preference data can undermine safety
This research reveals a critical safety-specific phenomenon in Direct Preference Optimization (DPO) alignment: using multiple models to generate preference data can actually reduce safety performance rather than improve it.
- Models trained with single-model synthetic preferences show better safety than those using multi-model approaches
- Jailbreaking attack success rates increase significantly when using multi-model preference data
- This counterintuitive finding challenges the common assumption that more diverse training data is always beneficial
- Researchers identified the safety preference reversal problem where multi-model approaches can inadvertently prioritize harmful responses
For security professionals, this research provides crucial insights for developing safer alignment strategies and highlights how seemingly better training approaches can create unexpected vulnerabilities in language models.
More is Less: The Pitfalls of Multi-Model Synthetic Preference Data in DPO Safety Alignment