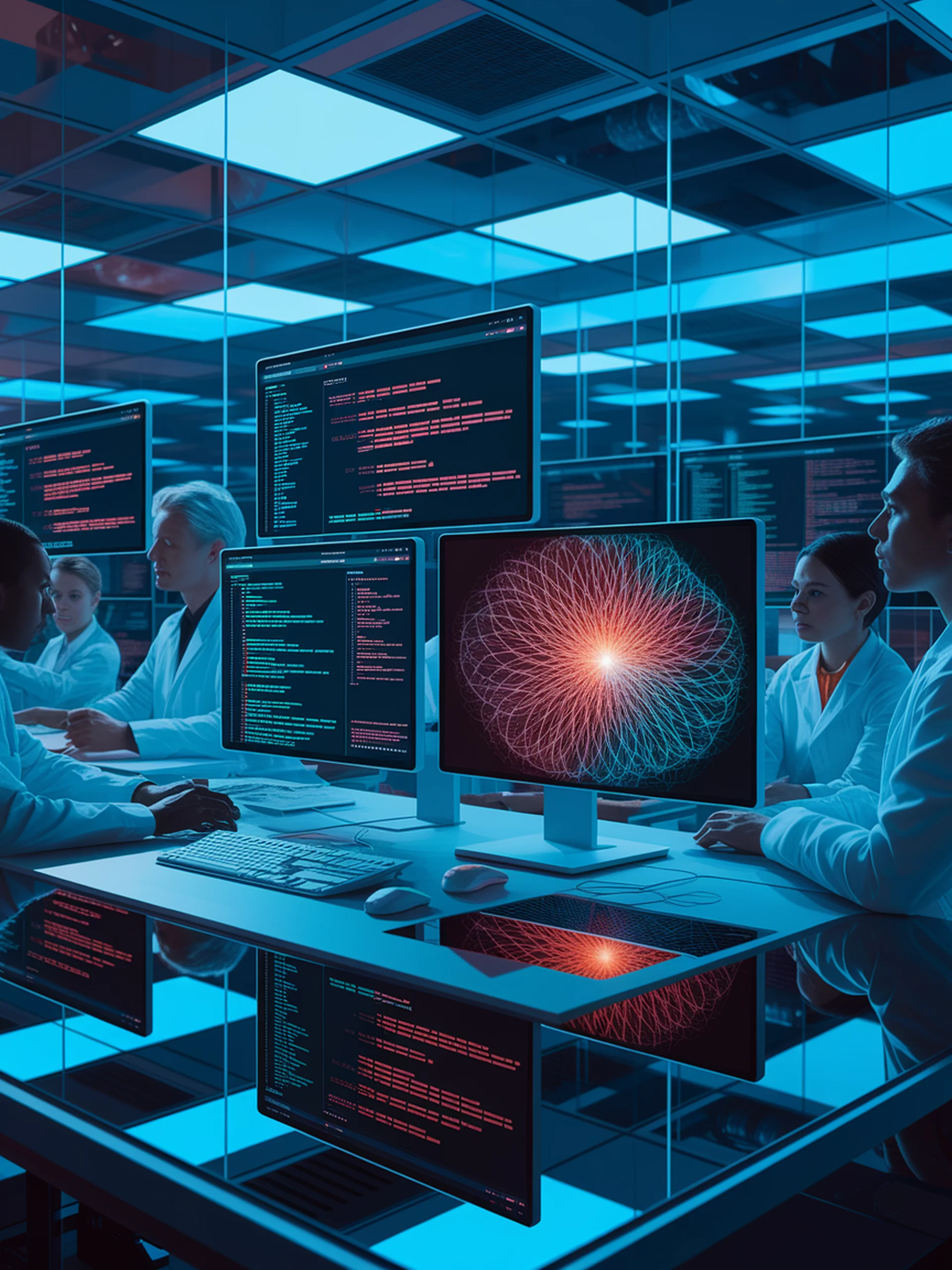
Exposing LLM Biases Through Complex Scenarios
Moving beyond simple prompts to reveal hidden value misalignments
This research introduces sophisticated evaluation techniques that expose hidden biases in LLMs by using complex dialogues and stories rather than simple adversarial prompts.
- LLMs have become adept at recognizing and avoiding single-sentence adversarial prompts
- Multi-turn dialogues and narrative contexts reveal biases that remain hidden in simpler tests
- The approach creates more realistic assessment scenarios mimicking how LLMs are used in real-world applications
- Results demonstrate that even safety-tuned models can reveal concerning ethical stances when tested with these complex scenarios
Security Implications: These findings highlight critical vulnerabilities in AI safety mechanisms, showing that current alignment techniques may create a false sense of security while problematic values remain embedded in models.
Beyond Single-Sentence Prompts: Upgrading Value Alignment Benchmarks with Dialogues and Stories