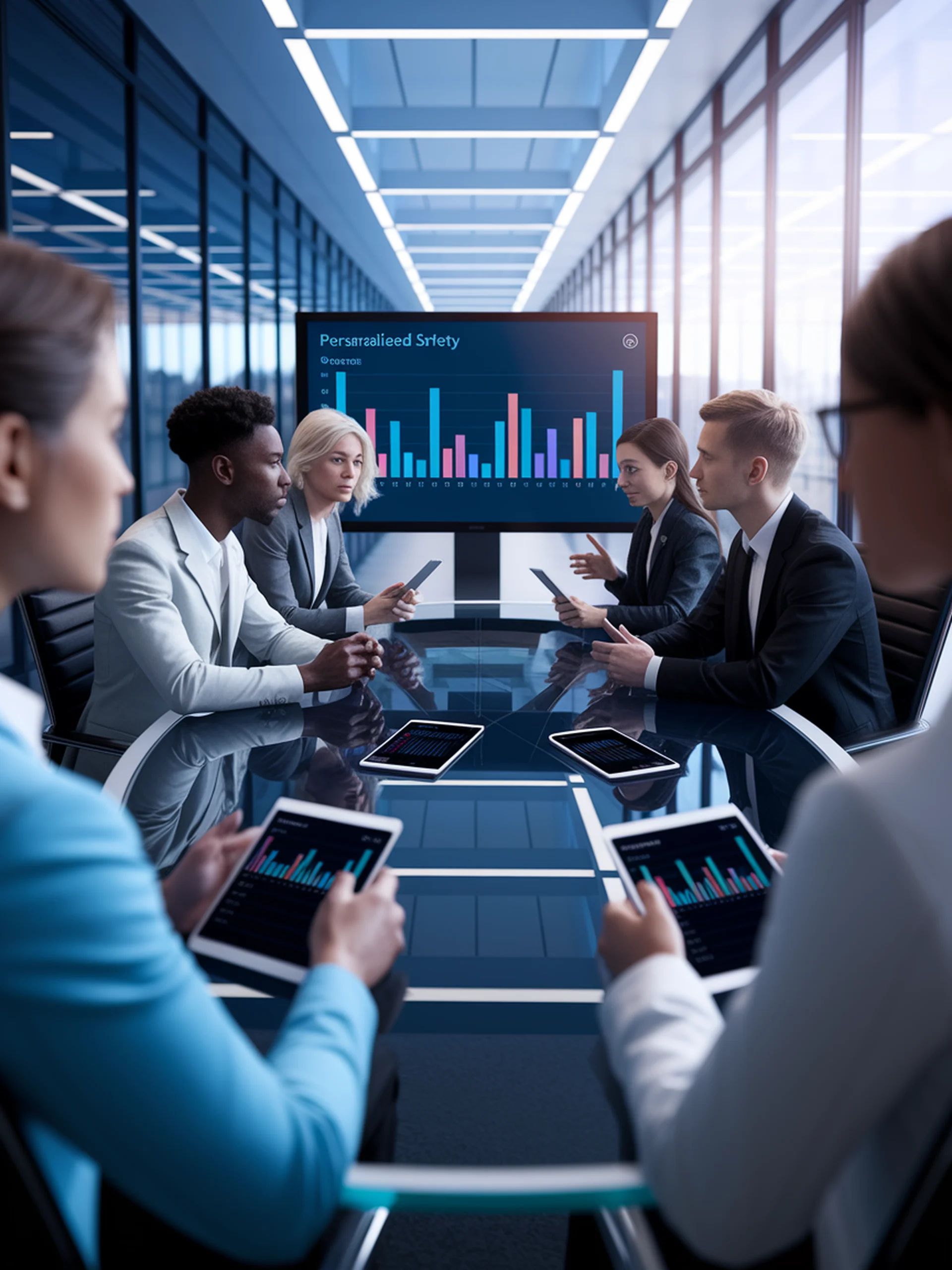
Personalized Safety in LLMs
Why one-size-fits-all safety standards fail users
This research introduces U-SAFEBENCH, a novel benchmark for evaluating how LLMs adapt safety responses to different user profiles, rather than applying universal standards.
- Evaluated 18 LLMs on their ability to provide user-appropriate safety responses
- Found significant safety gaps when dealing with different demographic profiles
- Demonstrated most current LLMs fail to adjust safety protocols for specific user needs
- Identified which models best adapt to user-specific contexts
For security professionals, this research highlights a critical vulnerability in current AI safety approaches—standardized safety measures may protect some users while failing others based on their profiles, preferences, and needs.
Is Safety Standard Same for Everyone? User-Specific Safety Evaluation of Large Language Models