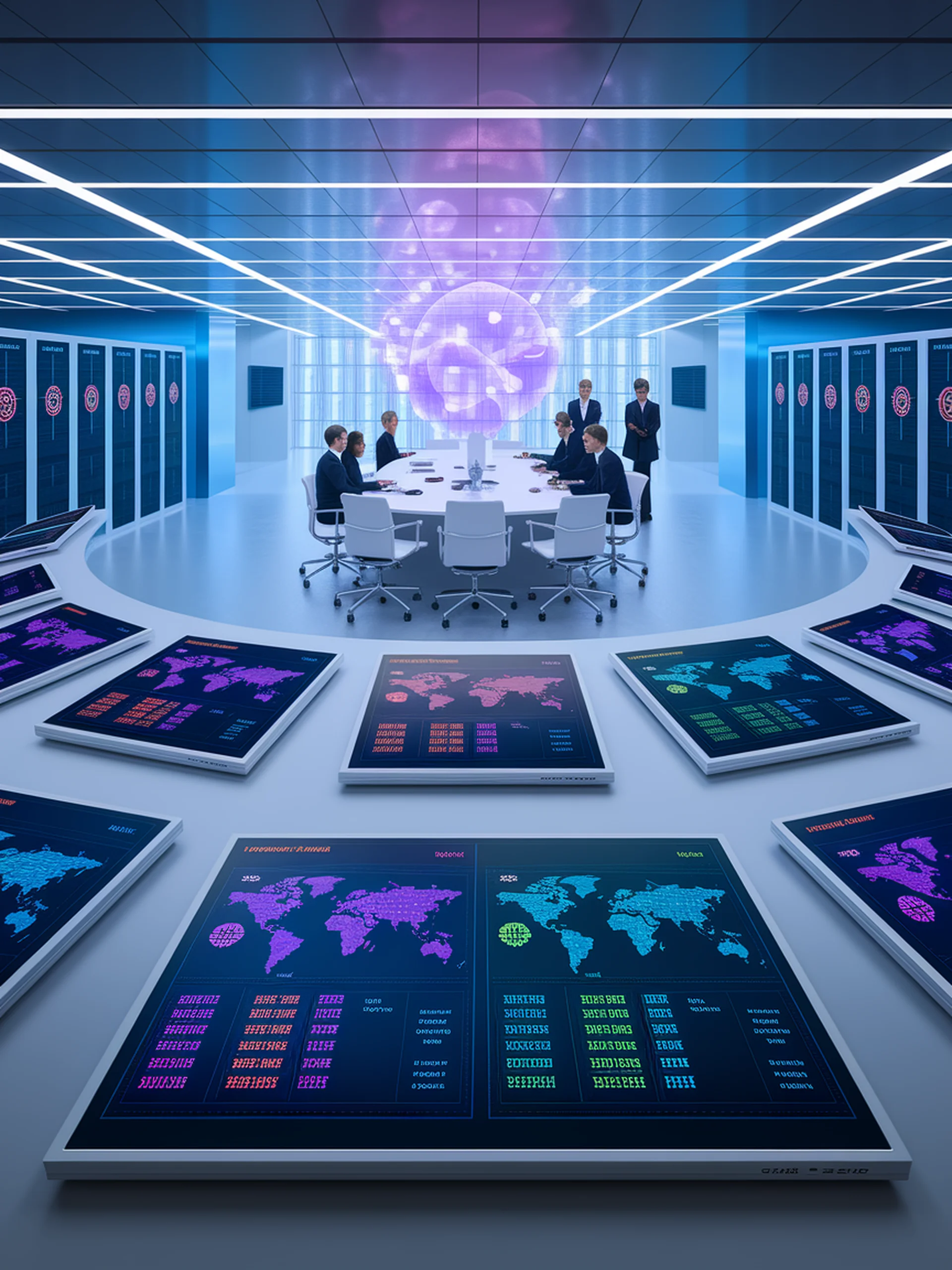
Detecting Bias in LLMs: A Framework for Safer AI
An adaptable approach for identifying harmful biases across contexts
ASCenD-BDS is a novel framework for detecting bias, discrimination, and stereotyping in Large Language Models across diverse linguistic and sociocultural contexts.
- Adaptable detection methodology that identifies harmful biases in AI systems
- Context-aware analysis that considers varying cultural and linguistic perspectives
- Stochastic approach for comprehensive bias identification
- Security-focused design to mitigate risks before AI deployment
This research directly addresses critical security concerns by providing tools to identify potentially harmful biases before they manifest in deployed AI systems, helping organizations build more trustworthy and equitable language models.