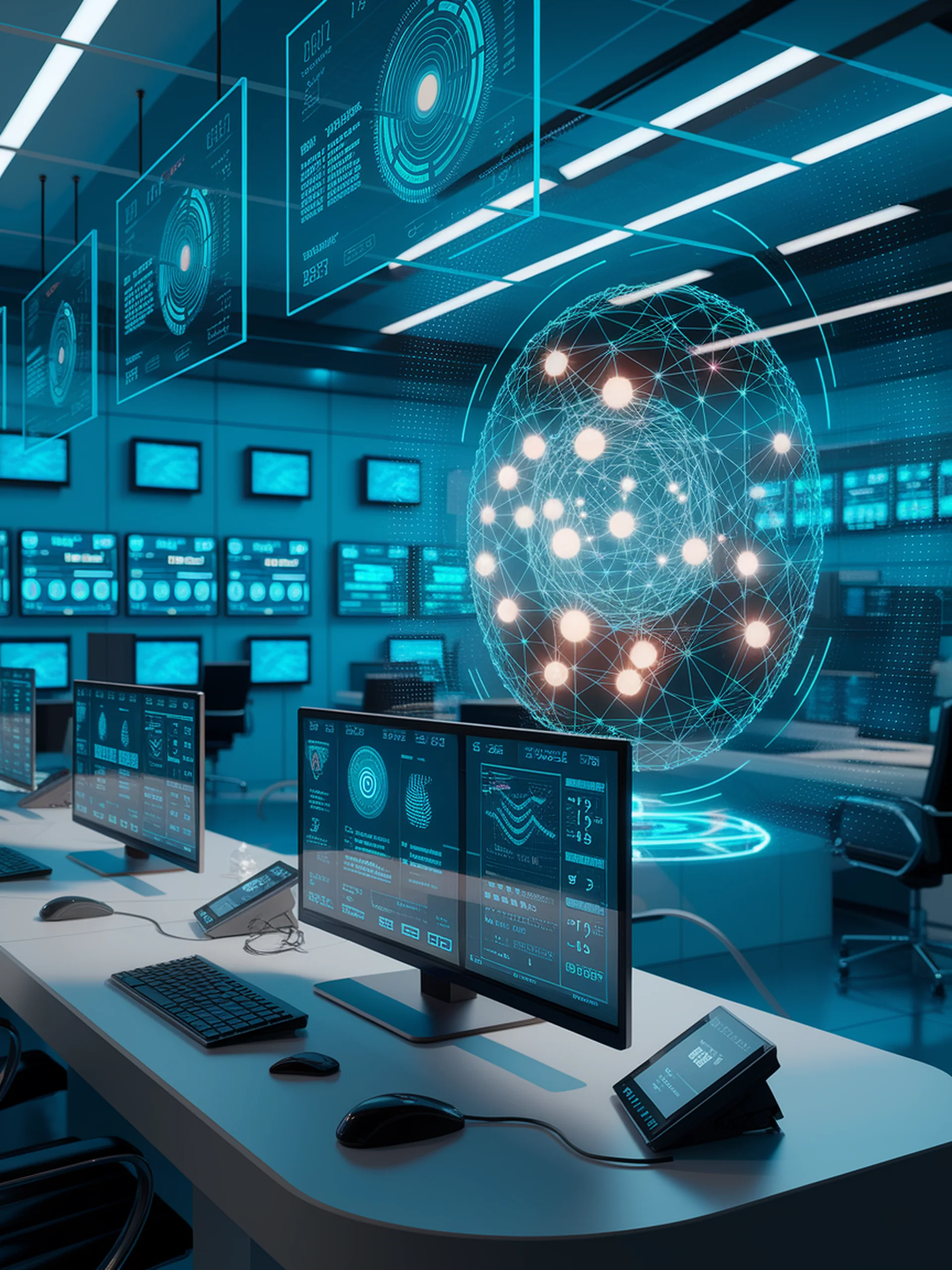
Adaptive Safety Rules for Safer AI
Enhancing LLM Security Through Dynamic Feedback Mechanisms
This research introduces data-adaptive safety rules for training reward models, significantly improving the safety performance of large language models through innovative reinforcement learning techniques.
- Develops dynamic safety evaluation that adapts to varying human preferences rather than using fixed criteria
- Achieves superior safety performance on benchmark tests compared to traditional RLHF methods
- Establishes a more nuanced feedback mechanism beyond simple paired comparisons
- Introduces fine-grained annotation approaches that better capture safety requirements
This advancement matters for security because it creates more robust guardrails against harmful outputs, enabling AI systems to better align with human safety expectations while adapting to evolving security concerns.