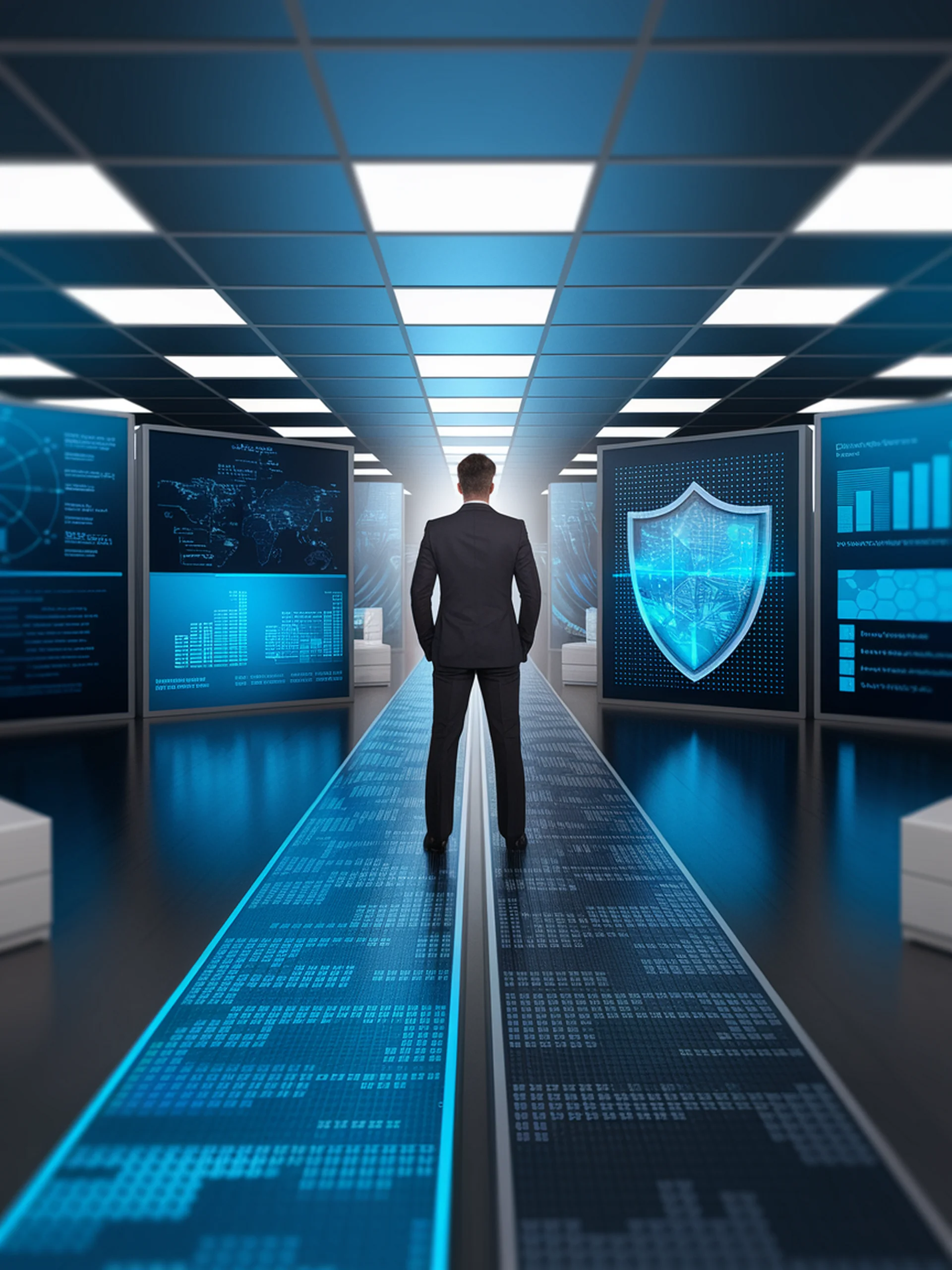
The Personalization Paradox in LLMs
Balancing Safety and Utility When Adapting to User Identity
This research reveals how personalization bias affects large language models, creating crucial safety-utility trade-offs when models adapt to user demographics.
- Models demonstrate varying levels of safety and utility when personalized to different user identities
- Performance biases emerge across different demographic groups, raising fairness concerns
- Researchers quantify these effects by evaluating both response safety to unsafe prompts and utility in knowledge tasks
- Strategic mitigation approaches are proposed to balance personalization benefits with safety risks
For security professionals, this work provides essential insights into how personalization may introduce vulnerabilities and fairness issues in LLM deployments—requiring thoughtful implementation strategies to maintain both safety and performance across diverse user populations.
Exploring Safety-Utility Trade-Offs in Personalized Language Models