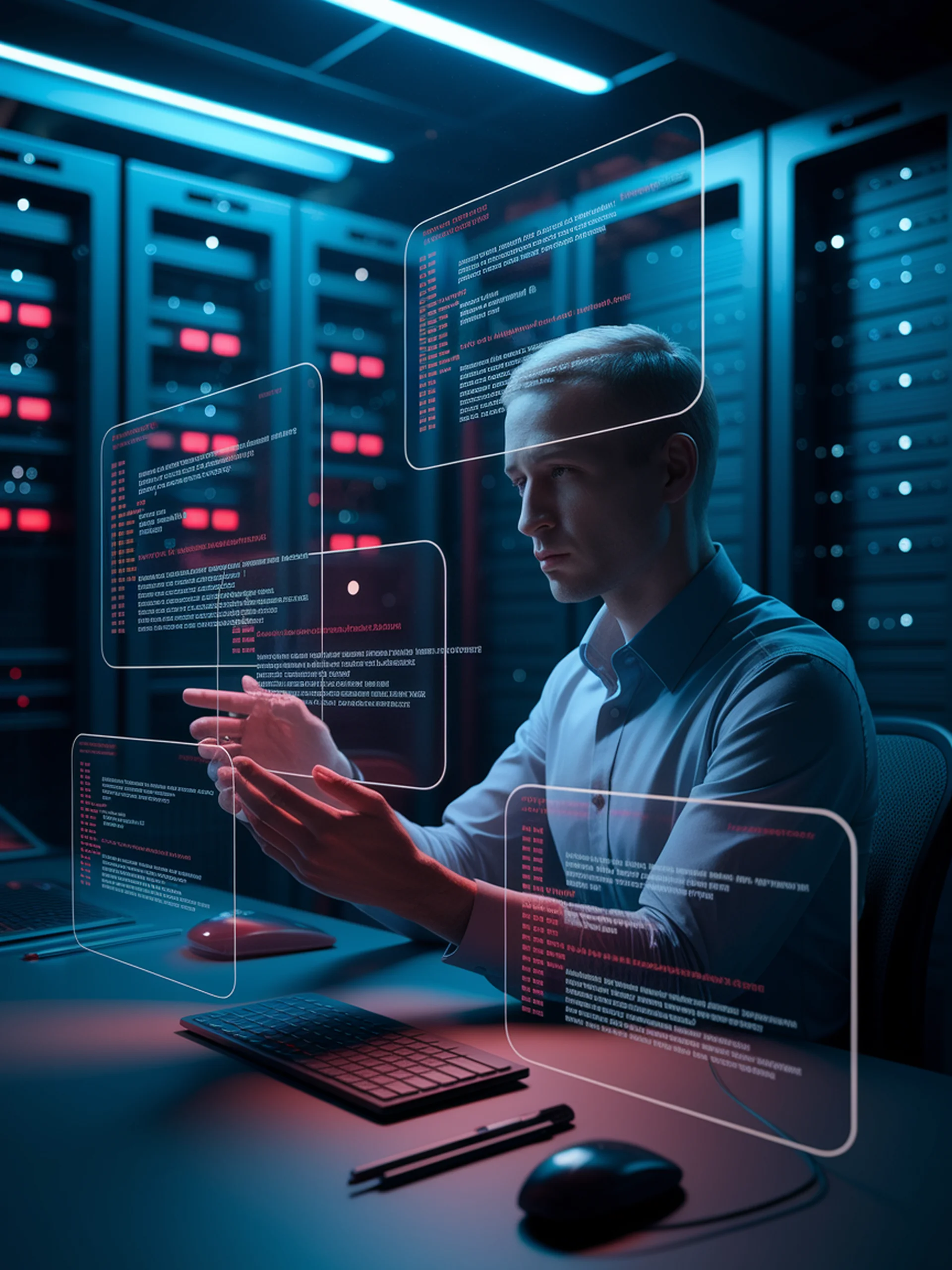
Unveiling Hidden Threats in LLMs
Detecting semantic backdoors that manipulate AI outputs
Research identifying how adversaries can implant conceptual-level triggers in LLMs that cause systematic output manipulation while evading traditional defenses.
- Semantic backdoors use meaning-based cues (ideological stances, cultural references) rather than obvious lexical patterns
- Traditional security measures miss these subtle conceptual vulnerabilities
- Proposes RAVEN detection framework to uncover hidden semantic vulnerabilities
- Critical for maintaining security and trustworthiness of AI systems in sensitive applications
Propaganda via AI? A Study on Semantic Backdoors in Large Language Models