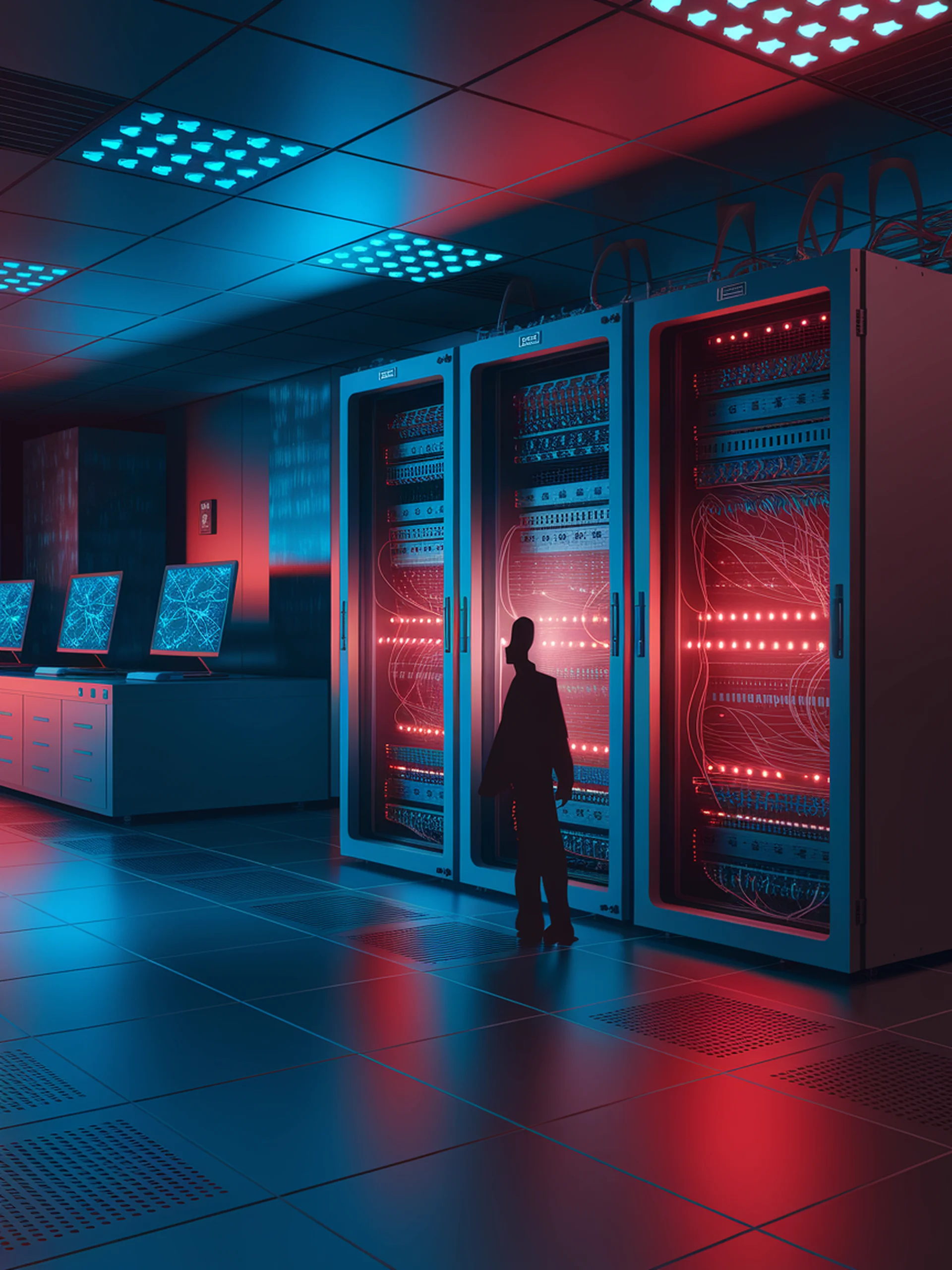
Security Vulnerabilities in RLHF Platforms
How adversaries can misalign language models through manipulation of reinforcement learning systems
This research exposes critical security flaws in Reinforcement Learning from Human Feedback (RLHF) platforms that could allow malicious actors to misalign language models.
- Demonstrates successful attacks against RLHF platforms without requiring expertise in ML algorithms
- Reveals how open-source RLHF tools can be exploited to create harmful model behaviors
- Highlights the urgent need for security assessments of these increasingly popular platforms
- Calls for safeguards to prevent malicious manipulation of alignment techniques
As RLHF becomes the standard approach for aligning LLMs with human values, understanding these vulnerabilities is essential for building trustworthy AI systems that resist adversarial manipulation.