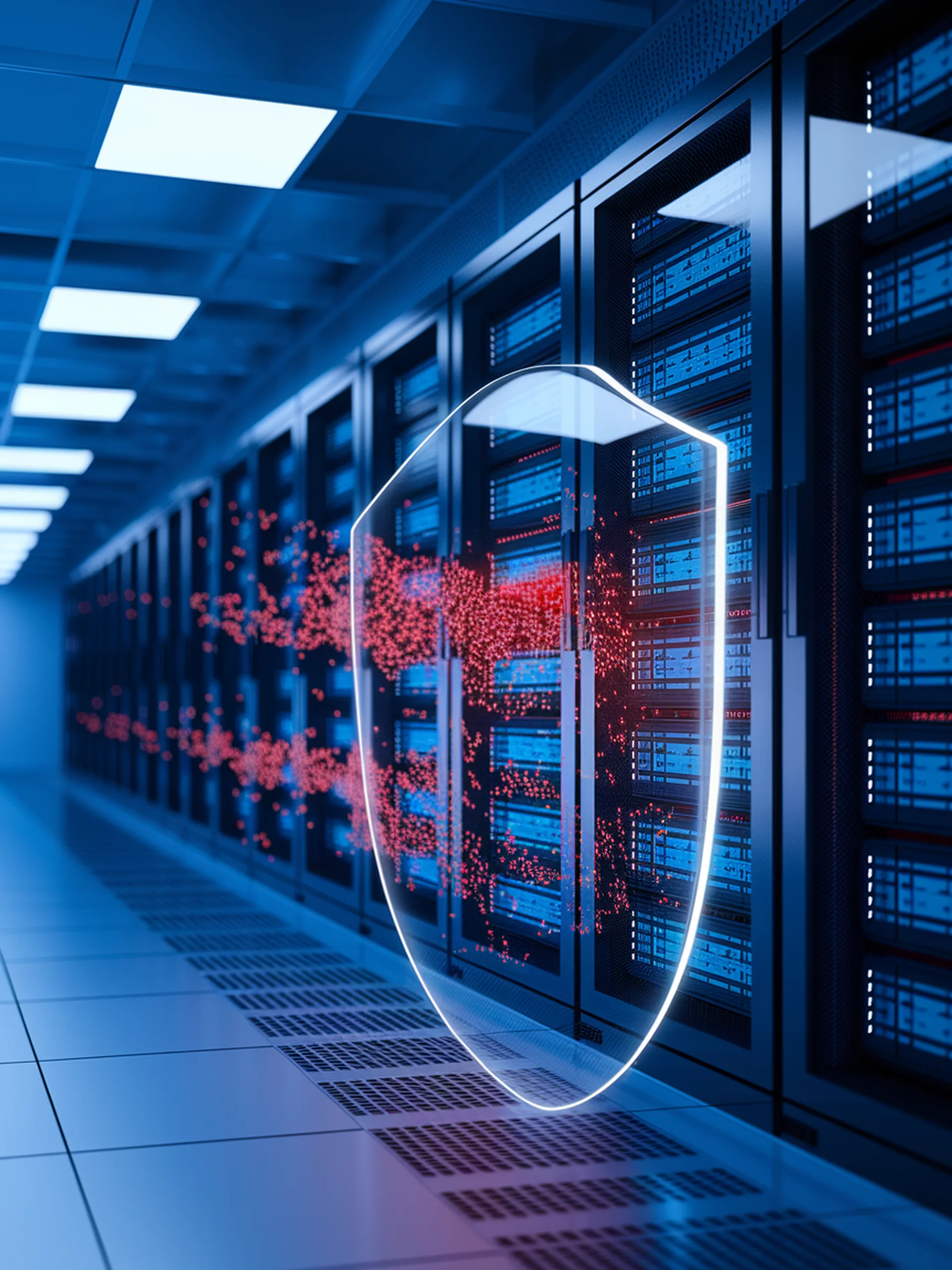
Defending AI Models from Poisoned Training Data
A novel adversarial training approach to counter label poisoning attacks
As AI systems increasingly rely on public data sources, FLORAL emerges as a powerful defense mechanism against adversaries who manipulate training labels to compromise model integrity.
- Introduces a support vector-based adversarial training strategy specifically designed to protect against label poisoning
- Addresses a critical security vulnerability in large language models that use human-annotated labels
- Offers a practical solution for maintaining model performance even when training data has been tampered with
- Particularly valuable for high-stakes AI deployments where model reliability is essential
This research provides security teams with a concrete method to fortify AI systems against an emerging threat vector, ensuring models remain trustworthy even when training on potentially compromised datasets.
Adversarial Training for Defense Against Label Poisoning Attacks