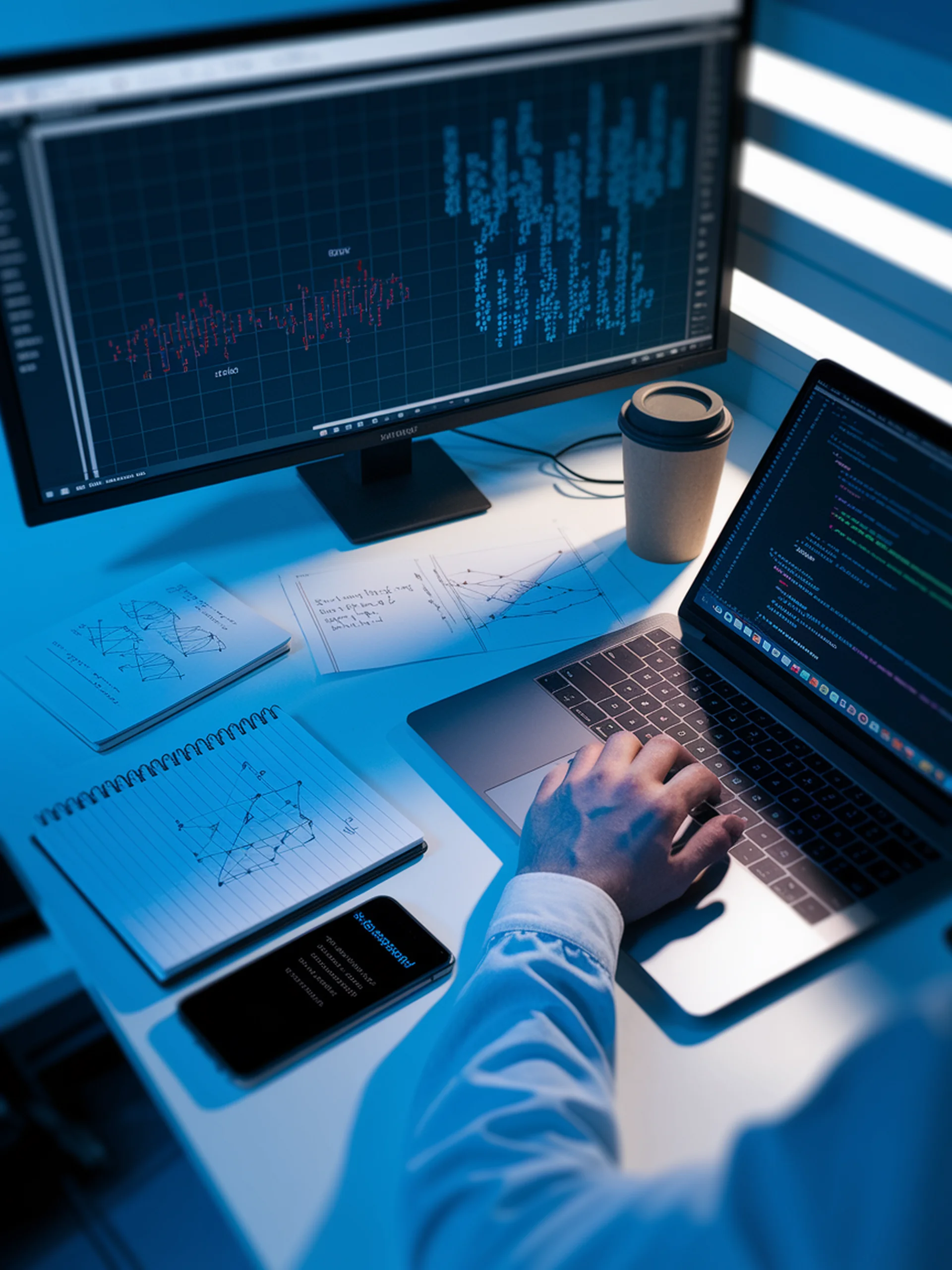
Synthetic Data for Better AI Security
Using LLMs to Generate OOD Data for Robust Classification
This research presents an innovative approach to out-of-distribution (OOD) detection by leveraging LLMs to generate synthetic OOD data, eliminating the need for external data sources.
- Creates high-quality OOD proxies using generative capabilities of Large Language Models
- Addresses the critical challenge of OOD data scarcity in classification systems
- Enables more reliable detection of misaligned and toxic AI outputs
- Enhances security of AI systems by improving their ability to recognize when inputs fall outside their training parameters
This advancement is crucial for security as it helps prevent AI systems from making dangerous predictions or generating harmful content when faced with unexpected inputs, significantly improving deployment reliability in real-world applications.
Out-of-Distribution Detection using Synthetic Data Generation