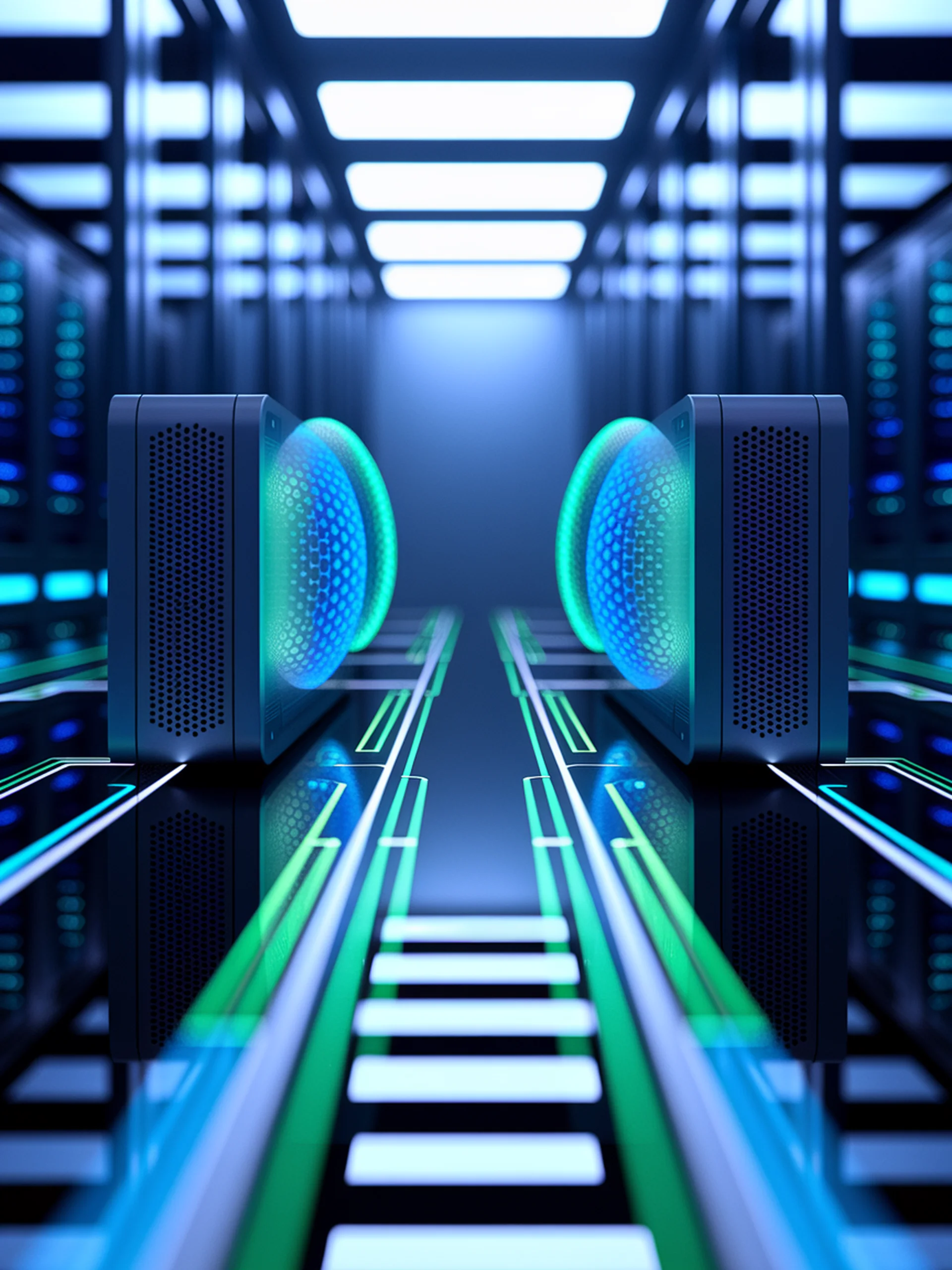
Secure AI Collaboration at the Edge
Building Resilient Multi-Task Language Models Against Adversarial Threats
This research introduces a novel approach for secure collaborative AI development at the wireless edge, enabling users to safely combine specialized language models into resilient multi-task systems.
- Addresses the challenge of efficiently creating multi-task LLMs without exhaustive retraining
- Proposes R-MTLLMF (Resilient Multi-Task Large Language Model Fusion), a method to protect against adversarial attacks
- Enables edge devices to safely share and combine model parameters without security compromises
- Focuses specifically on maintaining model integrity under worst-case adversarial noise scenarios
Why it matters: As AI deployment shifts to edge devices, this research provides critical security foundations for collaborative AI development without centralized infrastructure, protecting sensitive applications from malicious interference.
R-MTLLMF: Resilient Multi-Task Large Language Model Fusion at the Wireless Edge