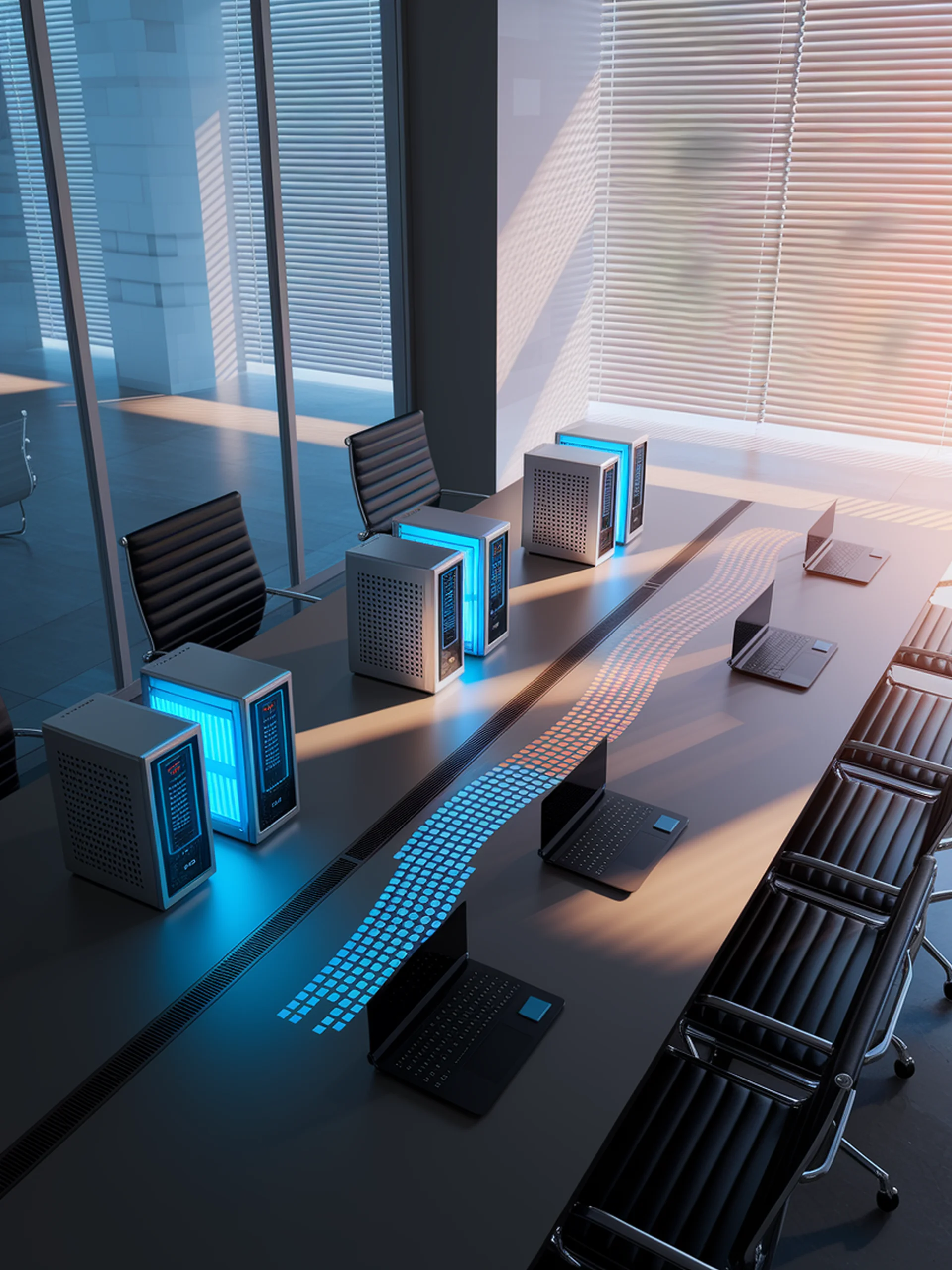
Hidden Costs of Faster AI
How acceleration techniques affect bias in LLMs
This research reveals that inference acceleration techniques (quantization, pruning, caching) can significantly amplify demographic biases in large language models.
- Acceleration methods that reduce computational costs can increase biased outputs
- Different acceleration techniques exhibit varying impacts on different types of bias
- Trade-off exists between inference efficiency and fairness/equity
- Security implications extend beyond performance to include social fairness concerns
For security professionals, this research highlights critical considerations when deploying accelerated LLMs in production environments, where biased outputs may create legal and ethical vulnerabilities.