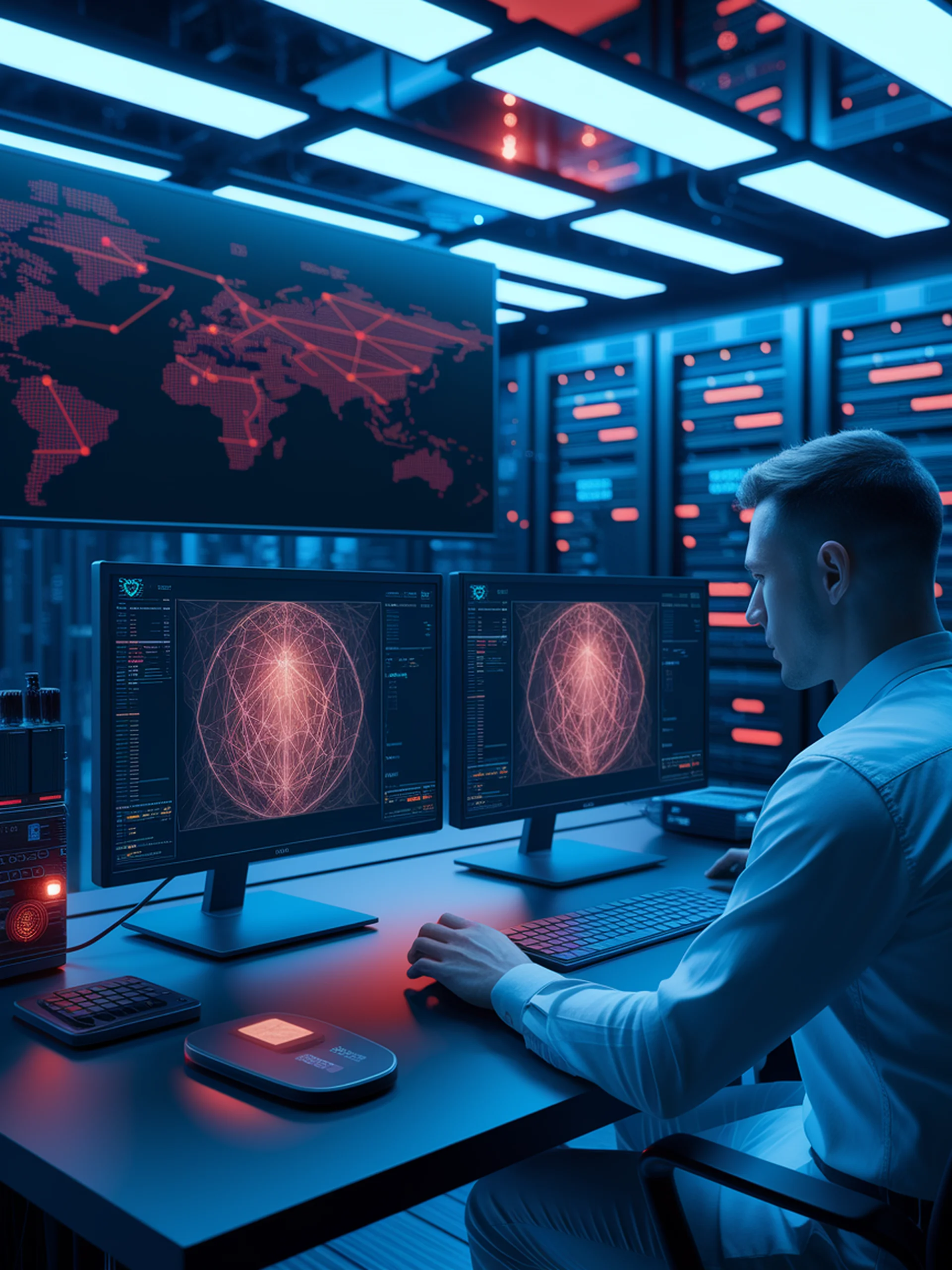
Exposing VLM Vulnerabilities
Self-supervised adversarial attacks on vision-language models
AnyAttack introduces a framework that can generate adversarial examples against vision-language models without requiring specific targets or labels, making real-world attacks more feasible.
- Creates universal adversarial perturbations that can affect multiple images and models
- Uses a self-supervised approach that doesn't need predefined attack targets
- Demonstrates critical security vulnerabilities in widely-used VLMs
- Requires immediate attention from security researchers and VLM developers
This research highlights significant security risks in multimodal AI systems currently being deployed across industries. As VLMs become more prevalent in critical applications, understanding these attack vectors becomes essential for building robust AI security protocols.
AnyAttack: Towards Large-scale Self-supervised Adversarial Attacks on Vision-language Models