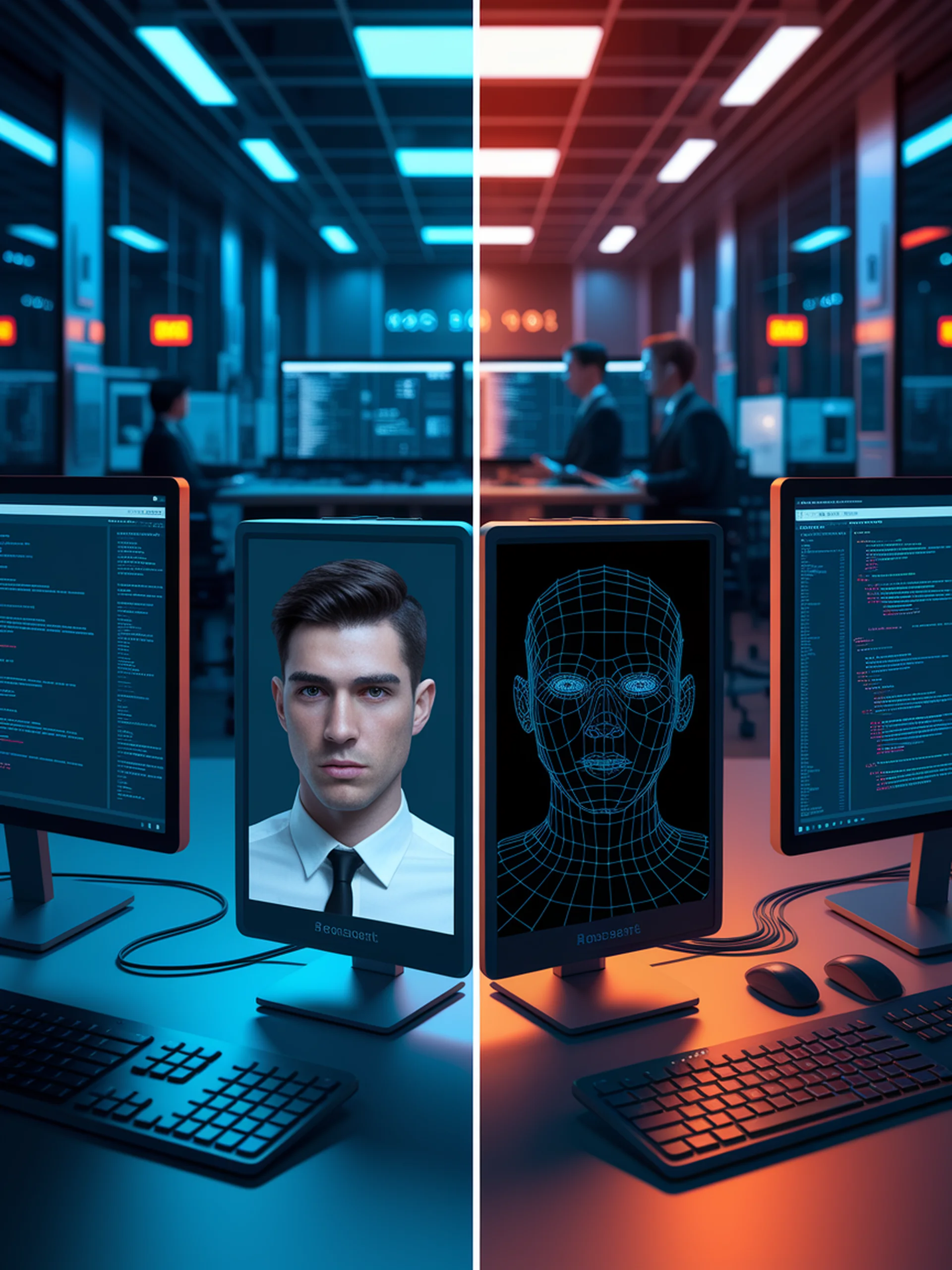
Backdoor Threats to Vision-Language Models
Identifying security risks with out-of-distribution data
This research introduces VLOOD, a novel approach to backdoor Vision-Language Models (VLMs) using only out-of-distribution data, representing a more realistic attack scenario.
- Demonstrates how attackers can compromise VLMs without access to original training data
- Reveals vulnerabilities in multimodal AI systems that combine vision and language capabilities
- Proposes practical attack vectors using readily available external data
- Highlights significant security implications for AI deployment in critical applications
This work matters because it exposes previously unexplored security risks in increasingly popular multimodal AI systems, showing how they can be manipulated to produce harmful outputs when processing seemingly innocent images.
Backdooring Vision-Language Models with Out-Of-Distribution Data